Pixel-Wise Contrastive Distillation
arxiv(2022)
摘要
We present the first pixel-level self-supervised distillation framework specified for dense prediction tasks. Our approach, called Pixel-Wise Contrastive Distillation (PCD), distills knowledge by attracting the corresponding pixels from student's and teacher's output feature maps. This pixel-to-pixel distillation demands for maintaining the spatial information of teacher's output. We propose a SpatialAdaptor that adapts the well-trained projection/prediction head of the teacher used to encode vectorized features to processing 2D feature maps. SpatialAdaptor enables more informative pixel-level distillation, yielding a better student for dense prediction tasks. Besides, in light of the inadequate effective receptive fields of small models, we utilize a plug-in multi-head self-attention module to explicitly relate the pixels of student's feature maps. Overall, our PCD outperforms previous self-supervised distillation methods on various dense prediction tasks. A backbone of ResNet-18 distilled by PCD achieves $37.4$ AP$^\text{bbox}$ and $34.0$ AP$^{mask}$ with Mask R-CNN detector on COCO dataset, emerging as the first pre-training method surpassing the supervised pre-trained counterpart.
更多查看译文
关键词
contrastive distillation,pixel-wise
AI 理解论文
溯源树
样例
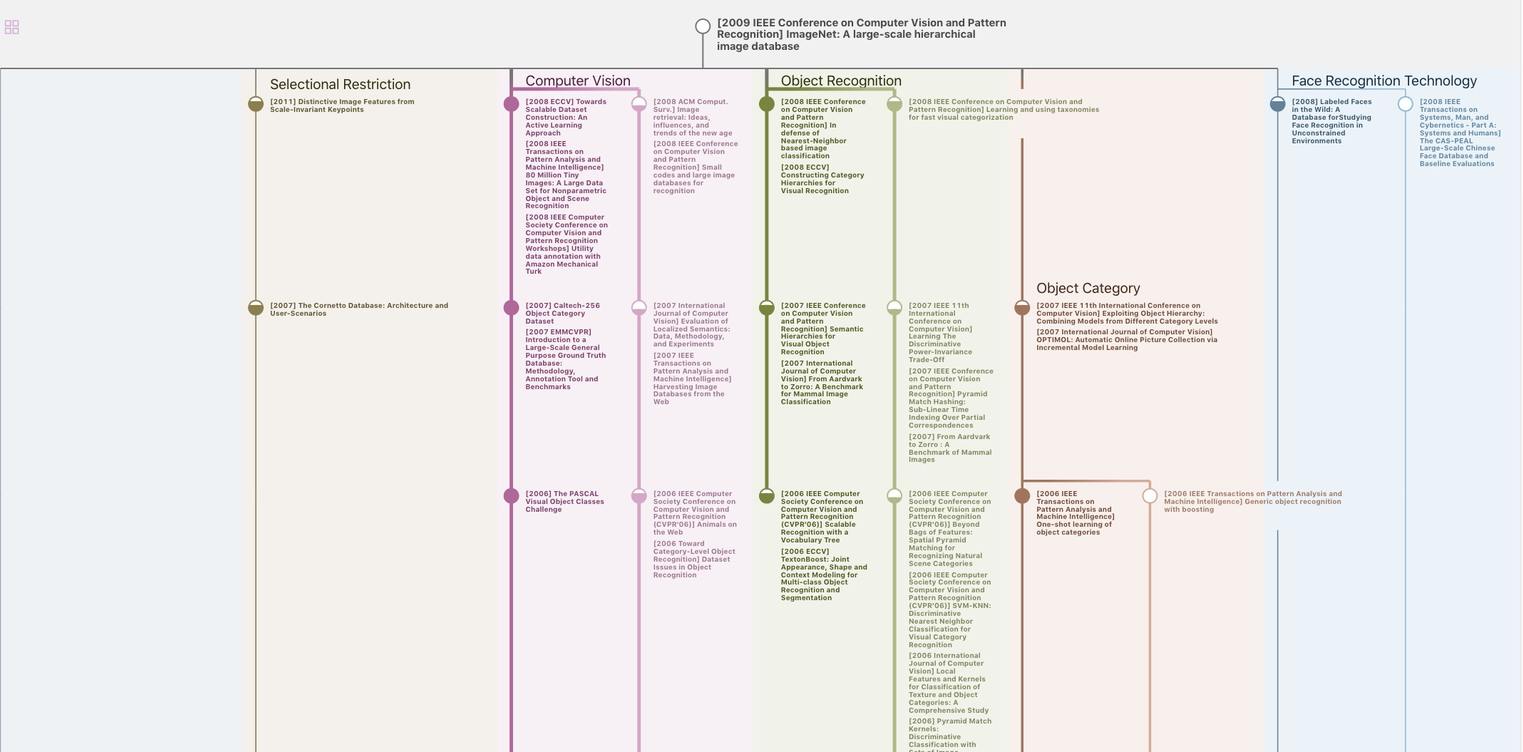
生成溯源树,研究论文发展脉络
Chat Paper
正在生成论文摘要