tinyCare: A tinyML-based Low-Cost Continuous Blood Pressure Estimation on the Extreme Edge
2022 IEEE 10th International Conference on Healthcare Informatics (ICHI)(2022)
摘要
We propose a solution that deploys Machine Learning (ML) techniques on resource-constrained edge devices (tinyMl)for the healthcare domain. In particular, we construct a complete end-to-end prototyped system that conducts ML inference with various ML techniques on microcontroller unit (MCU)-powered edge devices to predict blood-pressure-related vital metrics such as systolic (SBP), diastolic (DBP), and mean arterial (MAP) blood pressures using electrocardiogram (ECG) and photoplethysmogram (PPG) sensors. The proposed solution is trained and tested using over 500 hours of 12, 000 real intensive care unit data instances. Despite running on an extremely limited computation, power and memory budget, the proposed solution achieves comparable results to server-based state-of-the-art solutions. Furthermore, it meets the British Hypertension Society (BHS) standard for grade B (C in extremely-constrained devices). This is achieved by careful investigation of the correlation between a wide-set of ECG and PPG features and BP. Afterwards, we compress the ML inference models by only incorporating the minimal features that meet i) the edge constraints from one side, and ii) the standard's acceptable accuracy from the other side. Unlike existing solutions, the inference is entirely conducted on MCU-based edge devices without depending on any cloud-based infrastructure. Hence, the proposed solution improves robustness, accessibility, reliability, security, as well as data privacy.
更多查看译文
关键词
blood pressure,machine learning,tinyML,edge,monitoring,regression,BP,DBP,SBP,ECG,PPG
AI 理解论文
溯源树
样例
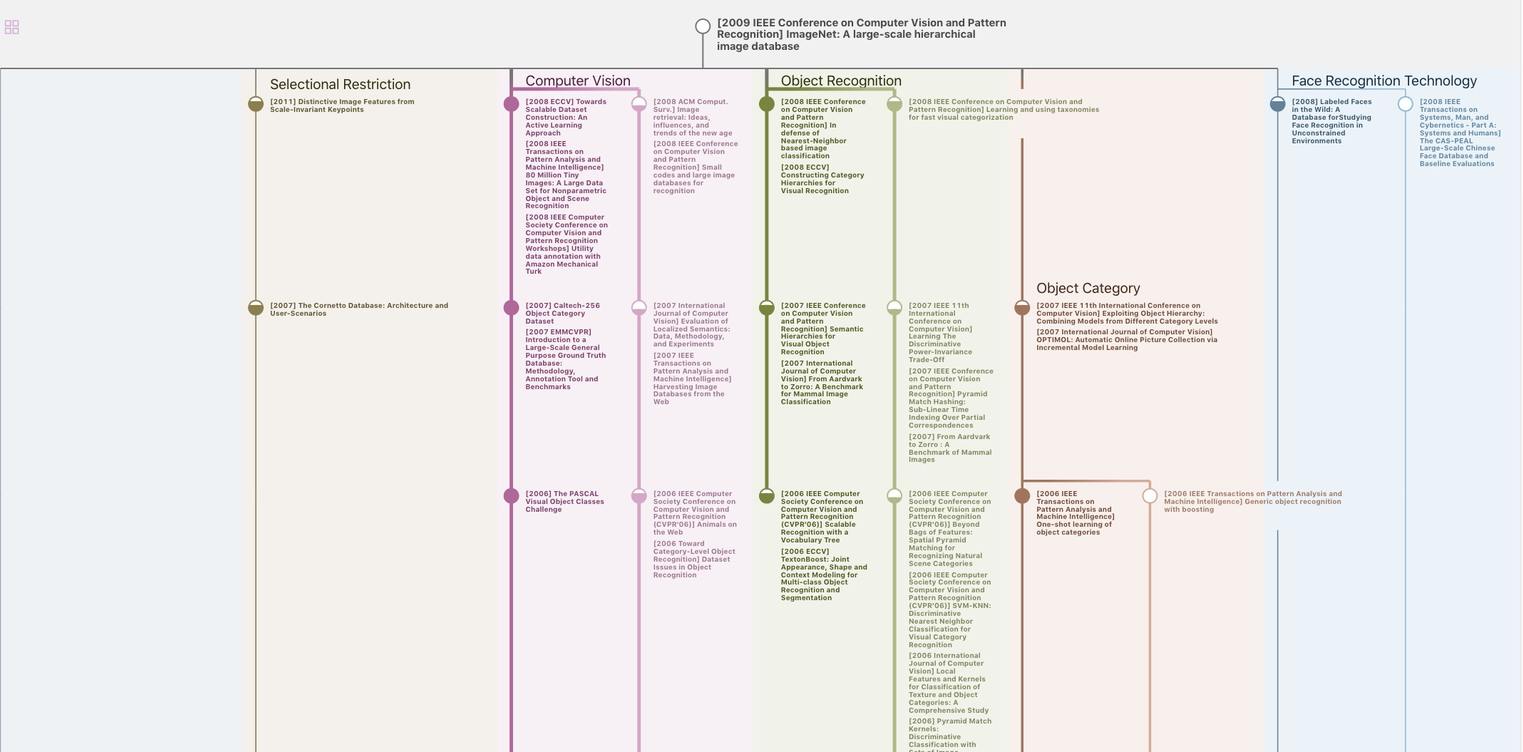
生成溯源树,研究论文发展脉络
Chat Paper
正在生成论文摘要