Combining Attention-based Models with the MeSH Ontology for Semantic Textual Similarity in Clinical Notes
2022 IEEE 10th International Conference on Healthcare Informatics (ICHI)(2022)
摘要
In this study, we present several transformer-based models as well as traditional machine learning methods to detect semantic textual similarity (STS) in clinical notes. We investigate transformer models pretrained on general English as well as clinical notes, and use generic English STS datasets as a supplemental corpus to clinical notes data. Our work is based on the 2019 National NLP Clinical Challenge (n2c2). We identify and annotate six types of sentences in the clinical notes corpus, and report an ensemble method that combines attention-based contextualized embeddings with a similarity score based on the MeSH ontology obtained by computing least common ancestors of clinical terms. Our approach does not need additional clinical data for model training, while still achieving comparable Pearson's correlation coefficient of 0.901.
更多查看译文
关键词
Electronic Health Records,Natural Language Processing,Clinical Semantic Textual Similarity,Transformers,MeSH
AI 理解论文
溯源树
样例
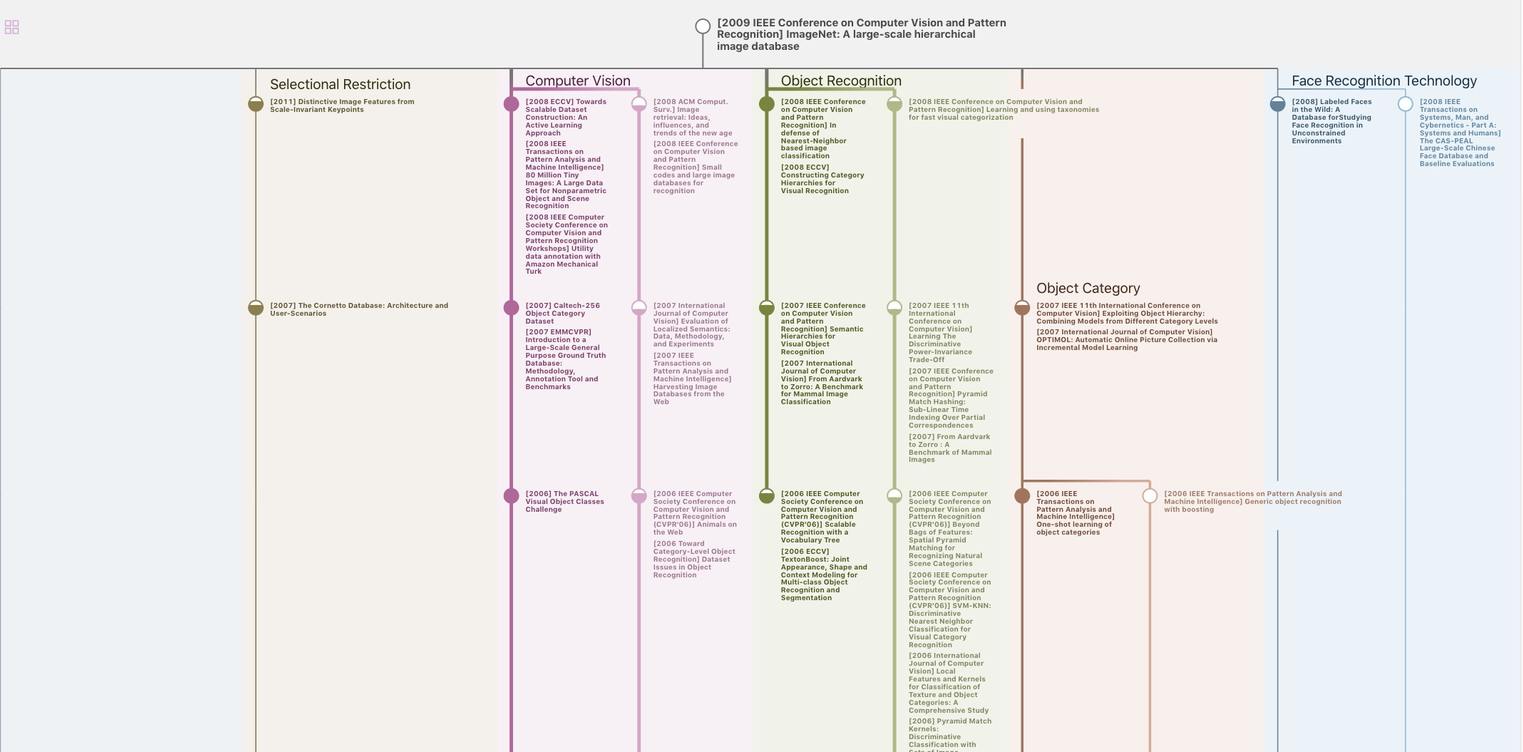
生成溯源树,研究论文发展脉络
Chat Paper
正在生成论文摘要