Comparative Evaluation of Gradient Boosting with Active Thresholding and Model Explainability for Peak Demand Forecasting
2022 15th International Conference on Human System Interaction (HSI)(2022)
摘要
The rapid advancement of the energy sector in terms of diverse energy generation options and increasing energy consumption loads has eventuated the need for highly accurate demand forecasting methods. The prevalence of large volumes of energy data streams and sophisticated Artificial Intelligence (AI) algorithms has enabled a rapid transition to AI-based forecasting methods that are more accurate and computationally efficient. Despite this transition, demand forecasting during peak events and peak temporal periods continues to be a challenge due to the irregularity and transience of such events. Besides the challenge of managing supply and demand, the financial viability of forecasting is also questioned when the forecast decreases in accuracy during peak periods when the energy price is an increasing function. In this paper, we have set out to address the challenge of peak demand forecasting by specifically transforming both input vectors and input attributes of the smart meter data streams. Input vectors are transformed using active thresholding while input attributes are transformed into a feature subset using model explainability. We have evaluated the effectiveness of this data transformation on the current state-of-the-art AI for energy demand forecasting, gradient boosting. We conduct a comparative evaluation using two real-world energy consumption datasets drawn from the La Trobe Energy AI/Analytics Platform (LEAP), of La Trobe University’s Net Zero Carbon Emissions Program. The proposed approach surpasses the baseline approach in both datasets, with an improvement of 27% for the second dataset which is a high energy consumption setting.
更多查看译文
关键词
Peak Forecast,Demand forecasting
AI 理解论文
溯源树
样例
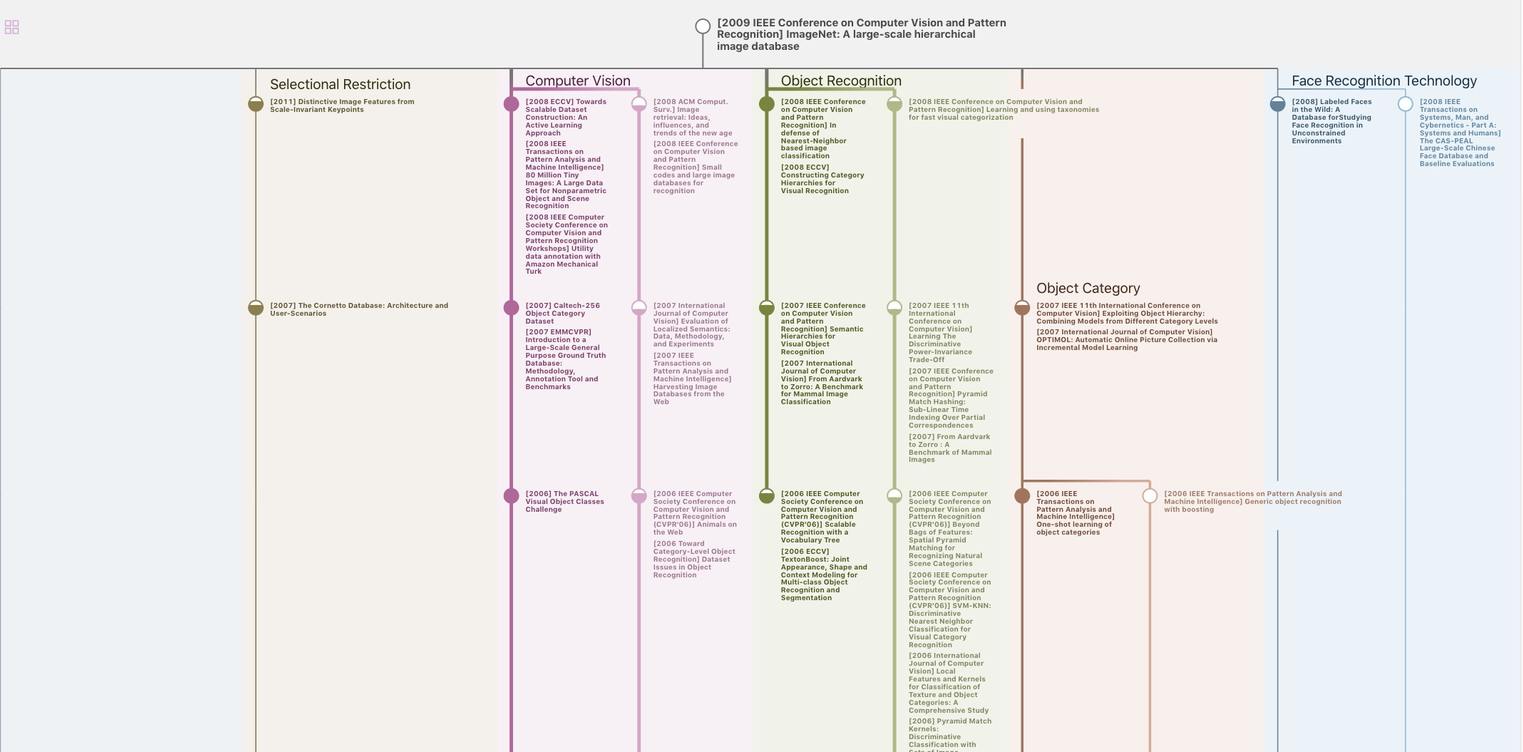
生成溯源树,研究论文发展脉络
Chat Paper
正在生成论文摘要