Recurrent graph optimal transport for learning 3D flow motion in particle tracking
arxiv(2023)
摘要
Flow visualization technologies such as particle tracking velocimetry are broadly used for studying three-dimensional turbulent flow in natural and industrial processes. Despite the advances in three-dimensional acquisition techniques, it is challenging to develop motion estimation algorithms in particle tracking due to large particle displacements, dense particle distributions and high computational cost. We present an end-to-end solution called graph optimal transport (GotFlow3D) to learn the three-dimensional fluid flow motion from consecutive particle images. The proposed model uses a graph neural network to extract geometric features and to further enrich the particle representations. The extracted deep features are subsequently used to correspond particles between consecutive frames, and the flow motion is then iteratively updated with a recurrent neural network approach. Experimental evaluations—including assessments on numerical experiments and validations on real-world experiments—demonstrate that GotFlow3D achieves state-of-the-art performance compared with recently developed scene flow learners and particle tracking algorithms. We believe that the high accuracy, robustness and generalization ability of our method can provide deeper insight into the complex dynamics of many physical and biological systems.
更多查看译文
关键词
Computer science,Fluid dynamics,Imaging techniques,Mechanical engineering,Engineering,general
AI 理解论文
溯源树
样例
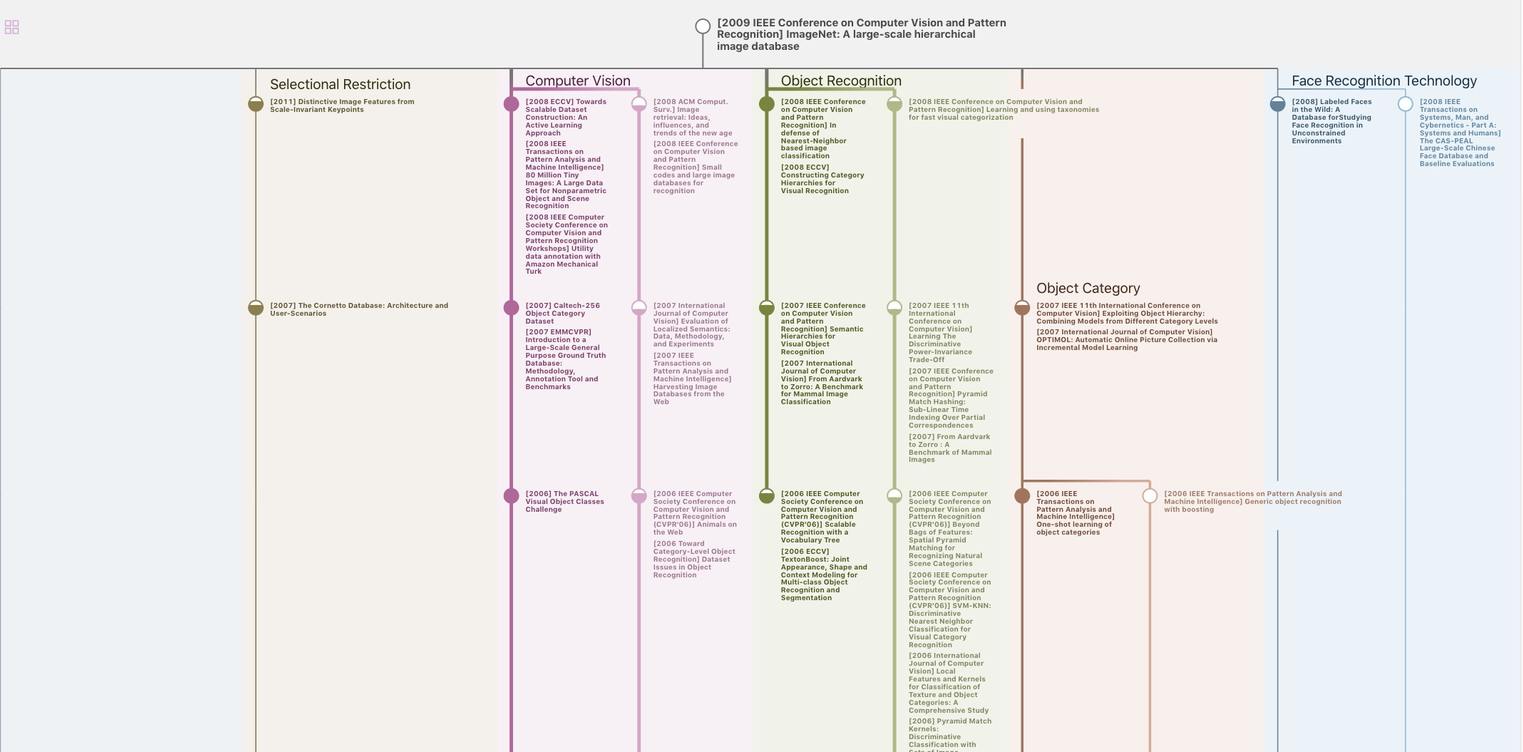
生成溯源树,研究论文发展脉络
Chat Paper
正在生成论文摘要