Applications of machine learning to a compact magnetic spectrometer for high repetition rate, laser-driven particle acceleration
Review of Scientific Instruments(2022)
摘要
Accurately and rapidly diagnosing laser–plasma interactions is often difficult due to the time-intensive nature of the analysis and will only become more so with the rise of high repetition rate lasers and the desire to implement feedback on a commensurate timescale. Diagnostic analysis employing machine learning techniques can help address this problem while maintaining a high degree of accuracy. We report on the application of machine learning to the analysis of a scintillator-based electron spectrometer for experiments on high intensity, laser–plasma interactions at the Colorado State University Advanced Lasers and Extreme Photonics facility. Our approach utilizes a neural network trained on synthetic data and tested on experiments to extract the accelerated electron temperature. By leveraging transfer learning, we demonstrate an improvement in the neural network accuracy, decreasing the network error by 50%.
更多查看译文
关键词
compact magnetic spectrometer,machine learning,particle,high repetition rate,laser-driven
AI 理解论文
溯源树
样例
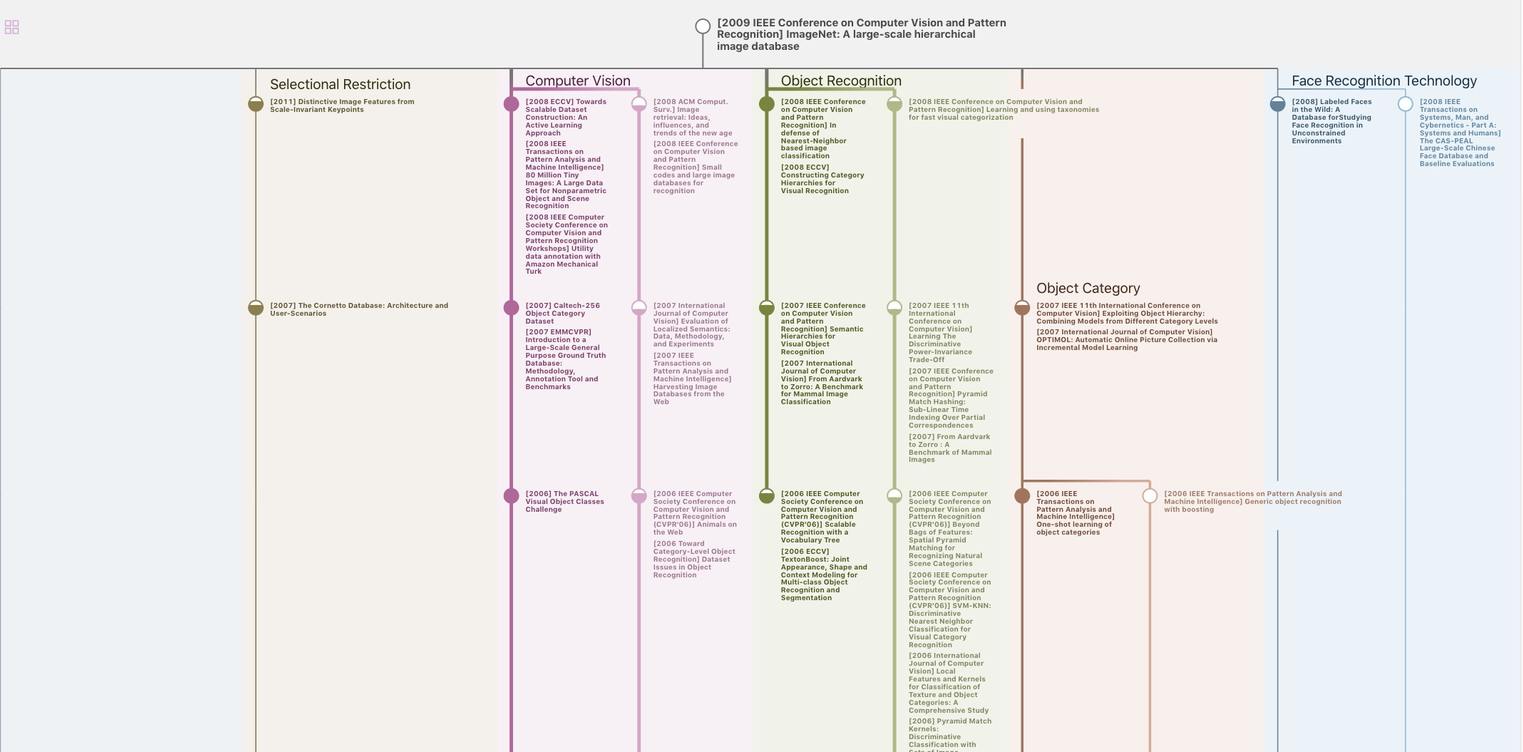
生成溯源树,研究论文发展脉络
Chat Paper
正在生成论文摘要