Contextual-Utterance Training for Automatic Speech Recognition
arxiv(2022)
摘要
Recent studies of streaming automatic speech recognition (ASR) recurrent neural network transducer (RNN-T)-based systems have fed the encoder with past contextual information in order to improve its word error rate (WER) performance. In this paper, we first propose a contextual-utterance training technique which makes use of the previous and future contextual utterances in order to do an implicit adaptation to the speaker, topic and acoustic environment. Also, we propose a dual-mode contextual-utterance training technique for streaming automatic speech recognition (ASR) systems. This proposed approach allows to make a better use of the available acoustic context in streaming models by distilling "in-place" the knowledge of a teacher, which is able to see both past and future contextual utterances, to the student which can only see the current and past contextual utterances. The experimental results show that a conformer-transducer system trained with the proposed techniques outperforms the same system trained with the classical RNN-T loss. Specifically, the proposed technique is able to reduce both the WER and the average last token emission latency by more than 6% and 40ms relative, respectively.
更多查看译文
关键词
automatic speech recognition,training,contextual-utterance
AI 理解论文
溯源树
样例
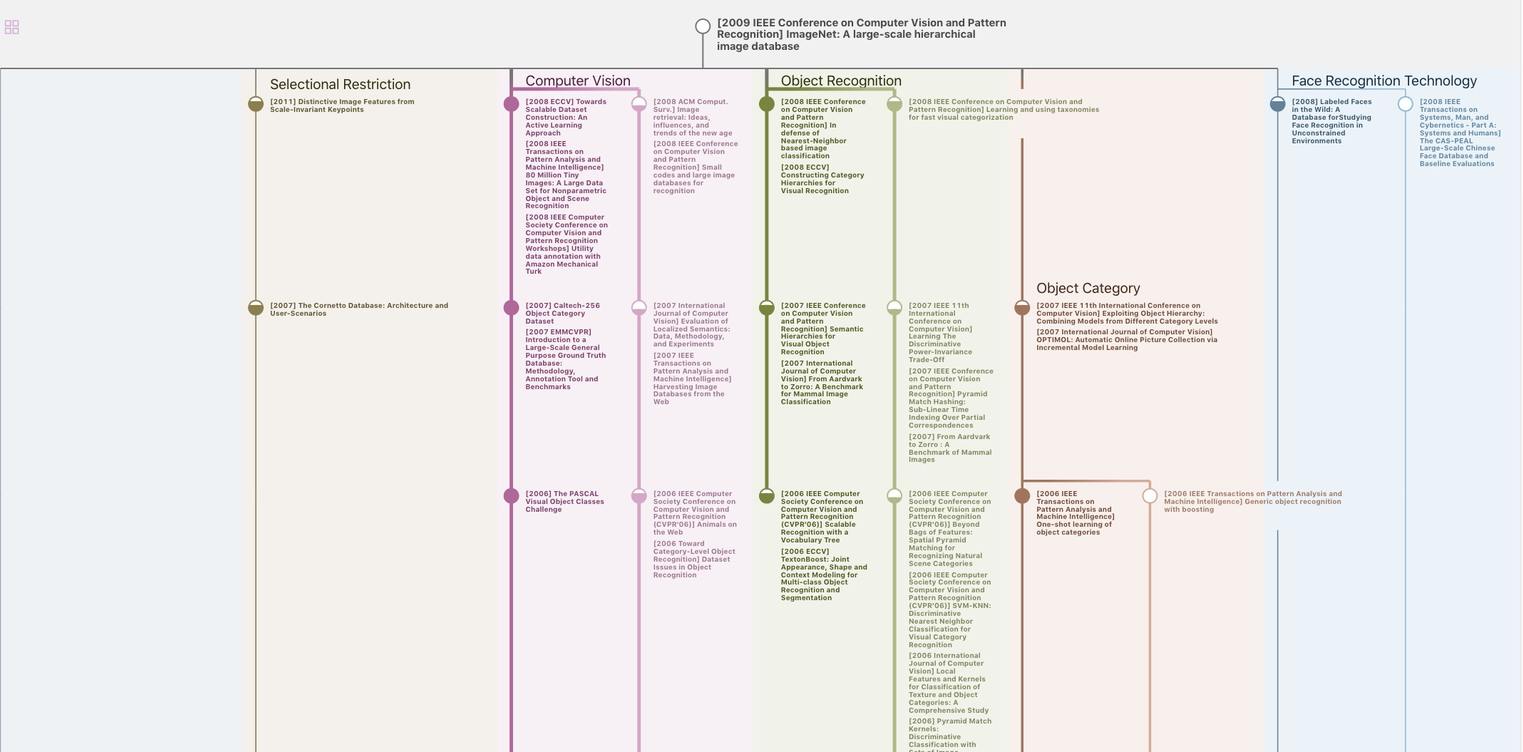
生成溯源树,研究论文发展脉络
Chat Paper
正在生成论文摘要