Joint Uplink-Downlink Capacity and Coverage Optimization via Site-Specific Learning of Antenna Settings
arxiv(2022)
摘要
We propose a novel framework for optimizing antenna parameter settings in a heterogeneous cellular network. We formulate an optimization problem for both coverage and capacity - in both the downlink (DL) and uplink (UL) - which configures the tilt angle, vertical half-power beamwidth (HPBW), and horizontal HPBW of each cell's antenna array across the network. The novel data-driven framework proposed for this non-convex problem, inspired by Bayesian optimization (BO) and differential evolution algorithms, is sample-efficient and converges quickly, while being scalable to large networks. By jointly optimizing DL and UL performance, we take into account the different signal power and interference characteristics of these two links, allowing a graceful trade-off between coverage and capacity in each one. Our experiments on a state-of-the-art 5G NR cellular system-level simulator developed by AT&T Labs show that the proposed algorithm consistently and significantly outperforms the 3GPP default settings, random search, and conventional BO. In one realistic setting, and compared to conventional BO, our approach increases the average sum-log-rate by over 60% while decreasing the outage probability by over 80%. Compared to the 3GPP default settings, the gains from our approach are considerably larger. The results also indicate that the practically important combination of DL throughput and UL coverage can be greatly improved by joint UL-DL optimization.
更多查看译文
关键词
coverage optimization,antenna,uplink-downlink,site-specific
AI 理解论文
溯源树
样例
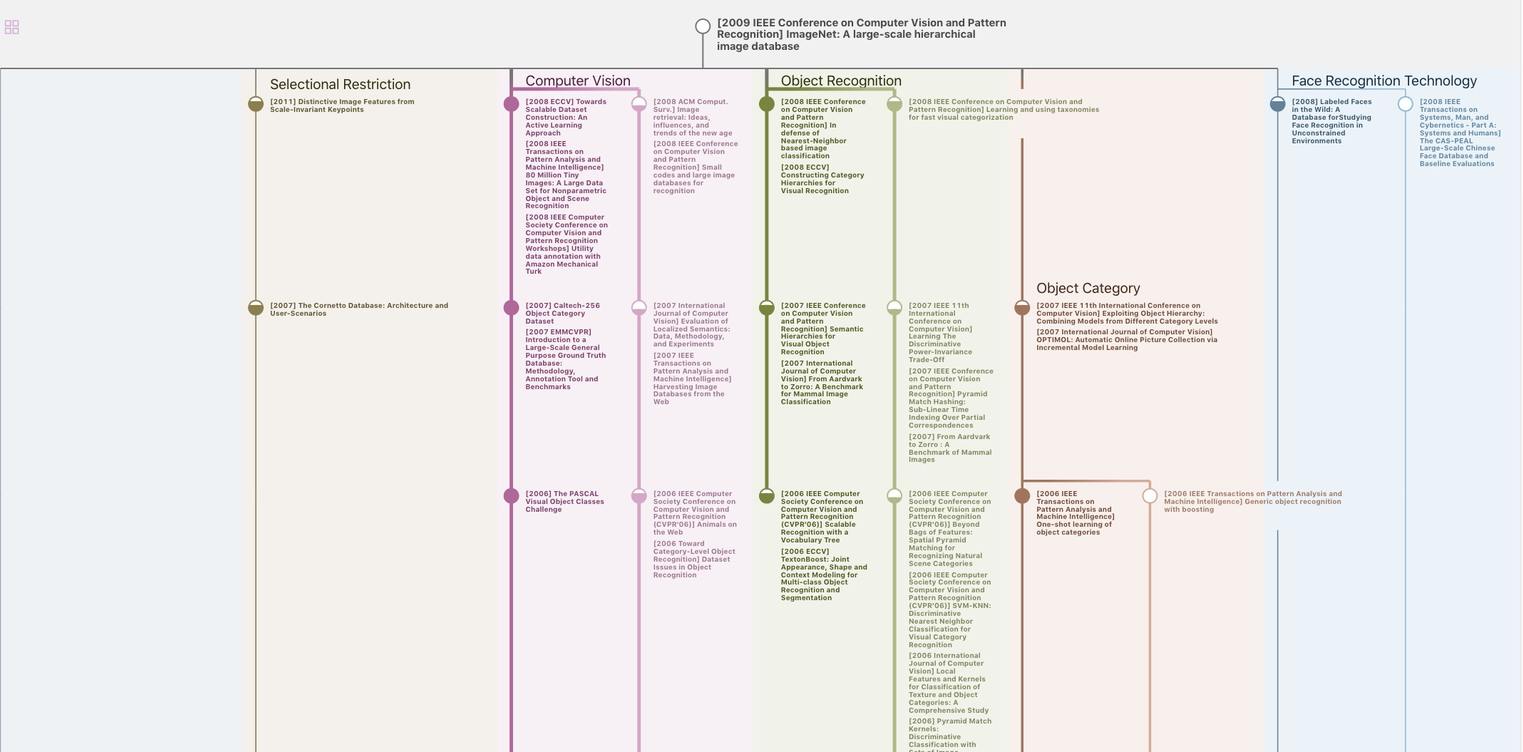
生成溯源树,研究论文发展脉络
Chat Paper
正在生成论文摘要