Meaningful machine learning robustness evaluation in real-world machine learning enabled system contexts
Artificial Intelligence and Machine Learning in Defense Applications IV(2022)
摘要
Applied research presented in this paper describes an approach to provide meaningful evaluation of the Machine Learning (ML) components in a Full Motion Video (FMV) Machine Learning Enabled System ( MLES). The MLES itself is not discussed in the paper. We focus on the experimental activity that has been designed to provide confidence that the MLES, when fielded under dynamic and uncertain conditions, performance will not be undermined by a lack of ML robustness. For example, to real-world changes of the same scene under differing light conditions. The paper details the technical approach and how it is applied to data, across the overall experimental pipeline, consisting of a perturbation engine, test pipeline and metric production. Data is from a small imagery dataset and the results are shown and discussed as part of a proof of concept study.
更多查看译文
关键词
Machine Learning, Object Detection, Verification & Validation, Meaningful Perturbations, Performance Metrics
AI 理解论文
溯源树
样例
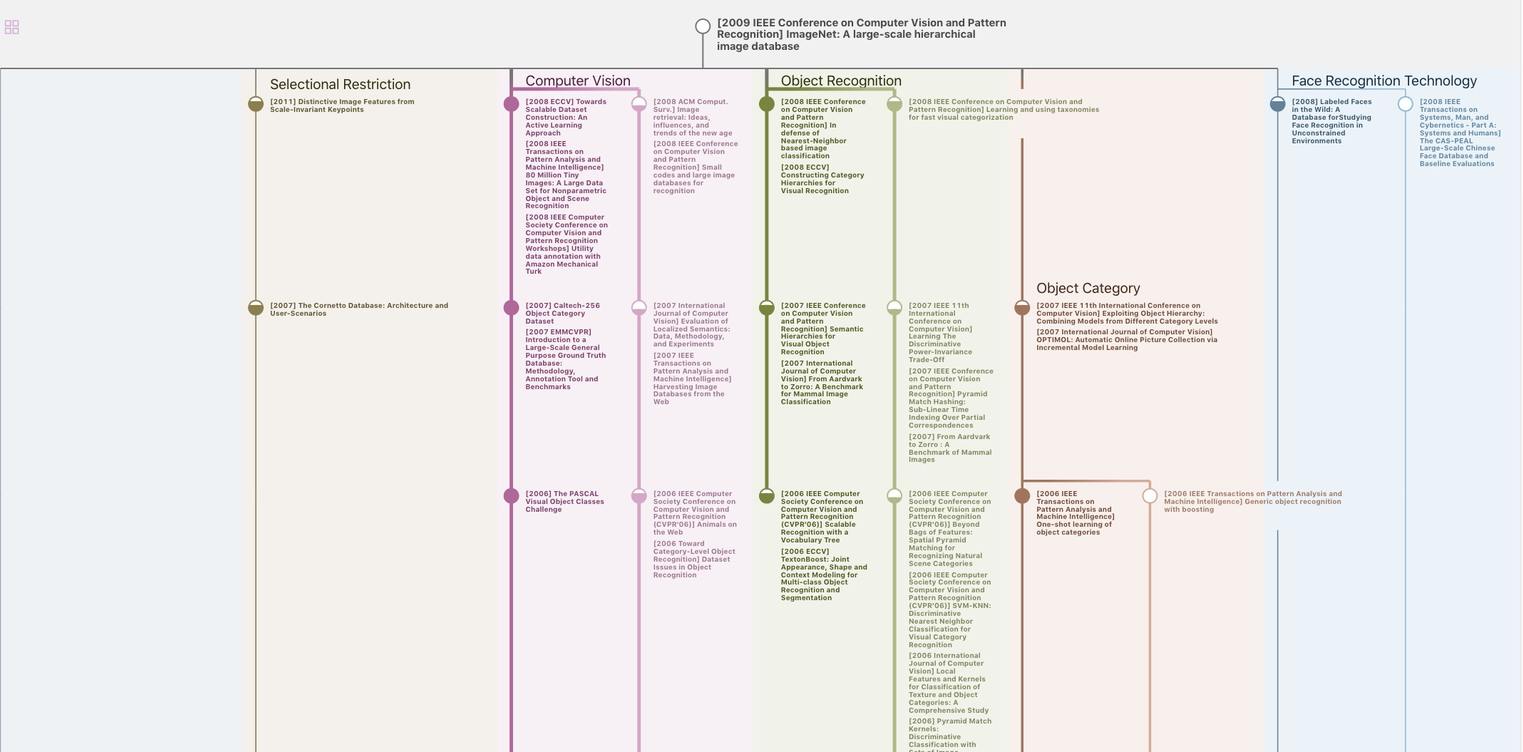
生成溯源树,研究论文发展脉络
Chat Paper
正在生成论文摘要