Improving Exertion and Wellbeing Prediction in Outdoor Running Conditions using Audio-based Surface Recognition.
International Workshop on Multimedia Content Analysis in Sports(2022)
摘要
ABSTRACTTimely detection of runner exertion is crucial for preventing overuse injuries and conditioning training. Similarly, maintaining high levels of wellbeing while running can improve retention rates for onboarders to the sport, with the associated benefits to public health that this entails. Thus, predicting exertion and wellbeing is a promising avenue of research for biomedical sports research. Previous work has shown that exertion and wellbeing can be predicted using biomechanical data collected from wearables attached to the runners' body. However, a particular challenge in outdoor running conditions is the mediating effect of running surface. We experimentally model this mediating effect by using surface-adapted models, which improve prediction rates for both variables. To that end, we investigate the feasibility of using audio-based surface classification to distinguish three main surface categories: gravel, asphalt, and dirt. Our best models achieve an unweighted average recall (UAR) of .619 and a UAR of .690 on our session-independent and session-dependent test set, respectively, which is an improvement over the .363 UAR achieved by a GPS-based approximation.
更多查看译文
AI 理解论文
溯源树
样例
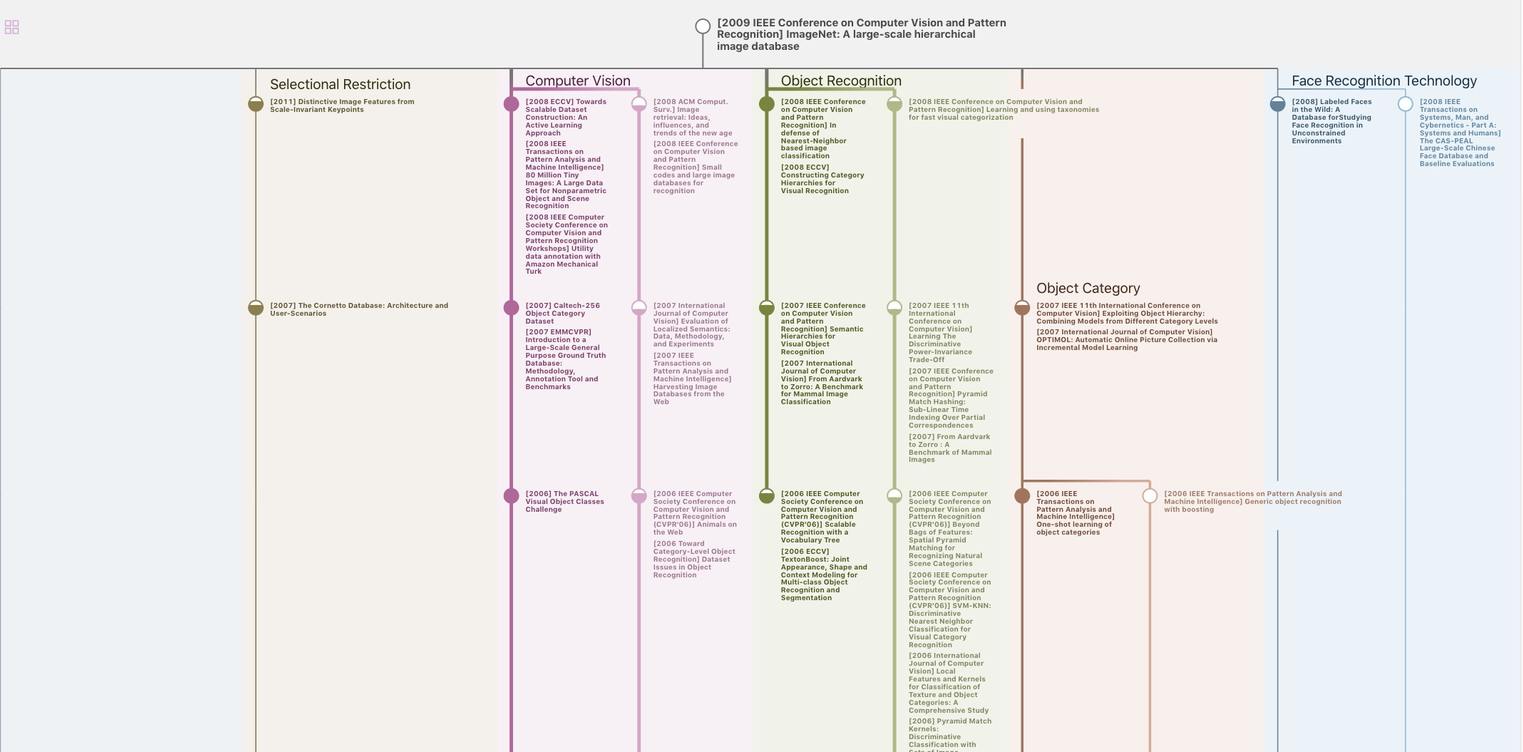
生成溯源树,研究论文发展脉络
Chat Paper
正在生成论文摘要