Predicting Analyte Concentrations from Electrochemical Aptasensor Signals Using LSTM Recurrent Networks
BIOENGINEERING-BASEL(2022)
摘要
Nanomaterial-based aptasensors are useful devices capable of detecting small biological species. Determining suitable signal processing methods can improve the identification and quantification of target analytes detected by the biosensor and consequently improve the biosensor's performance. In this work, we propose a data augmentation method to overcome the insufficient amount of available original data and long short-term memory (LSTM) to automatically predict the analyte concentration from part of a signal registered by three electrochemical aptasensors, with differences in bioreceptors, analytes, and the signals' lengths for specific concentrations. To find the optimal network, we altered the following variables: the LSTM layer structure (unidirectional LSTM (LSTM) and bidirectional LSTM (BLSTM)), optimizers (Adam, RMSPROP, SGDM), number of hidden units, and amount of augmented data. Then, the evaluation of the networks revealed that the highest original data accuracy increased from 50% to 92% by exploiting the data augmentation method. In addition, the SGDM optimizer showed a lower performance prediction than that of the ADAM and RMSPROP algorithms, and the number of hidden units was ineffective in improving the networks' performances. Moreover, the BLSTM nets showed more accurate predictions than those of the ULSTM nets on lengthier signals. These results demonstrate that this method can automatically detect the analyte concentration from the sensor signals.
更多查看译文
关键词
data augmentation, multi-class classifiers, classification, deep learning, long short-term memory neural networks, unidirectional LSTM, bidirectional LSTM, time-series aptasensor signal
AI 理解论文
溯源树
样例
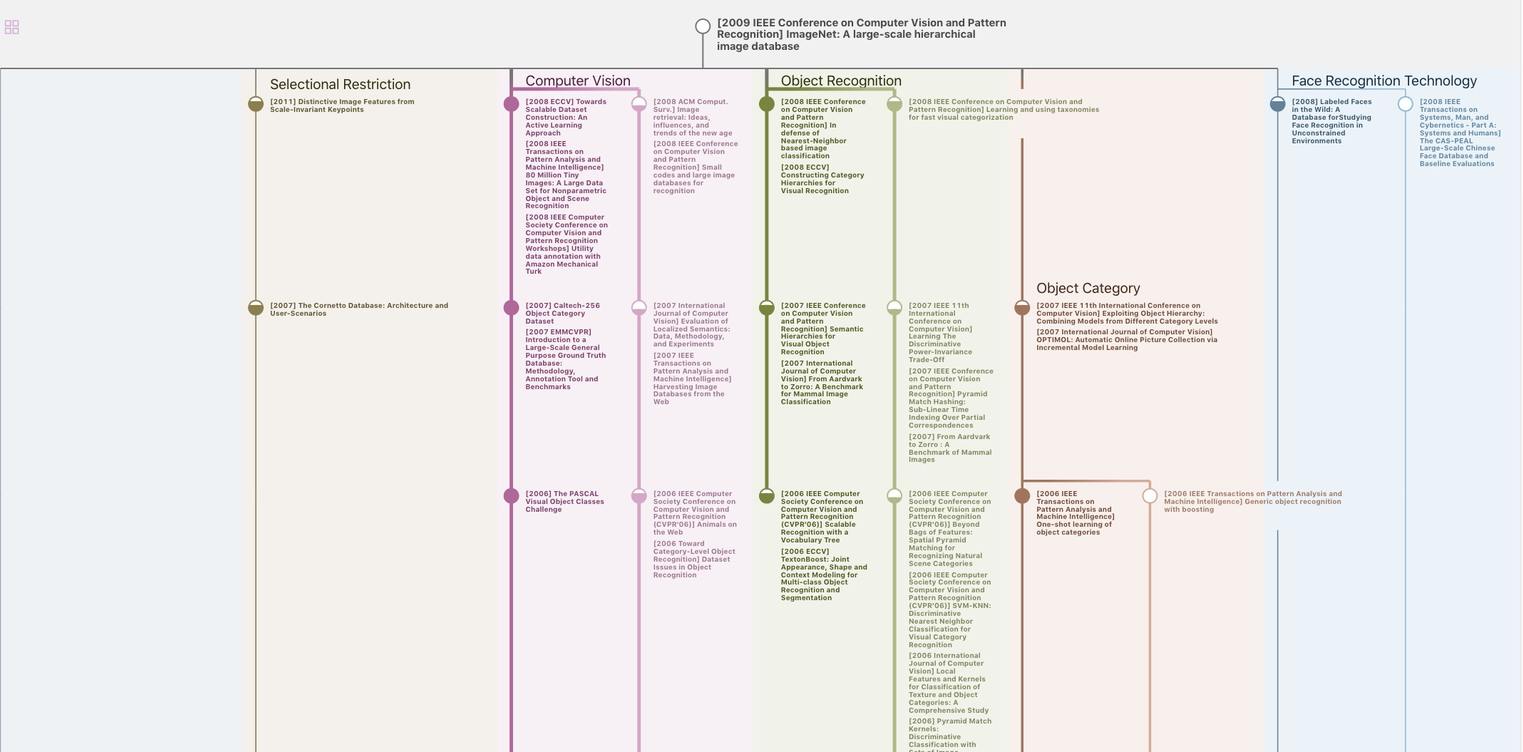
生成溯源树,研究论文发展脉络
Chat Paper
正在生成论文摘要