Classification of High-Dimensionality Data Using Machine Learning Techniques
Intelligent System Design(2022)
摘要
In the digitization world, a large volume of information is being produced across a few areas such as medical services, creation, Web, and associations. Machine learning techniques are utilized to reveal designs among the train of this information for decision-making. Not all the features in the datasets produced are significant for preparing the machine learning (ML) algorithms. A few features may be not important and some probably won’t influence the result of the forecast. Disregarding or eliminating these immaterial or less significant features reduces the weight on ML algorithms. In this work, principal component analysis (PCA) is researched on different most popular ML algorithms, Naïve Bayes, support vector machine (SVM) classifier and KNN classifier, using freely accessible MINIST dataset. Experimentation results demonstrate that ML algorithms with PCA produce better outcomes when dimensionality of the datasets is high.
更多查看译文
关键词
Dimensionality reduction, KNN, Machine learning, Naïve Bayes, PCA, SVM
AI 理解论文
溯源树
样例
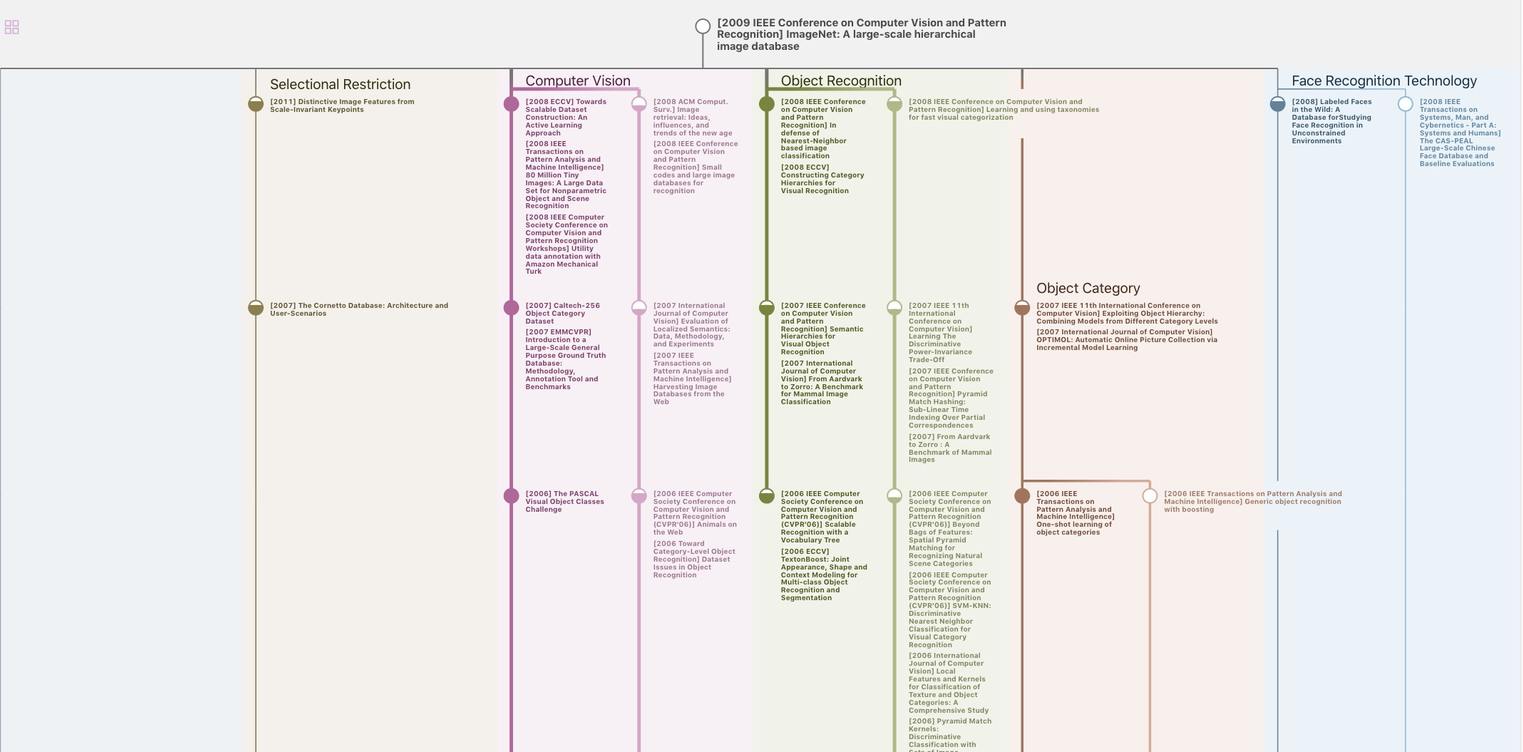
生成溯源树,研究论文发展脉络
Chat Paper
正在生成论文摘要