Imagined Speech Recognition in a Subject Independent Approach Using a Prototypical Network
XLV Mexican Conference on Biomedical Engineering(2022)
摘要
Brain-computer interface (BCI) systems have gained significant interest given the different biomedical applications in which they can be used to help disabled individuals to communicate or control external devices. Imagined speech is related to BCI systems controlled only by thinking about a vowel, phoneme, or word without any physical movement. In this paper, a Prototypical Network approach, named Proto-imEEG, is presented for the automatic classification of seven imagined phonemic/syllabic prompts and four imagined words by analyzing EEG data of the KaraOne dataset. The Prototypical Network is selected because of its ability to learn from a few samples, a common issue in EEG data. The embedding function of our Prototypical Network is based on a 1D-convolutional layer and bidirectional recurrent networks. The average classification accuracy achieved by Proto-imEEG is 92.04% and 96% by using a Long-Short Term Memory or a Gated Recurrent Unit, respectively, with an average inference time of 0.2 s. These results demonstrate superior performance to state-of-the-art methods in classifying the eleven classes of the KaraOne dataset. As far as we know, this is the first time that a Prototypical Network approach is used in imagined speech classification tasks.
更多查看译文
关键词
Imagined speech, Prototypical networks, KaraOne dataset, EEG data
AI 理解论文
溯源树
样例
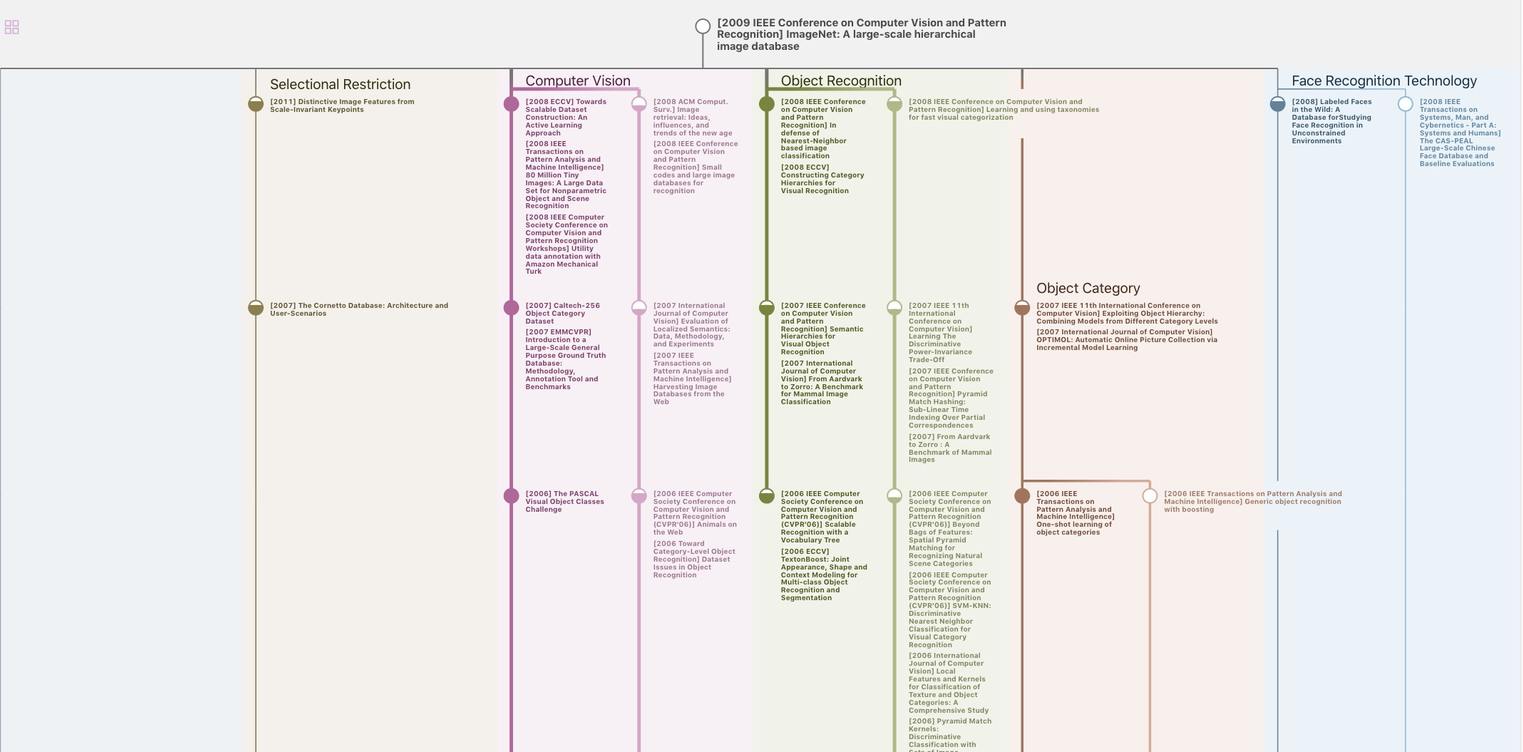
生成溯源树,研究论文发展脉络
Chat Paper
正在生成论文摘要