Neural Collapse in Deep Homogeneous Classifiers and The Role of Weight Decay.
IEEE International Conference on Acoustics, Speech, and Signal Processing (ICASSP)(2022)
摘要
Neural Collapse is a phenomenon recently discovered in deep classifiers where the last layer activations collapse onto their class means, while the means and last layer weights take on the structure of dual equiangular tight frames. In this paper we present results showing the role of weight decay in the emergence of Neural Collapse in deep homogeneous networks. We show that certain near-interpolating minima of deep networks satisfy the Neural Collapse condition, and this can be derived from the gradient flow on the regularized square loss. We also show that weight decay is necessary for neural collapse to occur. We support our theoretical analysis with experiments that confirm our results.
更多查看译文
关键词
Deep Learning,Inductive Bias,Neural Collapse,Weight Decay,Classification
AI 理解论文
溯源树
样例
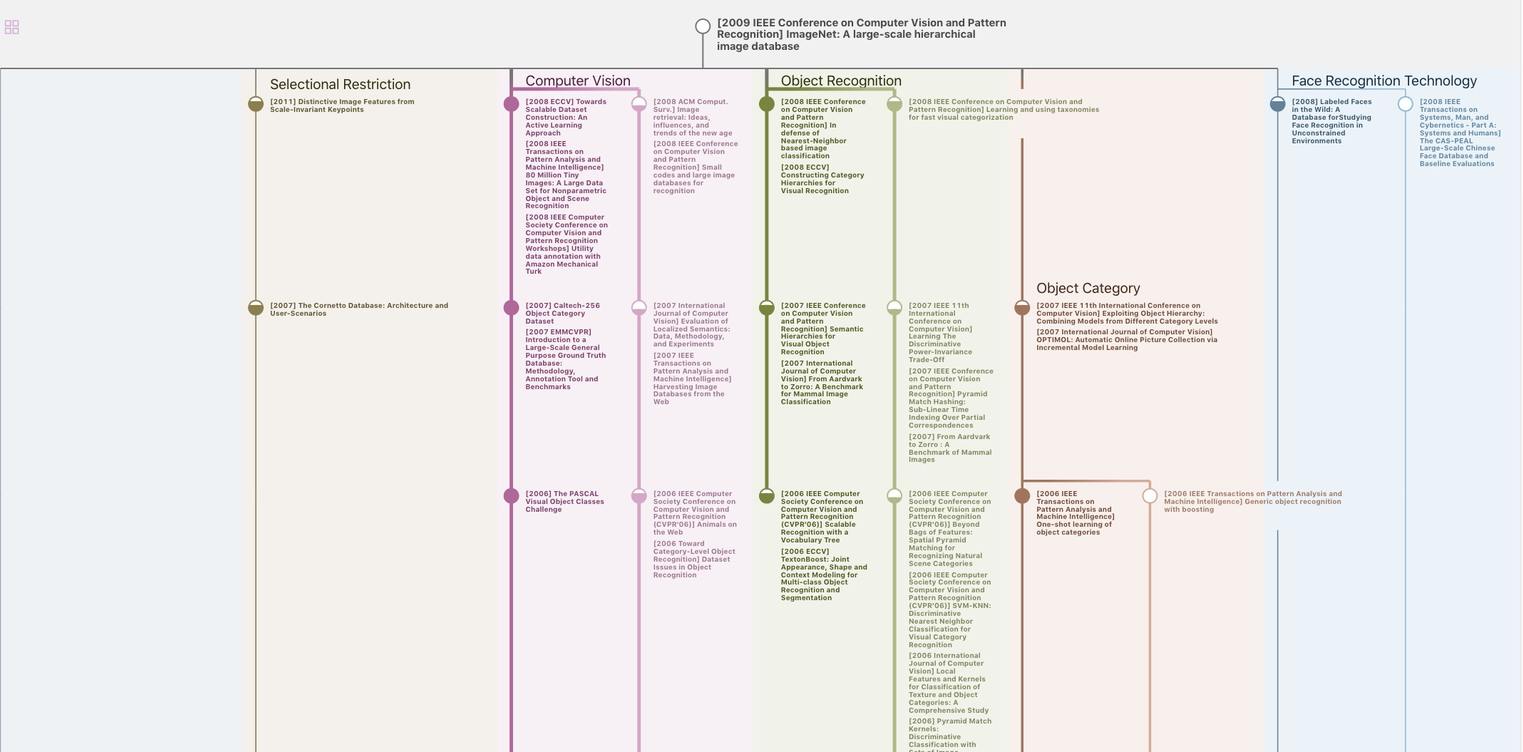
生成溯源树,研究论文发展脉络
Chat Paper
正在生成论文摘要