Free Lunch for Cross-Domain Occluded Face Recognition without Source Data.
IEEE International Conference on Acoustics, Speech, and Signal Processing (ICASSP)(2022)
摘要
Most recognizing occluded faces methods focus on synthetic-occluded faces for training due to the lack of real-occluded data. However, the performance may suffer from degradation since the synthetic-occluded and real-occluded face images are under different distributions. Hence, it draws our eyes to transfer the model from the synthetic to the real-world domain. In this paper, we propose a source data-free domain adaptive occluded face recognition framework to optimize the network in the target domain via redefining it as a pseudo labels denoising problem. To obtain reliable pseudo labels, we train synthetic-occluded and non-occluded images via distribution alignment to extract occlusion-robust features. Nonetheless, completely correct labels are still unattainable. Then, a denoising strategy is proposed to optimize pseudo labels by centroid-based feature clustering. Experiments show that the proposed approach can effectively recognize the real-occluded face; it also reminds the occluded faces recognition community about the feasibility of domain adaptation in existing tasks.
更多查看译文
关键词
occluded face recognition,adversarial learning,domain adaptation,source data-free
AI 理解论文
溯源树
样例
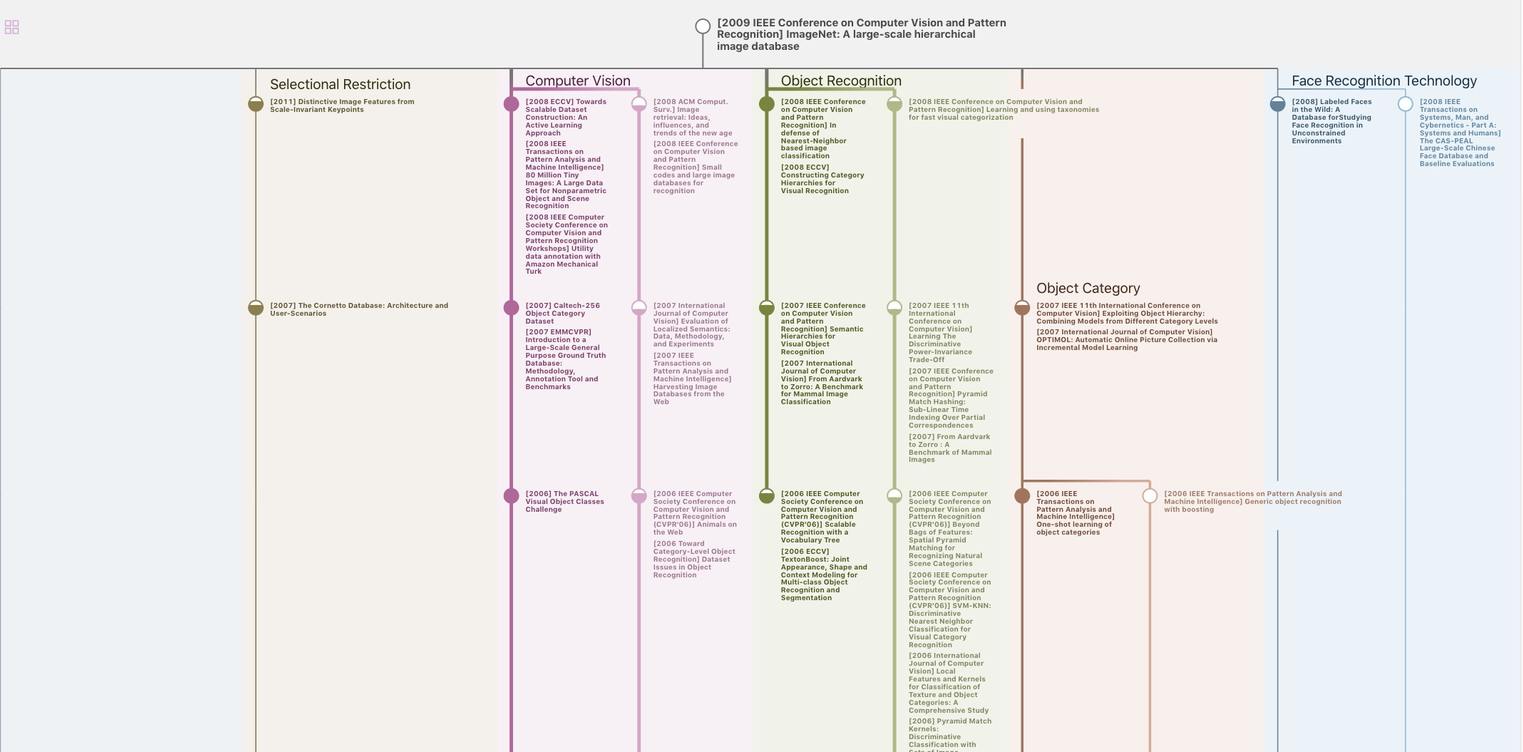
生成溯源树,研究论文发展脉络
Chat Paper
正在生成论文摘要