Cross-Domain Few-Shot Learning for Rare-Disease Skin Lesion Segmentation.
IEEE International Conference on Acoustics, Speech, and Signal Processing (ICASSP)(2022)
摘要
Recently, deep learning (DL)-based skin lesion segmentation in dermoscopic images has advanced the efficient diagnosis of skin diseases. Commonly, most of the DL-based methods require a large amount of training data and can only perform accurate predictions on pre-defined classes. However, there exist some rare skin diseases with very limited labeled samples, which poses great challenges to typical DL-based methods. Few-shot learning (FSL) technique, which aims to train models with abundant seen classes and then generalizes to related unseen classes, is promising in addressing a similar problem. Unfortunately, simply borrowing the typical FSL is infeasible since collecting such abundant seen-class data (common skin diseases), is also difficult. In this paper, we propose a cross-domain few-shot segmentation (CD-FSS) framework, which enables the model to leverage the learning ability obtained from the natural domain, to facilitate rare-disease skin lesion segmentation with limited data of common diseases. Specifically, the framework consists of two processes, i.e., specific learning and generic learning, which are alternately optimized in a meta-training manner. A specific learner and a generic learner are tailored to build relationships between both processes. Experimental results demonstrate that our framework significantly improves the generalization ability from natural domain to unseen medical domain.
更多查看译文
关键词
Few-shot segmentation,Cross-domain,Skin lesion
AI 理解论文
溯源树
样例
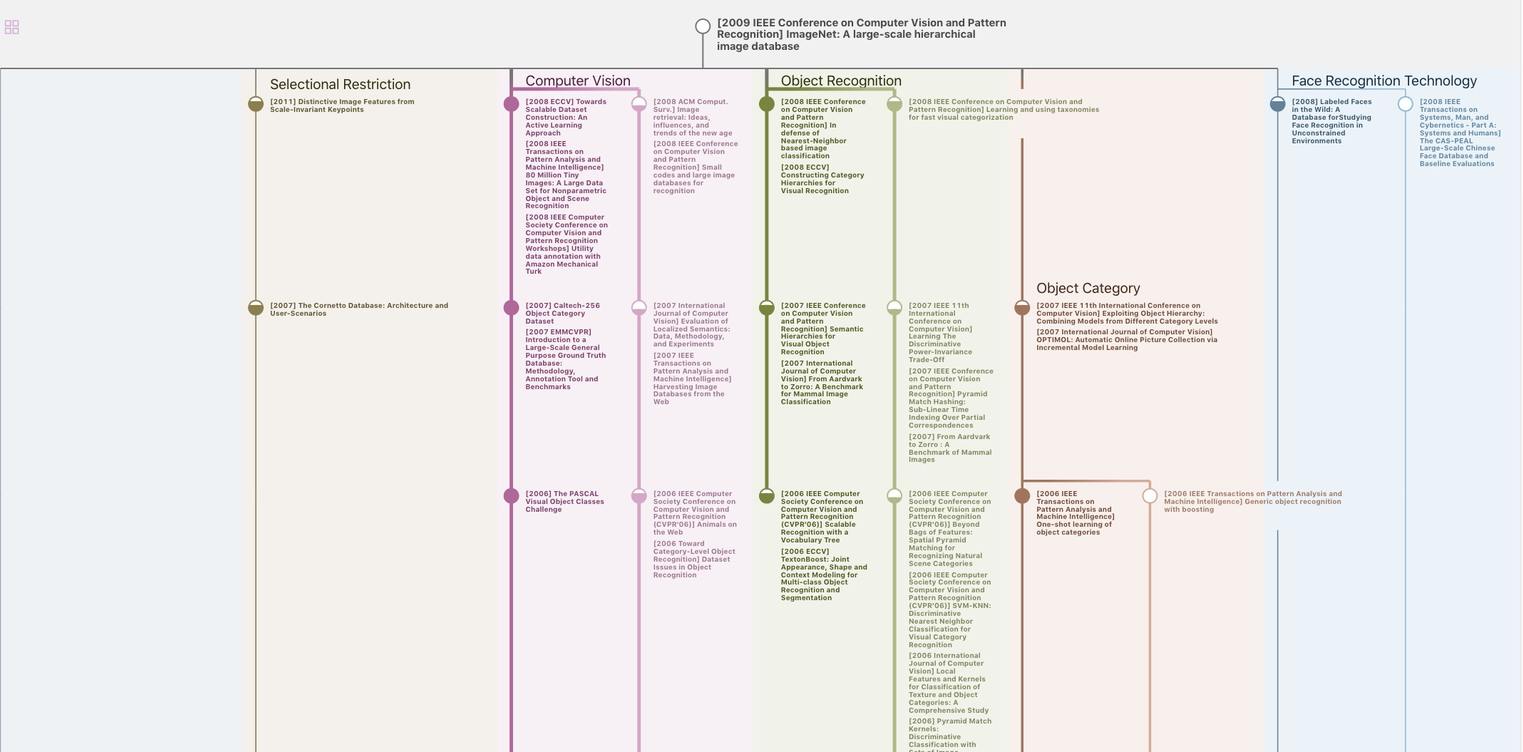
生成溯源树,研究论文发展脉络
Chat Paper
正在生成论文摘要