Deep Neural Network (DNN) Audio Coder Using A Perceptually Improved Training Method.
IEEE International Conference on Acoustics, Speech, and Signal Processing (ICASSP)(2022)
摘要
A new end-to-end audio coder based on a deep neural network (DNN) is proposed. To compensate for the perceptual distortion that occurred by quantization, the proposed coder is optimized to minimize distortions in both signal and perceptual domains. The distortion in the perceptual domain is measured using the psychoacoustic model (PAM), and a loss function is obtained through the two-stage compensation approach. Also, the scalar uniform quantization was approximated using a uniform stochastic noise, together with a compression-decompression scheme, which provides simpler but more stable learning without an additional penalty than the softmax quantizer. Test results showed that the proposed coder achieves more accurate noise-masking than the previous PAM-based method and better perceptual quality then the MP3 audio coder.
更多查看译文
关键词
DNN-based Audio Coder,PAM,Perceptual Loss Function
AI 理解论文
溯源树
样例
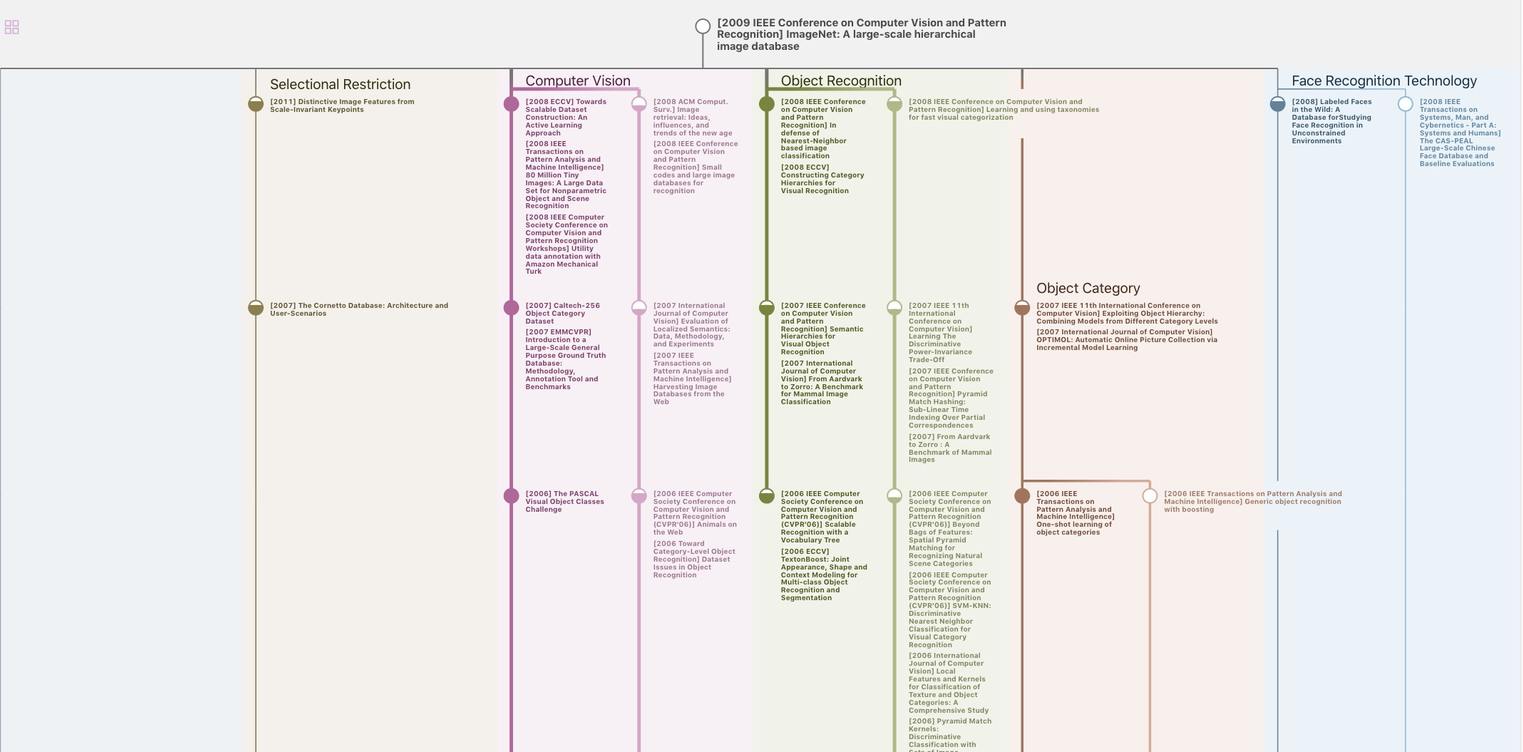
生成溯源树,研究论文发展脉络
Chat Paper
正在生成论文摘要