IDEAL: Improved DEnse locAL Contrastive Learning for Semi-Supervised Medical Image Segmentation
arxiv(2023)
摘要
Due to the scarcity of labeled data, Contrastive Self-Supervised Learning (SSL) frameworks have lately shown great potential in several medical image analysis tasks. However, the existing contrastive mechanisms are sub-optimal for dense pixel-level segmentation tasks due to their inability to mine local features. To this end, we extend the concept of metric learning to the segmentation task, using a dense (dis)similarity learning for pre-training a deep encoder network, and employing a semi-supervised paradigm to fine-tune for the downstream task. Specifically, we propose a simple convolutional projection head for obtaining dense pixel-level features, and a new contrastive loss to utilize these dense projections thereby improving the local representations. A bidirectional consistency regularization mechanism involving two-stream model training is devised for the downstream task. Upon comparison, our IDEAL method outperforms the SoTA methods by fair margins on cardiac MRI segmentation. Code available: https://github.com/hritam-98/IDEAL-ICASSP23
更多查看译文
关键词
learning,local,semi-supervised
AI 理解论文
溯源树
样例
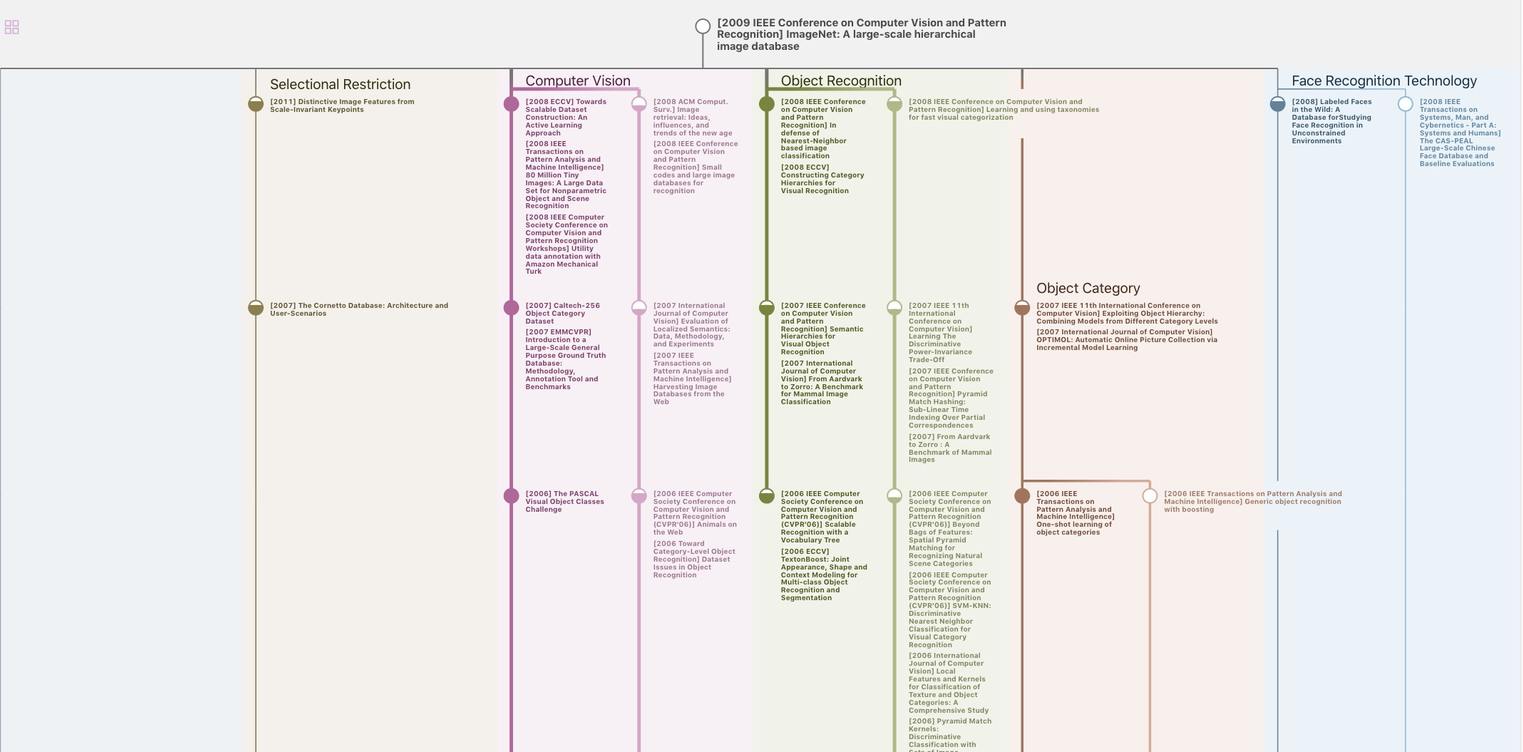
生成溯源树,研究论文发展脉络
Chat Paper
正在生成论文摘要