Improving the Tractability of SVC-based Robust Optimization
IFAC-PapersOnLine(2022)
摘要
Support Vector Clustering (SVC) has been proposed in the literature as a data-driven approach to build uncertainty sets in robust optimization. Unfortunately, the resulting SVC-based uncertainty sets induces a large number of additional variables and constraints in the robust counterpart of mathematical formulations. We propose two methods to approximate the resulting uncertainty sets and overcome these tractability issues. We evaluate these approaches on a production planning problem inspired from an industrial case study. The results obtained are compared with those of the SVC-based uncertainty set and the well known budget-based uncertainty set. We find that the approximated uncertainty set based formulation can be solved much faster than the SVC-based formulation. Still, the obtained solutions are comparable to the SVC-based solutions in term of performance.
更多查看译文
关键词
Data-driven,Robust Optimization,Production planning
AI 理解论文
溯源树
样例
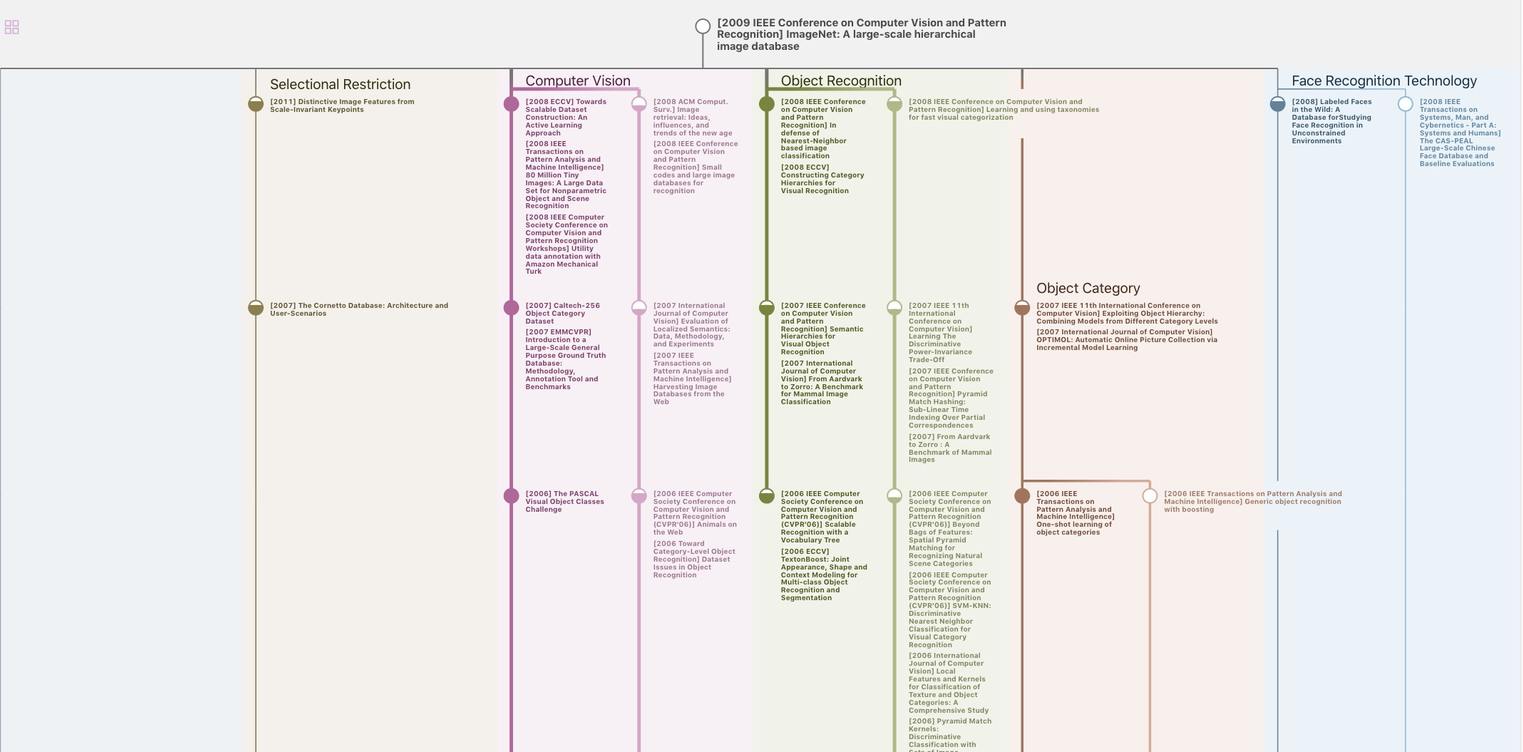
生成溯源树,研究论文发展脉络
Chat Paper
正在生成论文摘要