PredNAS: A Universal and Sample Efficient Neural Architecture Search Framework
arxiv(2022)
摘要
In this paper, we present a general and effective framework for Neural Architecture Search (NAS), named PredNAS. The motivation is that given a differentiable performance estimation function, we can directly optimize the architecture towards higher performance by simple gradient ascent. Specifically, we adopt a neural predictor as the performance predictor. Surprisingly, PredNAS can achieve state-of-the-art performances on NAS benchmarks with only a few training samples (less than 100). To validate the universality of our method, we also apply our method on large-scale tasks and compare our method with RegNet on ImageNet and YOLOX on MSCOCO. The results demonstrate that our PredNAS can explore novel architectures with competitive performances under specific computational complexity constraints.
更多查看译文
关键词
search
AI 理解论文
溯源树
样例
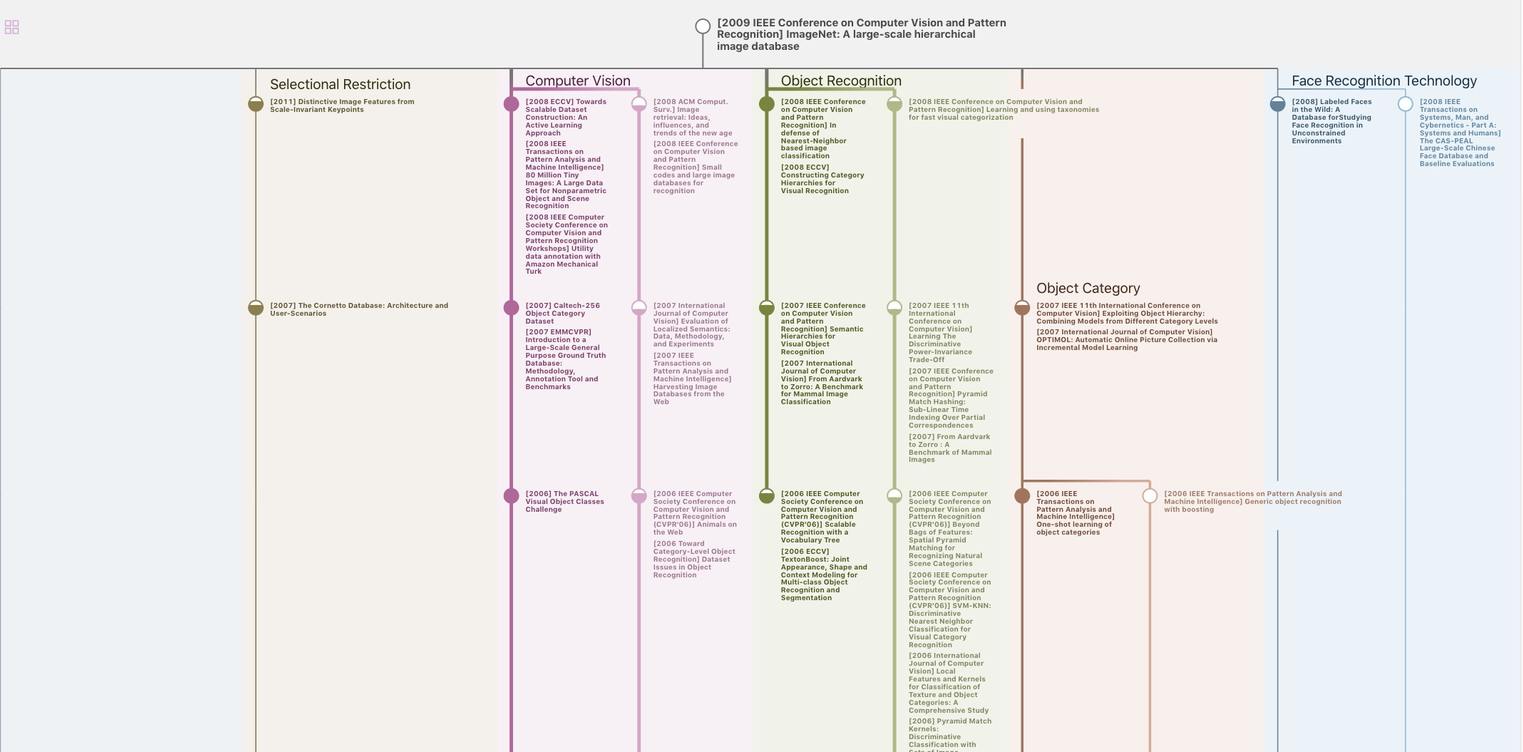
生成溯源树,研究论文发展脉络
Chat Paper
正在生成论文摘要