Surface Damage Identification for Heritage Site Protection: A Mobile Crowd-sensing Solution Based on Deep Learning
Journal on Computing and Cultural Heritage(2023)
摘要
This article addresses the general problem of built heritage protection against both deterioration and loss. To continuously monitor and update the structural health status, a crowd-sensing solution based on powerful and automatic deep learning technique is proposed. The aim of this solution is to get rid of the limitations of manual and visual damage detection methods that are costly and time-consuming. Instead, automatic visual inspection for damage detection on walls is efficiently and effectively performed using an embedded Convolutional Neural Network (CNN). This CNN detects the most frequent types of surface damage on wall photos. The study has been conducted in the Kasbah of Algiers where the four following types of damages have been considered: Efflorescence, spall, crack, and mold. The CNN is designed and trained to be integrated into a mobile application for a participatory crowd-sensing solution. The application should be widely and freely deployed, so any user can take a picture of a suspected damaged wall and get an instant and automatic diagnosis through the embedded CNN. In this context, we have chosen MobileNetV2 with a transfer learning approach. A set of real images have been collected and manually annotated and have been used for training, validation, and test. Extensive experiments have been conducted to assess the efficiency and the effectiveness of the proposed solution, using a 5-fold cross-validation procedure. Obtained results show in particular a mean weighted average precision of 0.868 +/- 0.00862 (with a 99% of confidence level) and a mean weighted average recall of 0.84 +/- 0.00729 (with a 99% of confidence level). To evaluate the performance of MobileNetV2 as a feature extractor, we conducted a comparative study with other small backbones. Further analysis of CNN activation using Grad-Cam has also been done. Obtained results show that our method remains effective even when using a small network and medium- to low-resolution images. MobileNetV2-based CNN size is smaller, and computational cost better, compared to the other CNNs, with similar performance results. Finally, detected surface damages have also been plotted on a geographic map, giving a global view of their distribution.
更多查看译文
关键词
Built heritage,surface damage classification,structural health monitoring,deep learning,CNN,data augmentation,small dataset,multi-label classification,K-fold cross-validation,crowd-sensing,GRAD-CAM,geographic mapping
AI 理解论文
溯源树
样例
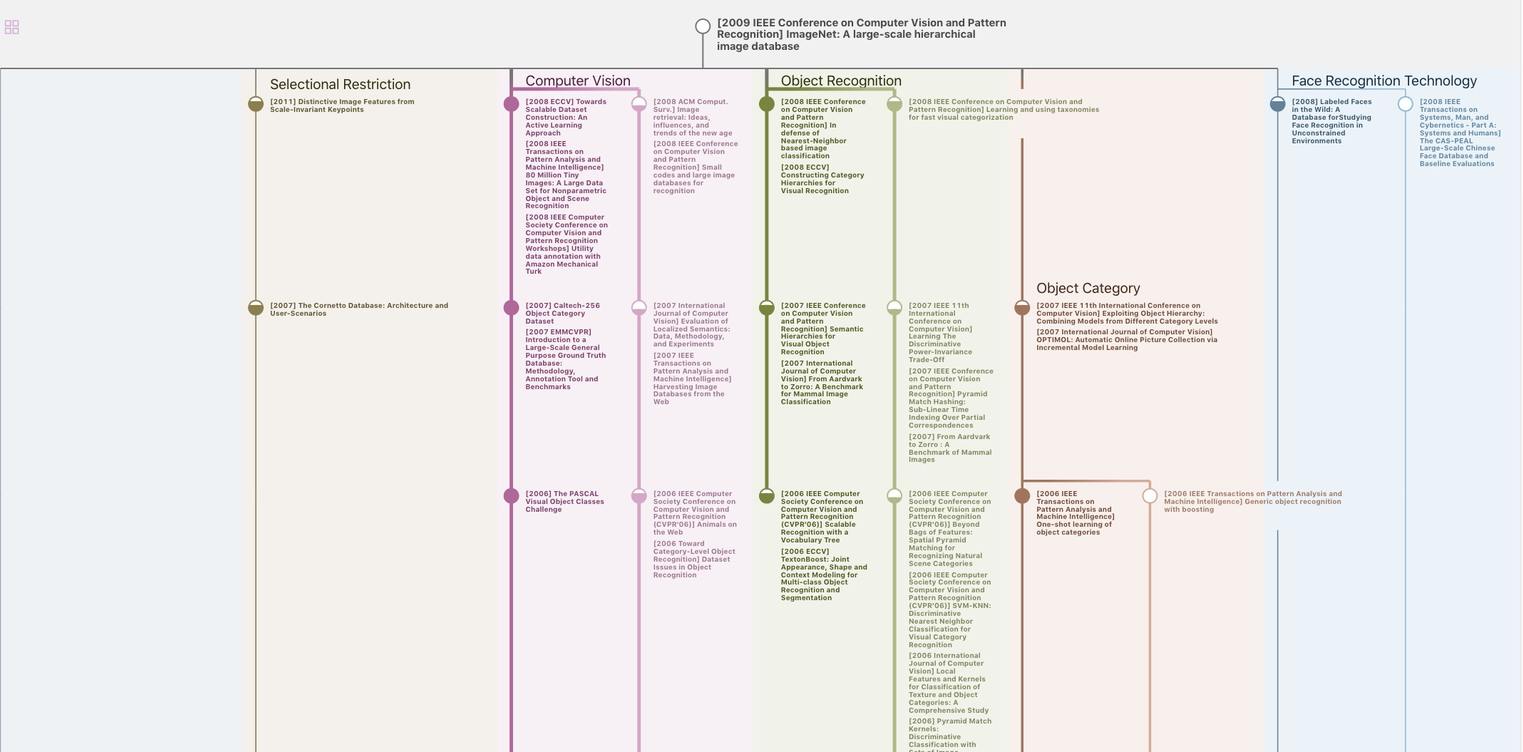
生成溯源树,研究论文发展脉络
Chat Paper
正在生成论文摘要