From Points to Functions: Infinite-dimensional Representations in Diffusion Models
arxiv(2022)
摘要
Diffusion-based generative models learn to iteratively transfer unstructured noise to a complex target distribution as opposed to Generative Adversarial Networks (GANs) or the decoder of Variational Autoencoders (VAEs) which produce samples from the target distribution in a single step. Thus, in diffusion models every sample is naturally connected to a random trajectory which is a solution to a learned stochastic differential equation (SDE). Generative models are only concerned with the final state of this trajectory that delivers samples from the desired distribution. Abstreiter et. al showed that these stochastic trajectories can be seen as continuous filters that wash out information along the way. Consequently, it is reasonable to ask if there is an intermediate time step at which the preserved information is optimal for a given downstream task. In this work, we show that a combination of information content from different time steps gives a strictly better representation for the downstream task. We introduce an attention and recurrence based modules that ``learn to mix'' information content of various time-steps such that the resultant representation leads to superior performance in downstream tasks.
更多查看译文
关键词
representation learning,diffusion models,score-based learning
AI 理解论文
溯源树
样例
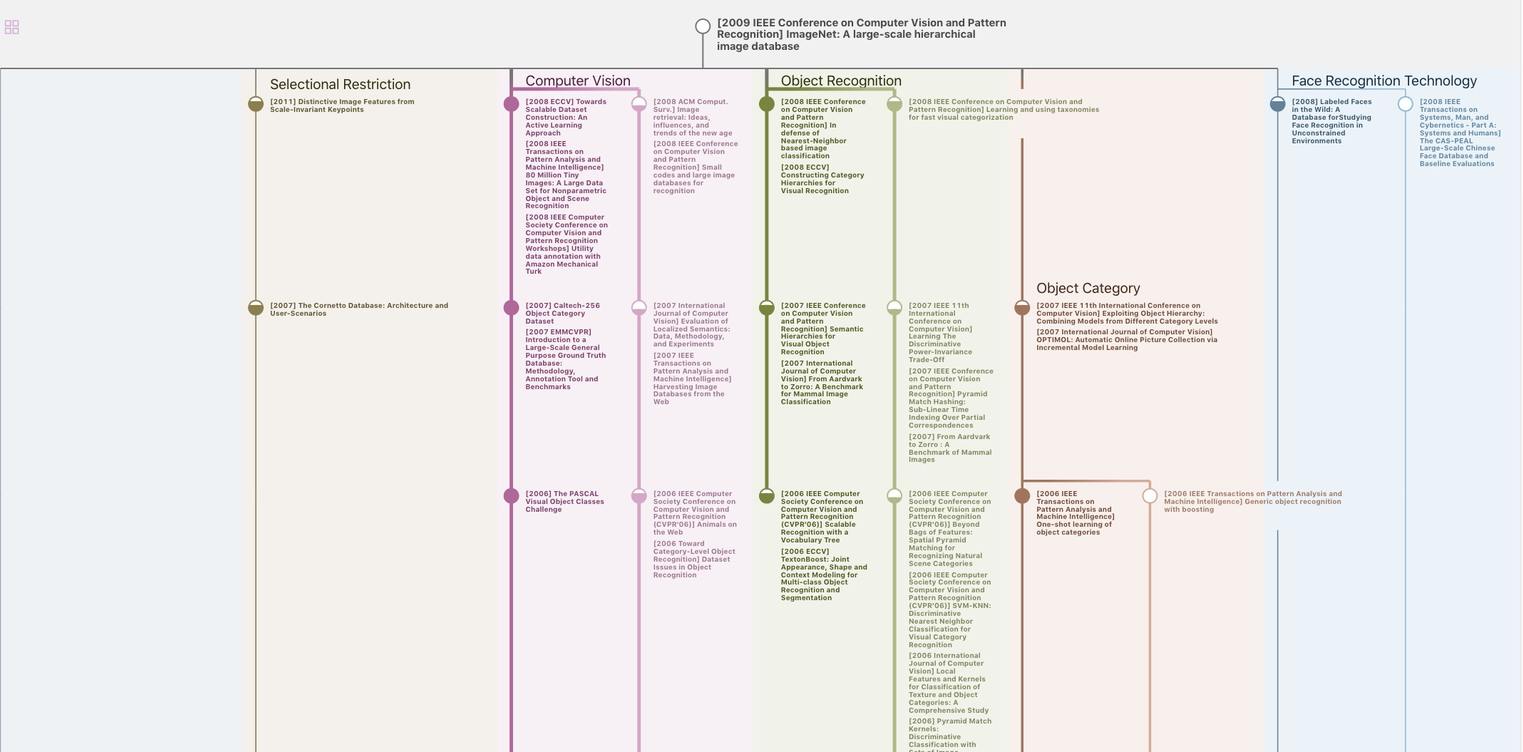
生成溯源树,研究论文发展脉络
Chat Paper
正在生成论文摘要