Improving Rainfall-Runoff Model Reliability Under Nonstationarity of Model Parameters: A Hypothesis Testing Based Framework
Water Resources Research(2022)
摘要
Rainfall-runoff models are used to project the impact of climate change on water resources. Often, models are parameterized over historical climate prior to simulating runoff under changed climates. An increasing body of literature indicates that this assumption of model parameter invariance to climate can introduce significant simulation biases as projected climate deviates from historical climate. One way to consider the effect of climate on model parameterization is to regionalize model parameters using historical data and use that information to project parameters' probable distribution under a changed climate. These approaches, termed trading space-for-time (TSFT), have shown promising results when simulating mean annual runoff, but their ability to reproduce time varying daily runoff response remains unexplored. Here, we design experiments to identify possible ways to account for the influence of climate on model parameters by building upon a recently developed Whittaker-biome-based TSFT framework. We perform 35 experiments, each representing different hypotheses regarding how model parameters may be altered with climate. We test these hypotheses for seven watersheds across the conterminous United States that undergo significant climate change between 1980-1990 and 1999-2009. Our results show that updated parameters for the best experiment increased median Nash-Sutcliffe Efficiency for the two most climate-impacted watersheds in our analyses from 0.37 to 0.35 to 0.64 and 0.49, respectively. Improvements in simulation performance for watersheds transitioning from the boreal forest and the woodland/shrubland biomes to the temperate seasonal forest biome were attributed to altered snow and routing parameters, respectively.
更多查看译文
关键词
rainfall-runoff model nonstationarity,trading space-for-time,Whittaker biomes,climate change,snow hydrology
AI 理解论文
溯源树
样例
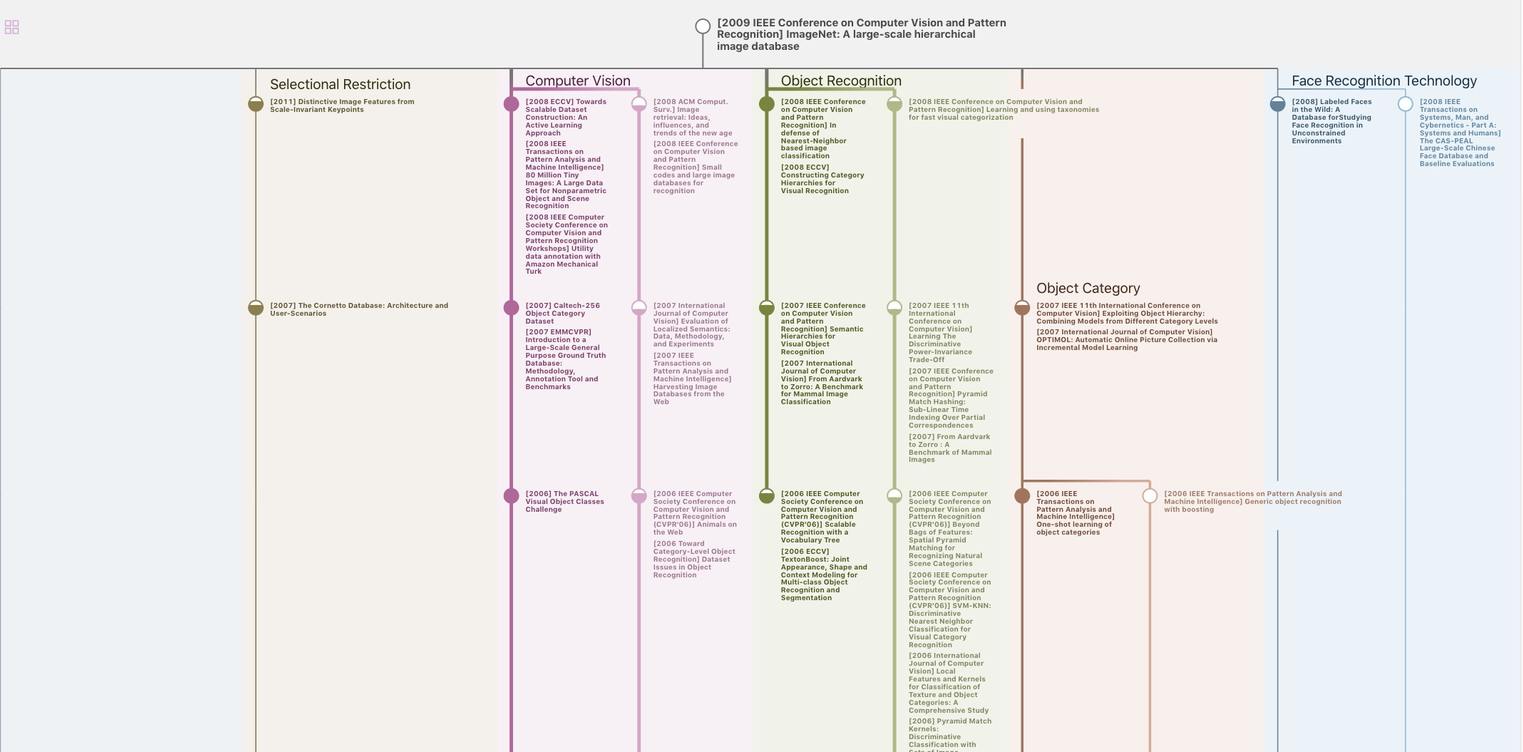
生成溯源树,研究论文发展脉络
Chat Paper
正在生成论文摘要