MAVISp: Multi-layered Assessment of VarIants by Structure for proteins
biorxiv(2022)
摘要
Cancer is a complex group of diseases due to the accumulation of mutations in tumor suppressors or oncogenes in the genome. Cancer alterations can be very heterogeneous, even in tumors from the same tissue, affecting the response to treatment and risks of relapse in different patients. The role of genomics variants on cancer predisposition, progression, and response to treatment continues to be realized. Thanks to advances in sequencing techniques and their introduction in a clinical setting, the number of genomic variants discovered is growing exponentially. Many of these variants are classified as Variants of Uncertain Significance (VUS), while other variants have been reported with conflicting evidence. Applications of bioinformatic-based approaches to characterize the effects of these variants demonstrated their full potential thanks to advances in machine learning, comparisons between predicted effects and cellular readouts, and advances in the field of structural biology and biomolecular simulations. We here introduce a modular structure-based framework for the annotations and classification of the impact of variants affecting the coding region of genes and impacting on the corresponding protein product (MAVISp, Multi-layered Assessment of VarIants by Structure for proteins) together with a Streamlit-based web application (https://github.com/ELELAB/MAVISp) where the variants and the data generated by the assessment are made available to the community for consultation or further studies. Currently, MAVISp includes information for ten different proteins and more than 4000 variants. New protein targets are routinely analyzed in batches through standardized Python-based workflows and high-throughput free energy and biomolecular simulations. We also illustrate the potential of the approach for each protein included in the database. New variants will be deposited on a regular base or in connection with future publications where the approach will be applied. Finally, we provide guidelines for new contributors who are interested in contributing to the collection in relation to their research.
### Competing Interest Statement
The authors have declared no competing interest.
更多查看译文
关键词
mavisp,proteins,variants,multi-layered
AI 理解论文
溯源树
样例
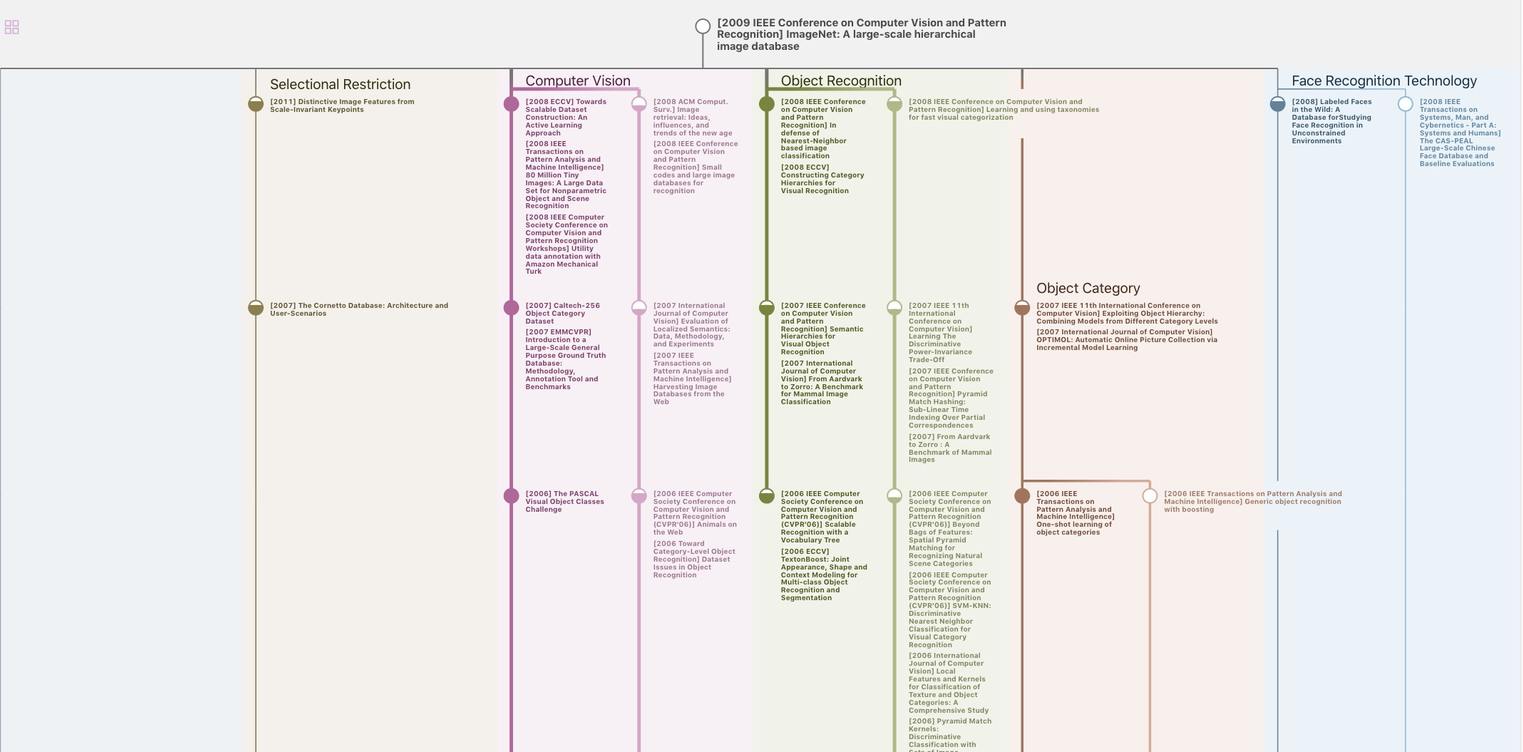
生成溯源树,研究论文发展脉络
Chat Paper
正在生成论文摘要