Unsupervised Term Extraction for Highly Technical Domains
arxiv(2022)
摘要
Term extraction is an information extraction task at the root of knowledge discovery platforms. Developing term extractors that are able to generalize across very diverse and potentially highly technical domains is challenging, as annotations for domains requiring in-depth expertise are scarce and expensive to obtain. In this paper, we describe the term extraction subsystem of a commercial knowledge discovery platform that targets highly technical fields such as pharma, medical, and material science. To be able to generalize across domains, we introduce a fully unsupervised annotator (UA). It extracts terms by combining novel morphological signals from sub-word tokenization with term-to-topic and intra-term similarity metrics, computed using general-domain pre-trained sentence-encoders. The annotator is used to implement a weakly-supervised setup, where transformer-models are fine-tuned (or pre-trained) over the training data generated by running the UA over large unlabeled corpora. Our experiments demonstrate that our setup can improve the predictive performance while decreasing the inference latency on both CPUs and GPUs. Our annotators provide a very competitive baseline for all the cases where annotations are not available.
更多查看译文
关键词
highly technical domains,extraction
AI 理解论文
溯源树
样例
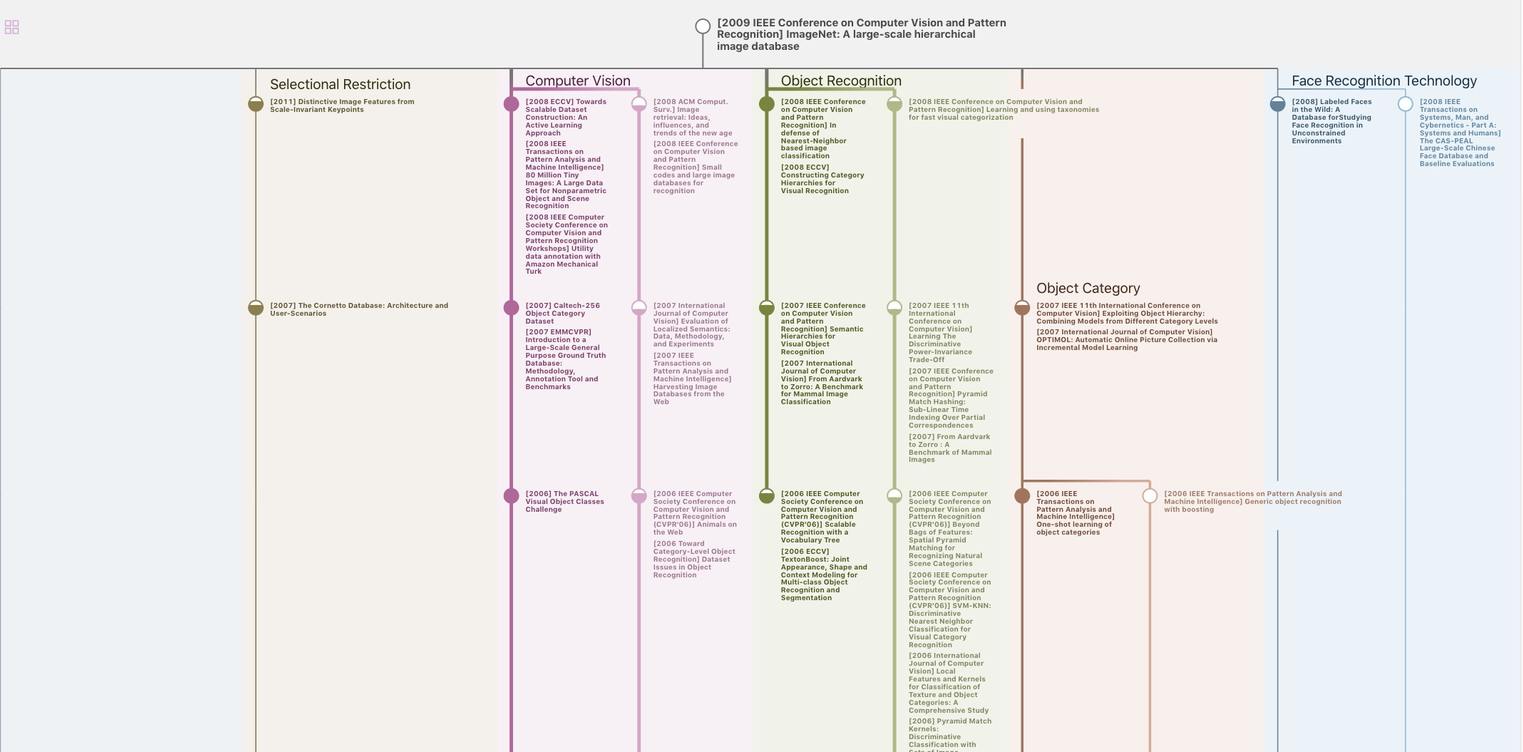
生成溯源树,研究论文发展脉络
Chat Paper
正在生成论文摘要