Generalised Likelihood Ratio Testing Adversaries through the Differential Privacy Lens
arxiv(2022)
摘要
Differential Privacy (DP) provides tight upper bounds on the capabilities of optimal adversaries, but such adversaries are rarely encountered in practice. Under the hypothesis testing/membership inference interpretation of DP, we examine the Gaussian mechanism and relax the usual assumption of a Neyman-Pearson-Optimal (NPO) adversary to a Generalized Likelihood Test (GLRT) adversary. This mild relaxation leads to improved privacy guarantees, which we express in the spirit of Gaussian DP and $(\varepsilon, \delta)$-DP, including composition and sub-sampling results. We evaluate our results numerically and find them to match the theoretical upper bounds.
更多查看译文
关键词
privacy,likelihood,testing
AI 理解论文
溯源树
样例
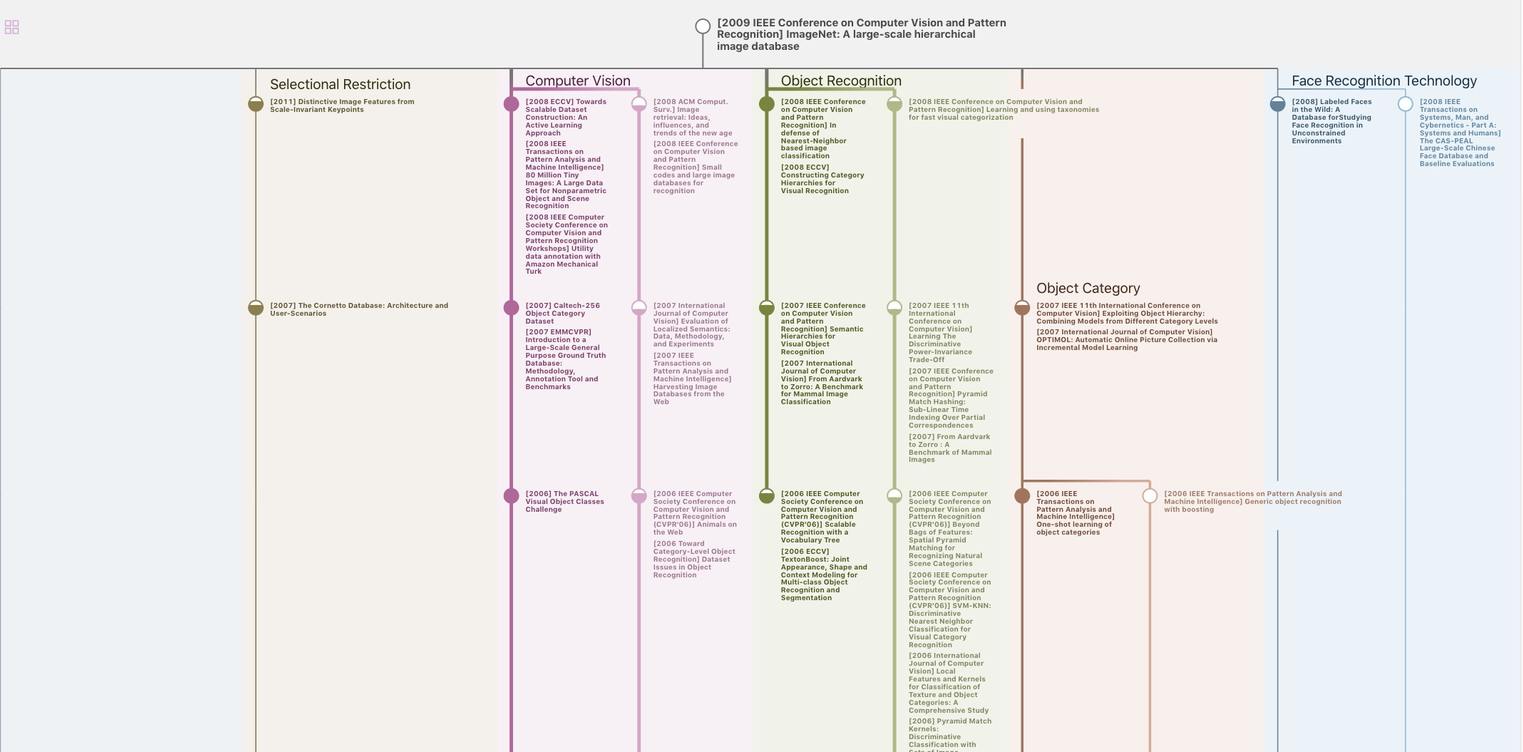
生成溯源树,研究论文发展脉络
Chat Paper
正在生成论文摘要