H-SAUR: Hypothesize, Simulate, Act, Update, and Repeat for Understanding Object Articulations from Interactions
arxiv(2022)
摘要
The world is filled with articulated objects that are difficult to determine how to use from vision alone, e.g., a door might open inwards or outwards. Humans handle these objects with strategic trial-and-error: first pushing a door then pulling if that doesn't work. We enable these capabilities in autonomous agents by proposing "Hypothesize, Simulate, Act, Update, and Repeat" (H-SAUR), a probabilistic generative framework that simultaneously generates a distribution of hypotheses about how objects articulate given input observations, captures certainty over hypotheses over time, and infer plausible actions for exploration and goal-conditioned manipulation. We compare our model with existing work in manipulating objects after a handful of exploration actions, on the PartNet-Mobility dataset. We further propose a novel PuzzleBoxes benchmark that contains locked boxes that require multiple steps to solve. We show that the proposed model significantly outperforms the current state-of-the-art articulated object manipulation framework, despite using zero training data. We further improve the test-time efficiency of H-SAUR by integrating a learned prior from learning-based vision models.
更多查看译文
关键词
object articulations,interactions,act,simulate,h-saur
AI 理解论文
溯源树
样例
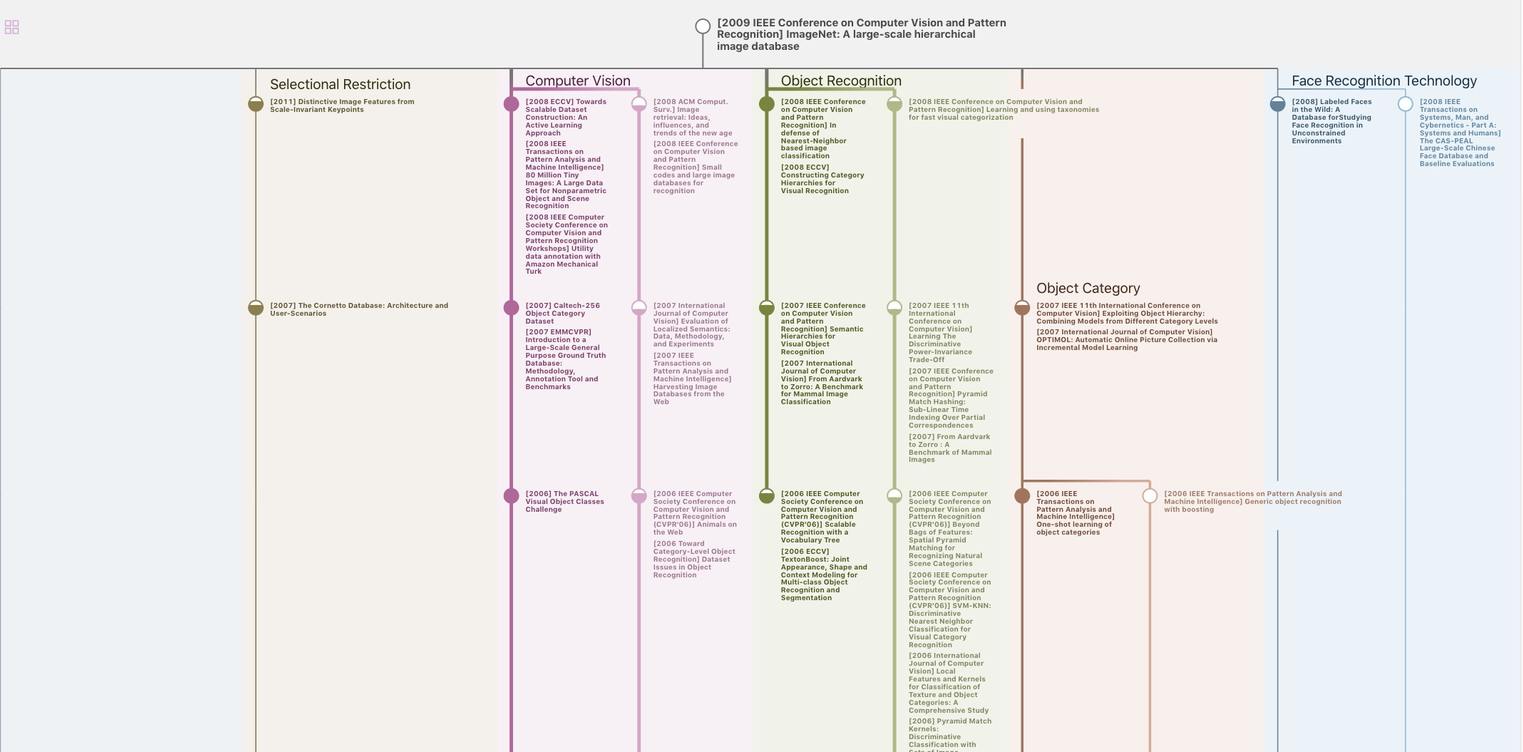
生成溯源树,研究论文发展脉络
Chat Paper
正在生成论文摘要