Bridging the Gap Between Target Networks and Functional Regularization
arxiv(2022)
摘要
Bootstrapping is behind much of the successes of Deep Reinforcement Learning. However, learning the value function via bootstrapping often leads to unstable training due to fast-changing target values. Target Networks are employed to stabilize training by using an additional set of lagging parameters to estimate the target values. Despite the popularity of Target Networks, their effect on the optimization is still misunderstood. In this work, we show that they act as an implicit regularizer. This regularizer has disadvantages such as being inflexible and non convex. To overcome these issues, we propose an explicit Functional Regularization that is a convex regularizer in function space and can easily be tuned. We analyze the convergence of our method theoretically and empirically demonstrate that replacing Target Networks with the more theoretically grounded Functional Regularization approach leads to better sample efficiency and performance improvements.
更多查看译文
关键词
target networks
AI 理解论文
溯源树
样例
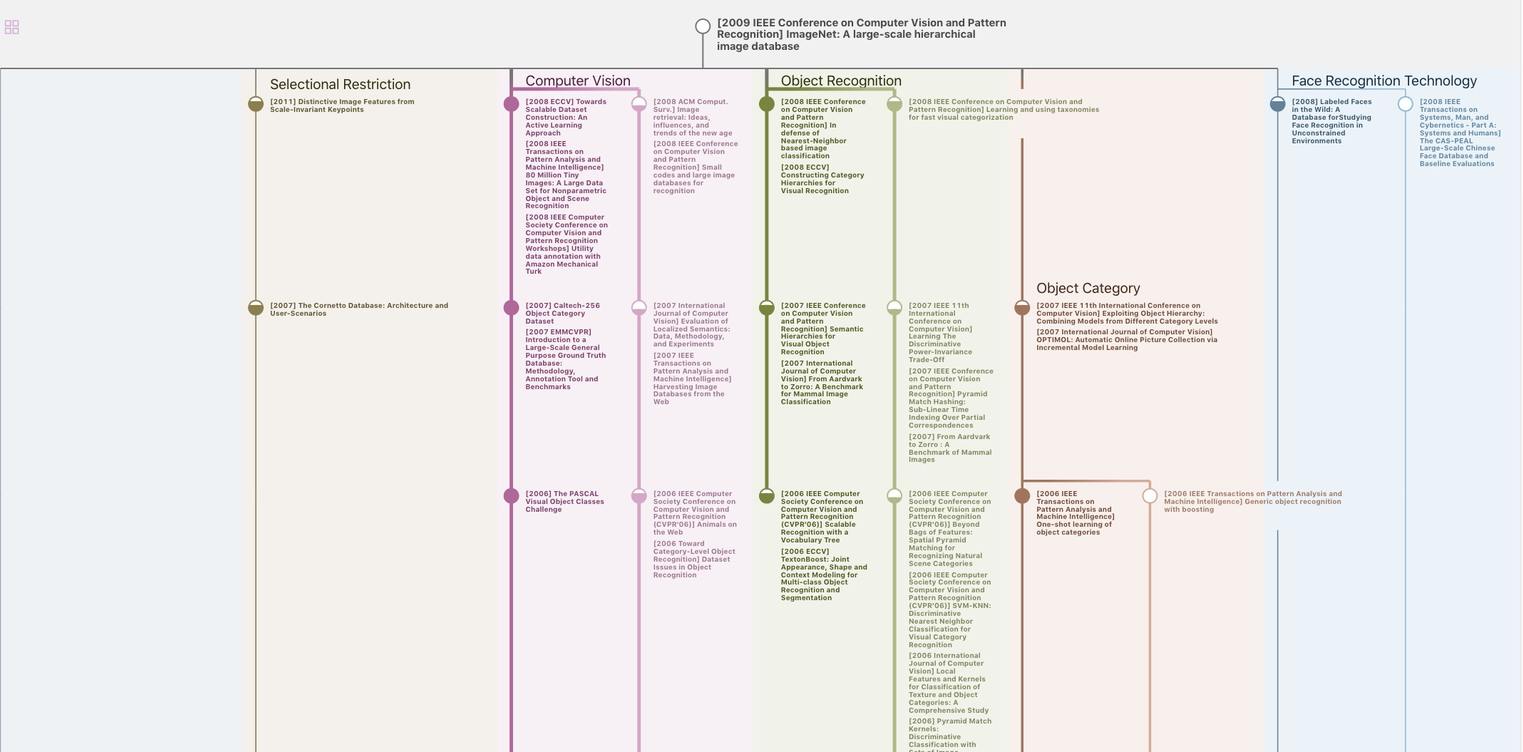
生成溯源树,研究论文发展脉络
Chat Paper
正在生成论文摘要