Automated hyperparameter optimization for simulating boundary conditions for acoustic and elastic wave propagation using deep learning
GEOPHYSICS(2023)
摘要
We have developed a deep learning framework to simulate the effect of boundary conditions for wave propagation in aniso-tropic media. To overcome the challenges associated with the stability of conventional implementation of boundary conditions for strongly anisotropic media, we develop an efficient algo-rithm using deep neural networks. We incorporate the hyper -parameter optimization (HPO) workflow in the deep learning framework to automate the network training process. Hyper -parameter selection is a crucial step in model building and has a direct impact on the performance of machine learning models. We implement three different HPO techniques, namely random search, Hyperband, and Bayesian optimization, for sim-ulating boundary conditions and compare the strengths and drawbacks of these techniques. We train the network using a few shot locations and time slices enabling the network to learn how to remove boundary reflections and simulate wave propa-gation for unbounded media. The automated deep learning framework with HPO improves the performance of deep learn-ing models by achieving the optimal minima and significantly improves the efficiency of the workflow. The benefit of this ap-proach is its simple implementation and significant reduction of reflections at the boundaries, especially in the case of tilted transverse isotropic media. We validate our approach by com-paring wave propagation at the boundaries using our algorithm with the output obtained using the unbounded media simulated by padding the model. Tests on different models with acoustic and elastic wave propagation verify the effectiveness of our ap-proach.
更多查看译文
关键词
hyperparameter optimization,elastic wave propagation,deep learning,boundary conditions
AI 理解论文
溯源树
样例
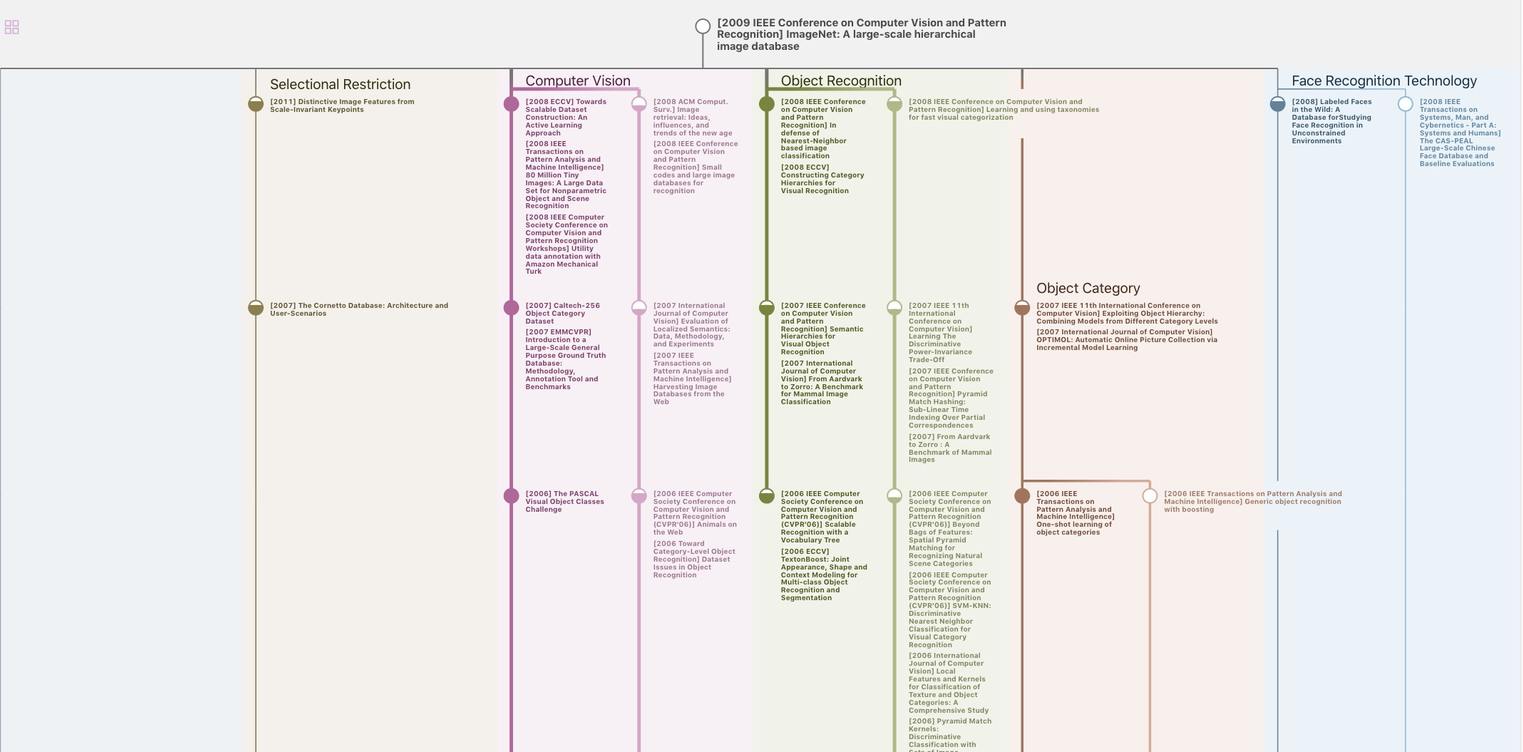
生成溯源树,研究论文发展脉络
Chat Paper
正在生成论文摘要