Edge-distributed Coordinated Hyper-Parameter Search for Energy Saving SON Use-Case
2022 IEEE INTERNATIONAL CONFERENCE ON COMMUNICATIONS WORKSHOPS (ICC WORKSHOPS)(2022)
摘要
Energy Efficient operation of ultra-dense heterogeneous network deployments is a big challenge for mobile networks. AI-assisted energy saving is one of the potential selforganizing network use cases for radio access network intelligence that can be used to predict the service load. This prediction can in turn be leveraged for proactively turning OFF/ON the capacity booster small cells within the coverage of always ON macro cells. These ML workloads can reside in macro cell base stations as opposed to conventional cloud-centric architecture to meet beyond 5G ambitious requirements of ultra-low latency, highest reliability, and scalability. However, the power-hungry hyperparameter search of ML workloads distributed at edges of the radio access network is a major challenge that can have substantial effect on the overall energy-efficiency of the network. In this paper, we illustrate how coordinated efficient training of distributed edge-ML models driven energy saving functions can enhance network energy efficiency. We validate the proposed method through a data-driven simulation methodology augmenting real traffic traces and comparing it with variants of legacy edge-ML hyper-parameter search techniques.
更多查看译文
关键词
Energy Saving, Hyper-Parameter Search, Edge, Machine learning, 6G, SON, Energy Efficiency.
AI 理解论文
溯源树
样例
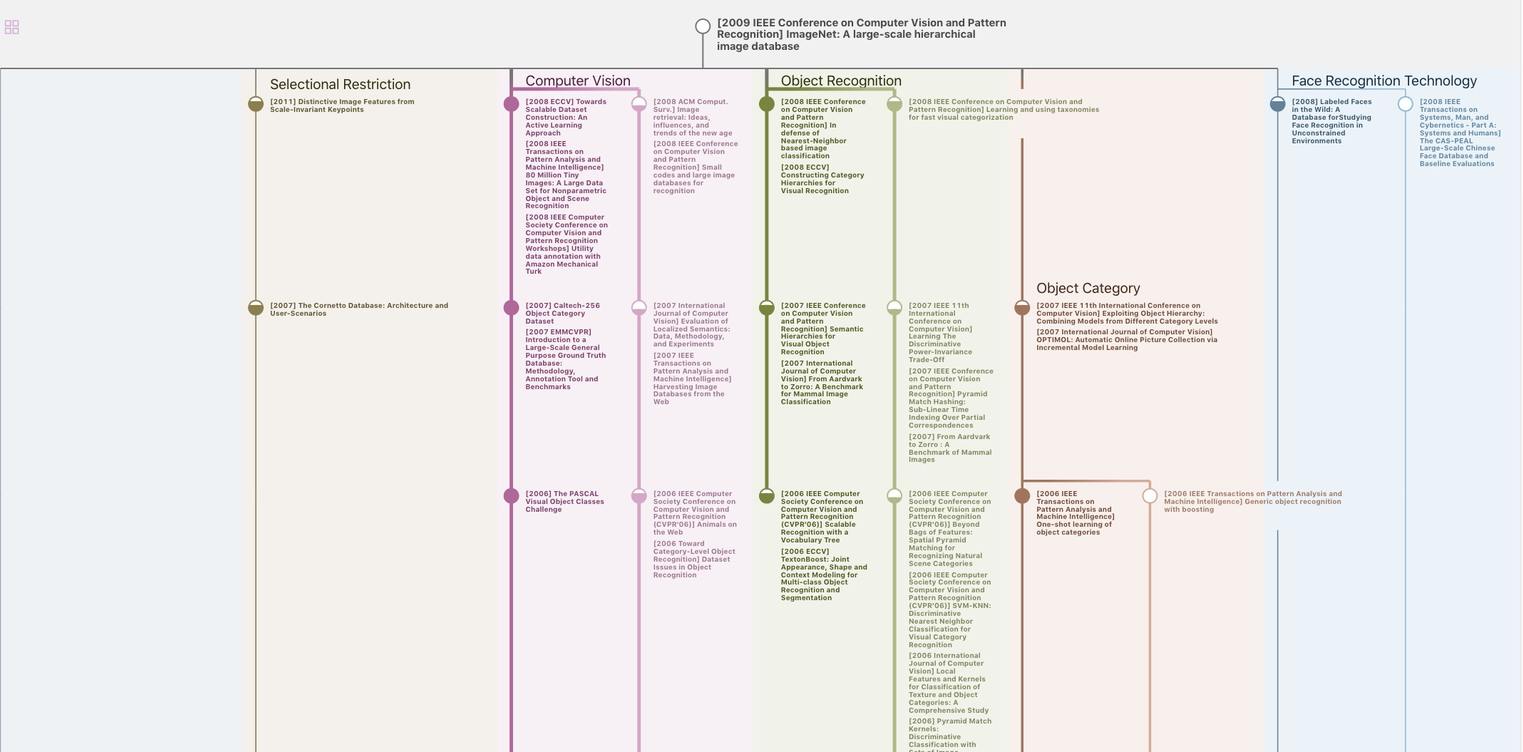
生成溯源树,研究论文发展脉络
Chat Paper
正在生成论文摘要