Short-term load forecasting based on empirical wavelet transform and random forest
ELECTRICAL ENGINEERING(2022)
摘要
Aiming at the problem of strong randomness and low forecasting accuracy in short-term electric load, a method based on empirical wavelet transform and random forest is proposed. In this method, the noise is extracted and stripped by wavelet transform, and the original data are decomposed into several groups of low or high frequencies. The empirical wavelet transform is more adaptive, and details are demonstrated accurately. These decomposed modes are used as characteristic variables to forecast with random forest. This method has three advantages: (1) Because of the instability of electric data, the empirical wavelet decomposition can be used to characterize the non-stationary signal characteristics. (2) Empirical wavelet transform has more advantages in time domain analysis because of its correlation to signal removal and the tendency of noise whitening. (3) More adaptive details with empirical mode (noise is no exception) are expressed in the random forest, and it still demonstrates accurate results even after data features are lost. The Australian electricity data in different periods are used for case analysis. The results compared with other methods have shown that the model could reveal effectively the influence of random noise and improve the accuracy and reliability of short-term load forecasting.
更多查看译文
关键词
Empirical wavelet transform (EWT), Random forest (RF), Short-term load forecasting
AI 理解论文
溯源树
样例
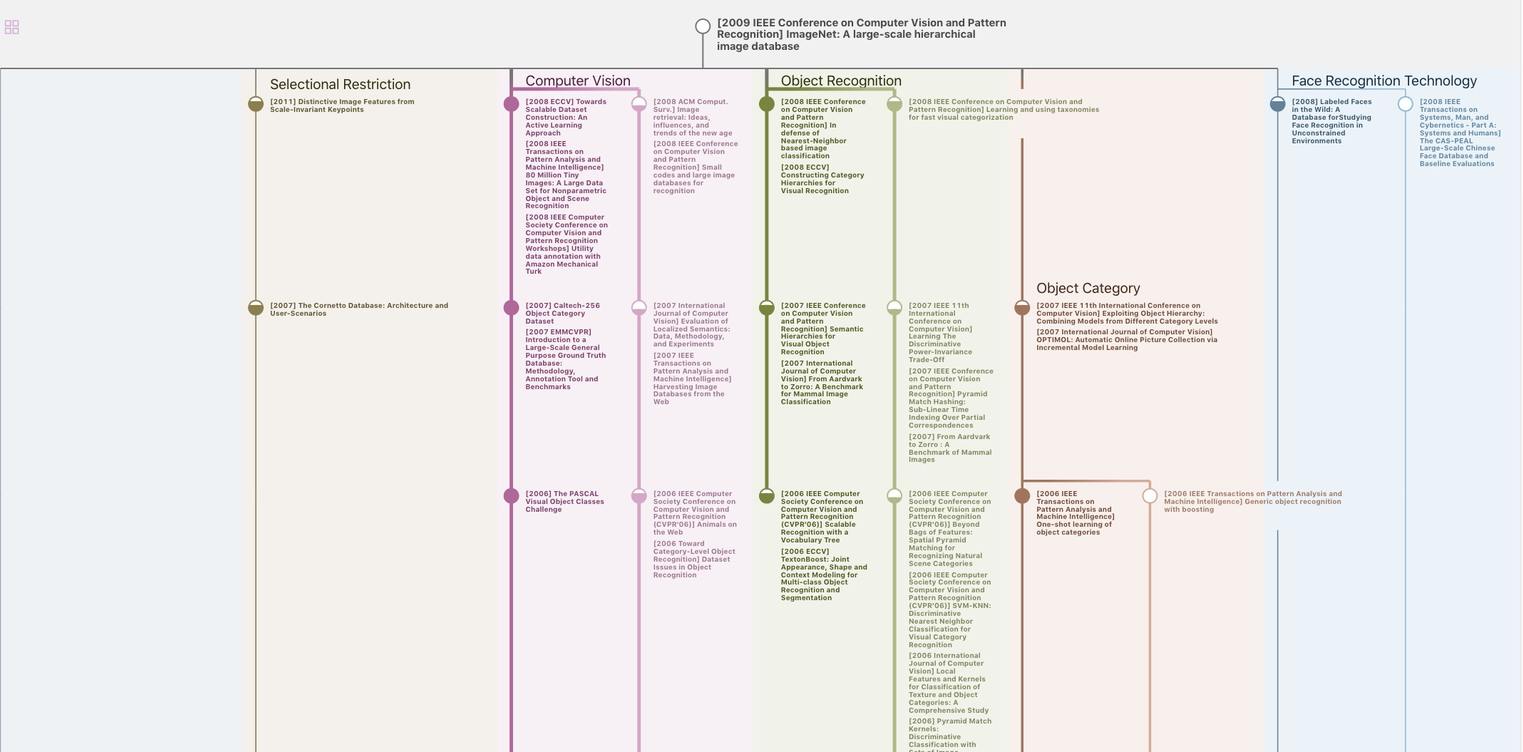
生成溯源树,研究论文发展脉络
Chat Paper
正在生成论文摘要