Sleep Behavior Detection Based on Pseudo-3D Convolutional Neural Network and Attention Mechanism
IEEE ACCESS(2022)
摘要
Good sleep quality is very important for everyone to protect physical and mental health. People's sleep behavior at night reflects their sleep status. In this paper, we propose a method to detect people's sleep behavior at night by adopting Pseudo-3D (P3D) convolution neural network with attention mechanism. In particular, we propose a new structure, which integrates Squeeze-and-Excitation (SE) blocks into P3D blocks, named P3D-Attention. For the input video, we use P3D blocks to extract spatial-temporal features, and use SE blocks to pay more attentions to the important channel features. The proposed network is tested on the Sleep Action (SA) dataset, which consists of five different actions, namely turn over, get up, fall off bed, play mobile phone, and normal sleep. Experimental results show that the proposed network achieves reasonably good detection results, and the accuracy rate on the test set can reach 90.67%. Compared with 3D convolutional neural networks (C3D), our proposed network can increase the accuracy by about 6% with only 1/6 model parameter size, and achieves an average prediction speed about 1.75 item/s. Compared with the residual spatiotemporal convolution network (R(2+1)D), our proposed network can increase the accuracy rate by about 1.5% with less than 1/2 model parameter size.
更多查看译文
关键词
Feature extraction, Behavioral sciences, Three-dimensional displays, Convolutional neural networks, Neural networks, Image coding, Sleep, Pseudo-3D convolution, attention mechanism, spatial-temporal feature extraction, sleep behavior detection
AI 理解论文
溯源树
样例
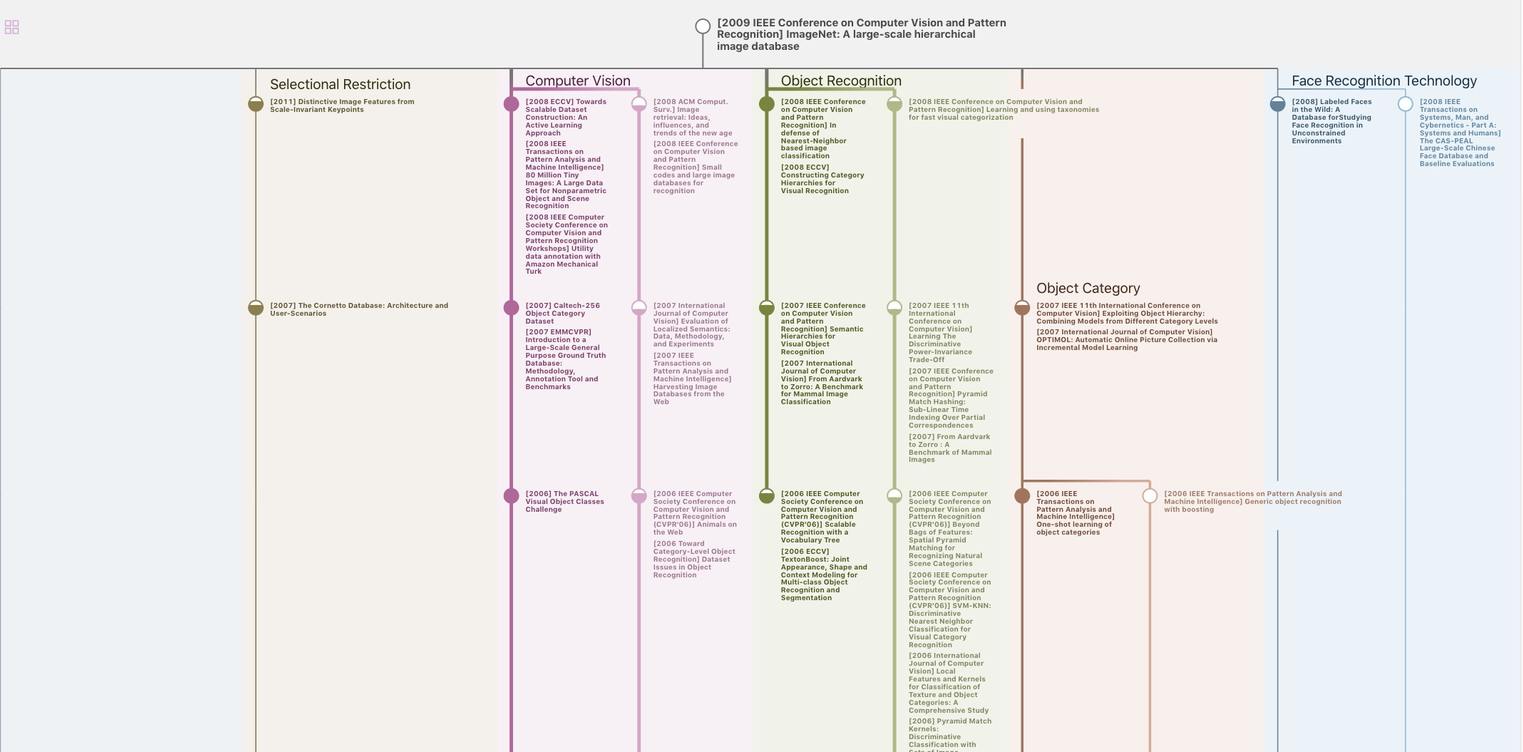
生成溯源树,研究论文发展脉络
Chat Paper
正在生成论文摘要