Continuous-time echo state networks for predicting power system dynamics
ELECTRIC POWER SYSTEMS RESEARCH(2022)
摘要
With the growing penetration of converter-interfaced generation in power systems, the dynamical behavior of these systems is rapidly evolving. One of the challenges with converter-interfaced generation is the increased number of equations, as well as the required numerical timestep, involved in simulating these systems. Within this work, we explore the use of continuous-time echo state networks as a means to cheaply, and accurately, predict the dynamic response of power systems subject to a disturbance for varying system parameters. We show an application for predicting frequency dynamics following a loss of generation for varying penetrations of grid-following and grid-forming converters. We demonstrate that, after training on 20 solutions of the fullorder system, we achieve a median nadir prediction error of 0.17 mHz with 95% of all nadir prediction errors within +/- 4 mHz. We conclude with some discussion on how this approach can be used for parameter sensitivity analysis and within optimization algorithms to rapidly predict the dynamical behavior of the system.
更多查看译文
关键词
Data-driven modeling techniques, Electro-magnetic transients, Machine learning, Power system dynamics
AI 理解论文
溯源树
样例
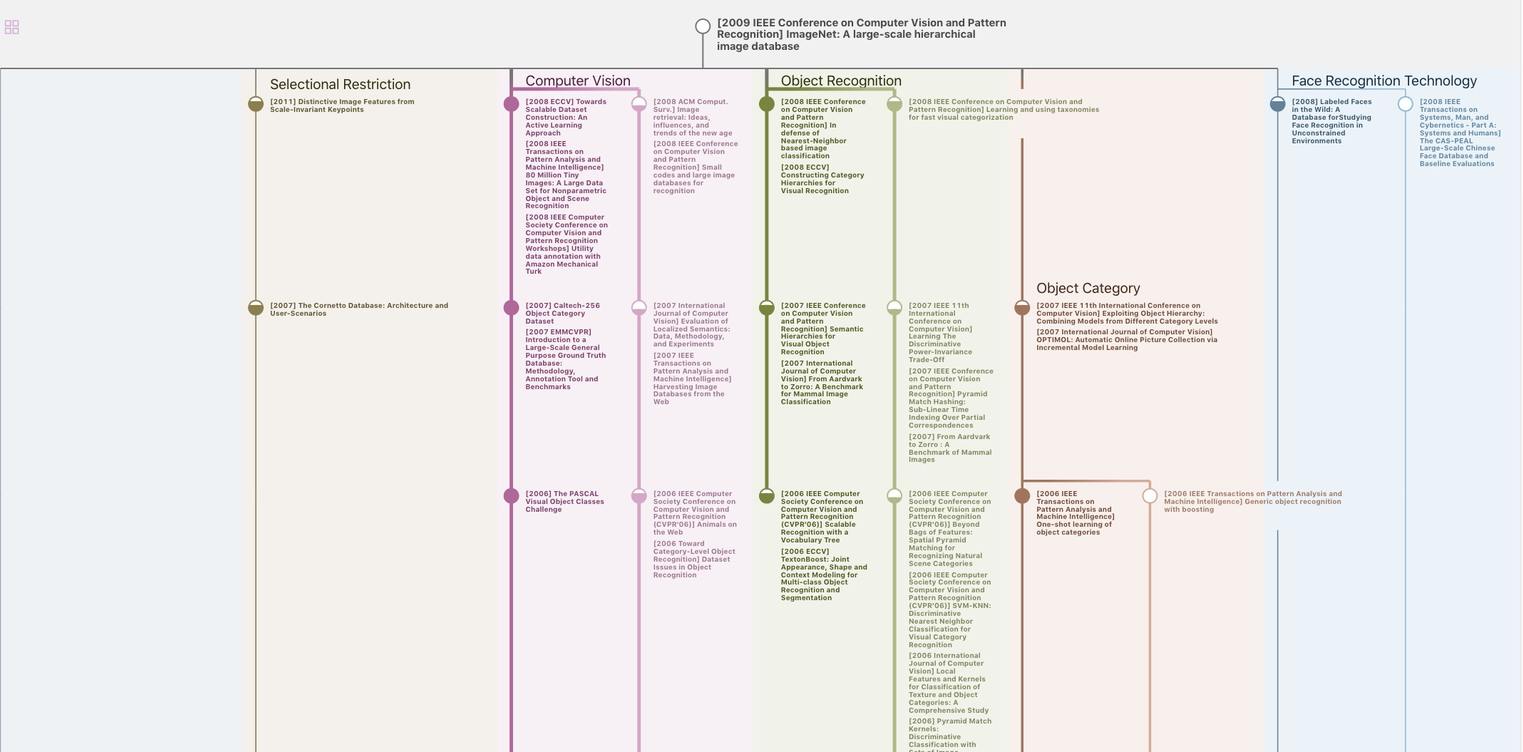
生成溯源树,研究论文发展脉络
Chat Paper
正在生成论文摘要