A Deep-Learning Based Method for Analysis of Students' Attention in Offline Class
ELECTRONICS(2022)
摘要
Students' actual learning engagement in class, which we call learning attention, is a major indicator used to measure learning outcomes. Obtaining and analyzing students' attention accurately in offline classes is important empirical research that can improve teachers' teaching methods. This paper proposes a method to obtain and measure students' attention in class by applying a variety of deep-learning models and initiatively divides a whole class into a series of time durations, which are categorized into four states: lecturing, interaction, practice, and transcription. After video and audio information is taken with Internet of Things (IoT) technology in class, Retinaface and the Vision Transformer (ViT) model is used to detect faces and extract students' head-pose parameters. Automatic speech recognition (ASR) models are used to divide a class into a series of four states. Combining the class-state sequence and each student's head-pose parameters, the learning attention of each student can be accurately calculated. Finally, individual and statistical learning attention analyses are conducted that can help teachers to improve their teaching methods. This method shows potential application value and can be deployed in schools and applied in different smart education programs.
更多查看译文
关键词
smart education, smart class, learning attention, deep learning, head-pose estimation
AI 理解论文
溯源树
样例
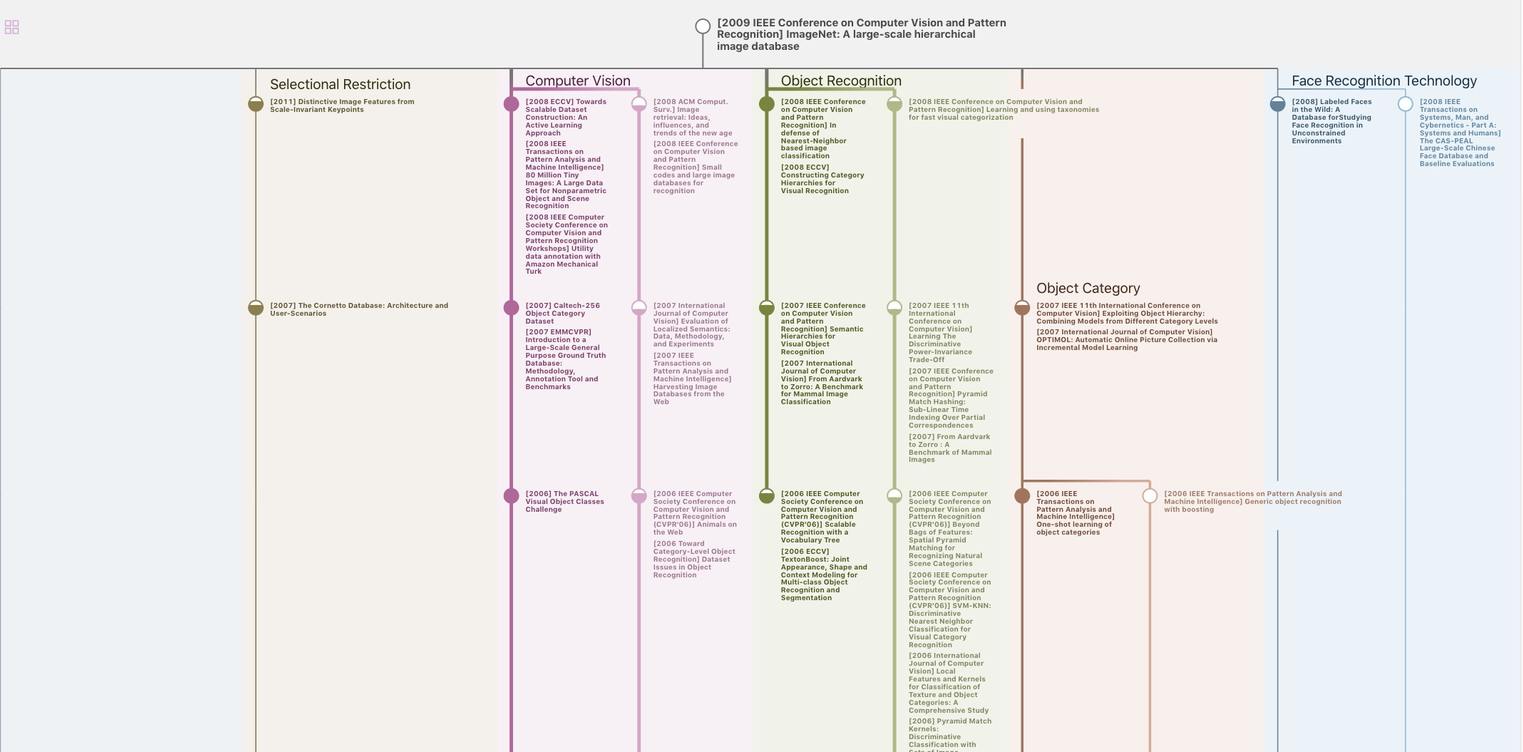
生成溯源树,研究论文发展脉络
Chat Paper
正在生成论文摘要