Online state of health estimation for lithium-ion batteries based on a dual self-attention multivariate time series prediction network
ENERGY REPORTS(2022)
摘要
With the development of cloud and edge computing, deep learning based on big data has been widely utilized for lithium-ion battery state of health (SoH) online estimation, where improving the accuracy, robustness, and real-time applicability are current research challenges. Focusing on these points, this paper proposes a novel health feature analysis and screening method and a dual self-attention multivariate time series estimation network (DSMTNet). First, the correlation between all feature sequences and the SoH is evaluated by the Pearson correlation coefficient method. On this basis, the 15 most relevant features are selected by the light gradient boosting machine method as the DSMTNet input. Next, multi-head convolutional neural networks are utilized for encoding the battery features to enhance the final representation learning results. Then, a global attention unit is utilized to model the weights of the encoded feature sequences to extract common information, and a local attention unit is chosen to obtain the differentiated information, which is used as supplementary information. Finally, the accuracy, robustness, and computing time of the DSMTNet method are verified on experimental data. The results prove the superiority of the proposed method compared with other implemented approaches.(C) 2022 The Author(s). Published by Elsevier Ltd.
更多查看译文
关键词
Lithium-ion battery, State of health, Feature screening, Dual attention mechanism
AI 理解论文
溯源树
样例
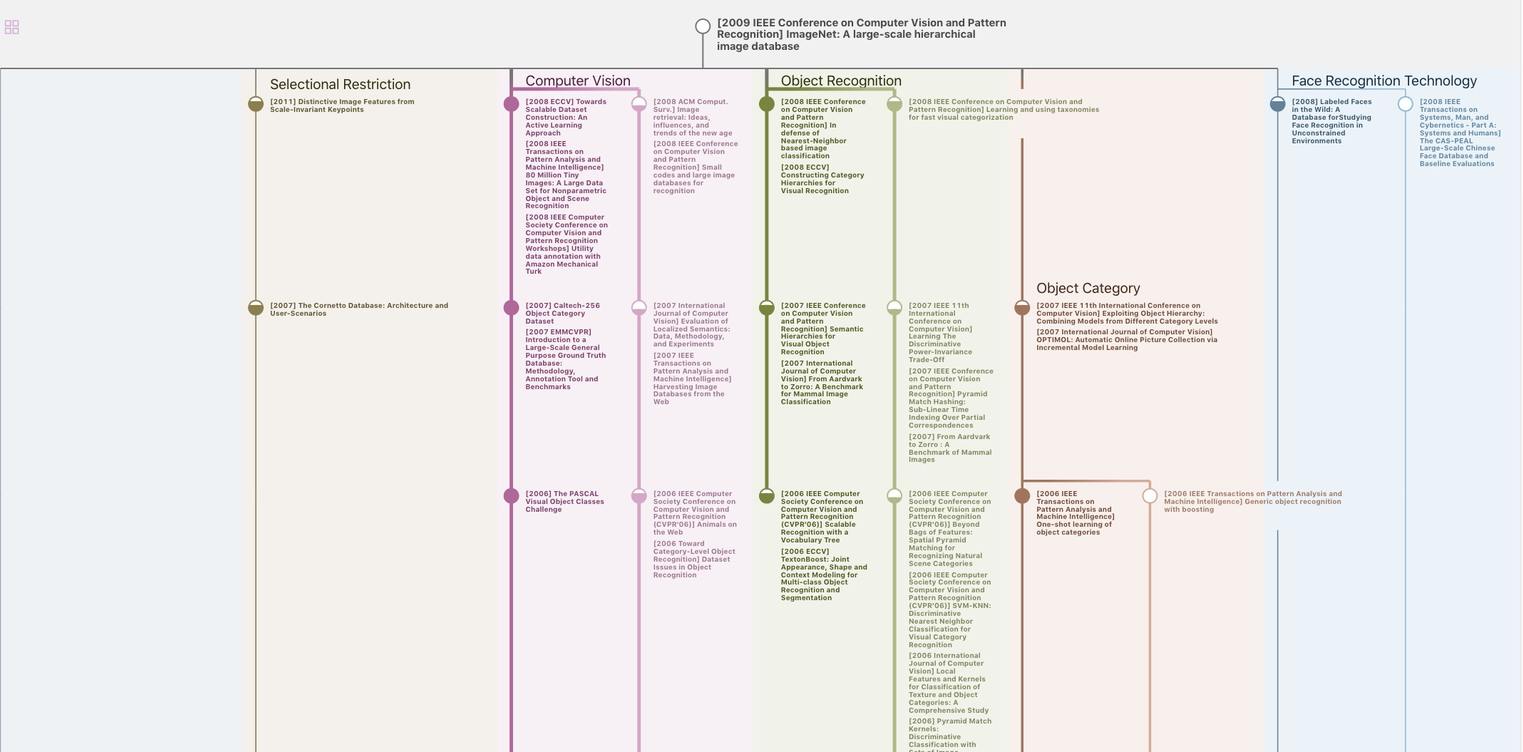
生成溯源树,研究论文发展脉络
Chat Paper
正在生成论文摘要