Short-Term Interval Prediction of Wind Power Based on KELM and a Universal Tabu Search Algorithm
SUSTAINABILITY(2022)
摘要
Installed wind power has significantly grown in recent years to synchronize with the ever-increasing demand for environment-friendly and renewable energy. However, wind energy has significant uncertainty or random futures, and will give rise to destructive effects on the safety operations of the power system. In this respect, an accurate and reliable wind power prediction is of great significance for improving the power system stability and optimizing the dispatch plan. Compared with traditionally deterministic point forecast techniques, probabilistic forecasting approaches can provide more stochastic information to quantify the random characteristics of wind power and to estimate its impacts on the power system. Moreover, the interval of the output power is a key stochastic information on wind power. In general, an interval prediction needs to compromise the calibration and the average width of the predicted interval. To find the best combination of these two metrics, a methodology based on a kernel extreme learning machine (KELM) and an improved universal tabu search algorithm is proposed. In the proposed methodology, to eliminate the inherent randomness on the weights between the input and hidden lays in the commonly used extreme learning machine, a radial-basis-function-based kernel extreme learning machine is proposed, and an improved tabu search method is introduced to optically compromise the calibration and the average width of the predicted interval to overcome the deficiency of existing algorithms, such as the insufficient global search ability of a particle swarm optimization. A prototype wind farm is utilized as a case study to verify the efficiency and advantage of the proposed methodology.
更多查看译文
关键词
global optimization, interval prediction, kernel extreme learning machine, tabu search algorithm, wind power
AI 理解论文
溯源树
样例
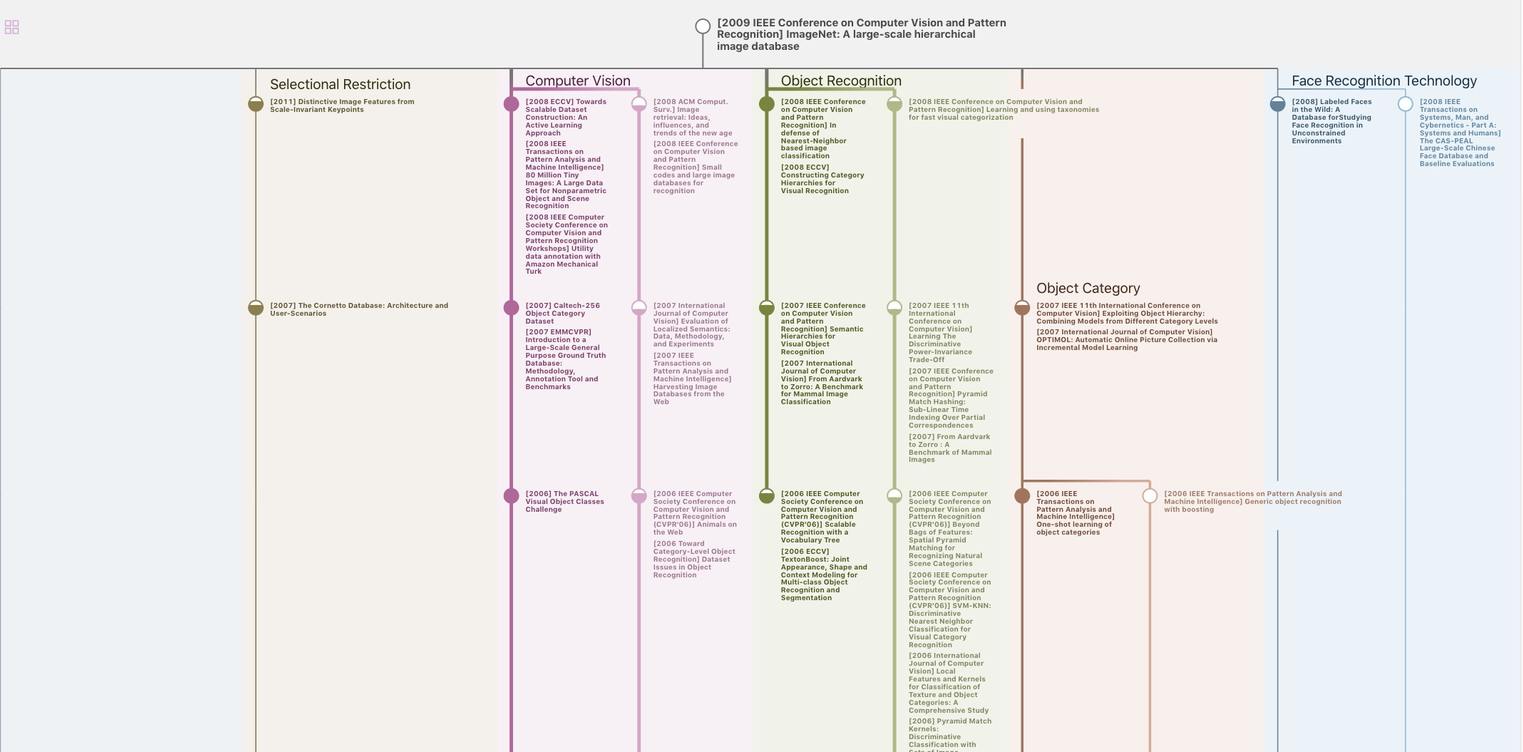
生成溯源树,研究论文发展脉络
Chat Paper
正在生成论文摘要