Automatic Homogenization of Time Series: How to Use Metadata?
ATMOSPHERE(2022)
摘要
Long time series of observed climate data are often affected by changes in the technical conditions of the observations, which cause non-climatic biases, so-called inhomogeneities. Such inhomogeneities can be removed, at least partly, by the spatial comparison and statistical analysis of the data, and by the use of documented information about the historical changes in technical conditions, so-called metadata. Large datasets need the use of automatic or semiautomatic homogenization methods, but the effective use of non-quantitative metadata information within automatic procedures is not straightforward. The traditional approach suggests that a piece of metadata can be considered in statistical homogenizations only when the statistical analysis indicates a higher than threshold probability of inhomogeneity occurrence at or around the date of the metadata information. In this study, a new approach is presented, which suggests that the final inhomogeneity corrections should be done by the ANOVA correction model, and all the metadata dates likely indicating inhomogeneities according to the content of the metadata should be included in that correction step. A large synthetic temperature benchmark dataset has been created and used to test the performance of the ACMANT homogenization method both with traditional metadata use and with the suggested new method. The results show that while the traditional metadata use provides only 1-4% error reduction in comparison with the residual errors obtained by the homogenization without metadata, this ratio reaches 8-15% in the new, permissive use of metadata. The usefulness of metadata depends on the test dataset properties and homogenization method, these aspects are examined and discussed.
更多查看译文
关键词
time series, homogenization, metadata, benchmarking, ACMANT, ANOVA correction method, climate change detection
AI 理解论文
溯源树
样例
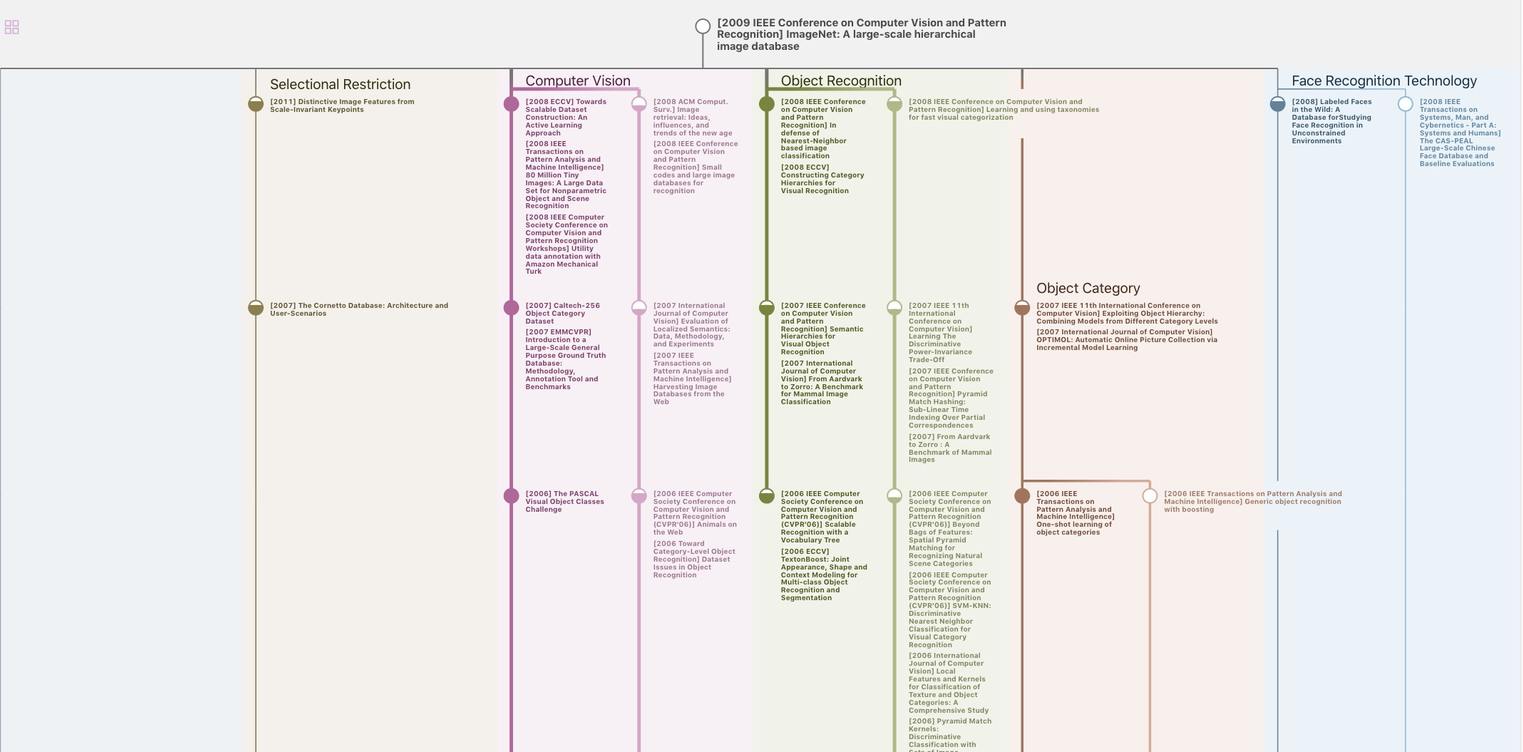
生成溯源树,研究论文发展脉络
Chat Paper
正在生成论文摘要