Comparison of Two Microcontroller Boards for On-Device Model Training in a Keyword Spotting Task
2022 11th Mediterranean Conference on Embedded Computing (MECO)(2022)
摘要
Machine learning applications on resource-constrained devices such as microcontroller units often use models trained externally on more powerful devices. This approach, however, limits a later adaptation of the machine learning model in the device to changing data. Differently, on-device training allows the model to be updated for new datasets, but the training process needs to take into account the resource limitations of the device. This paper compares on-device training performance for a keyword spotting task using two popular microcontroller boards, Arduino Nano 33 BLE Sense and Arduino Portenta H7, in terms of inference accuracy, training latency, and current consumption. We use feedforward neural networks having a single hidden layer for models. The inference accuracy has been significantly improved using the Portenta H7 board by employing more neurons fitted to its memory budget, compared to the Nano board. With a neural network having 25 neurons for a hidden layer, the 5.0 x inference and 4.2 x training speedups are achieved using the Arduino Portenta H7 board, compared to the Arduino Nano 33 BLE Sense. While the memory of the Arduino Nano 33 BLE Sense is capable to train a neural network for the keyword spotting task, the Arduino Portenta H7 gives new possibilities for exploring more complex models for more complex problems thanks to a larger memory budget and adapting a model to new data in lower latency.
更多查看译文
关键词
TinyML,machine learning,IoT
AI 理解论文
溯源树
样例
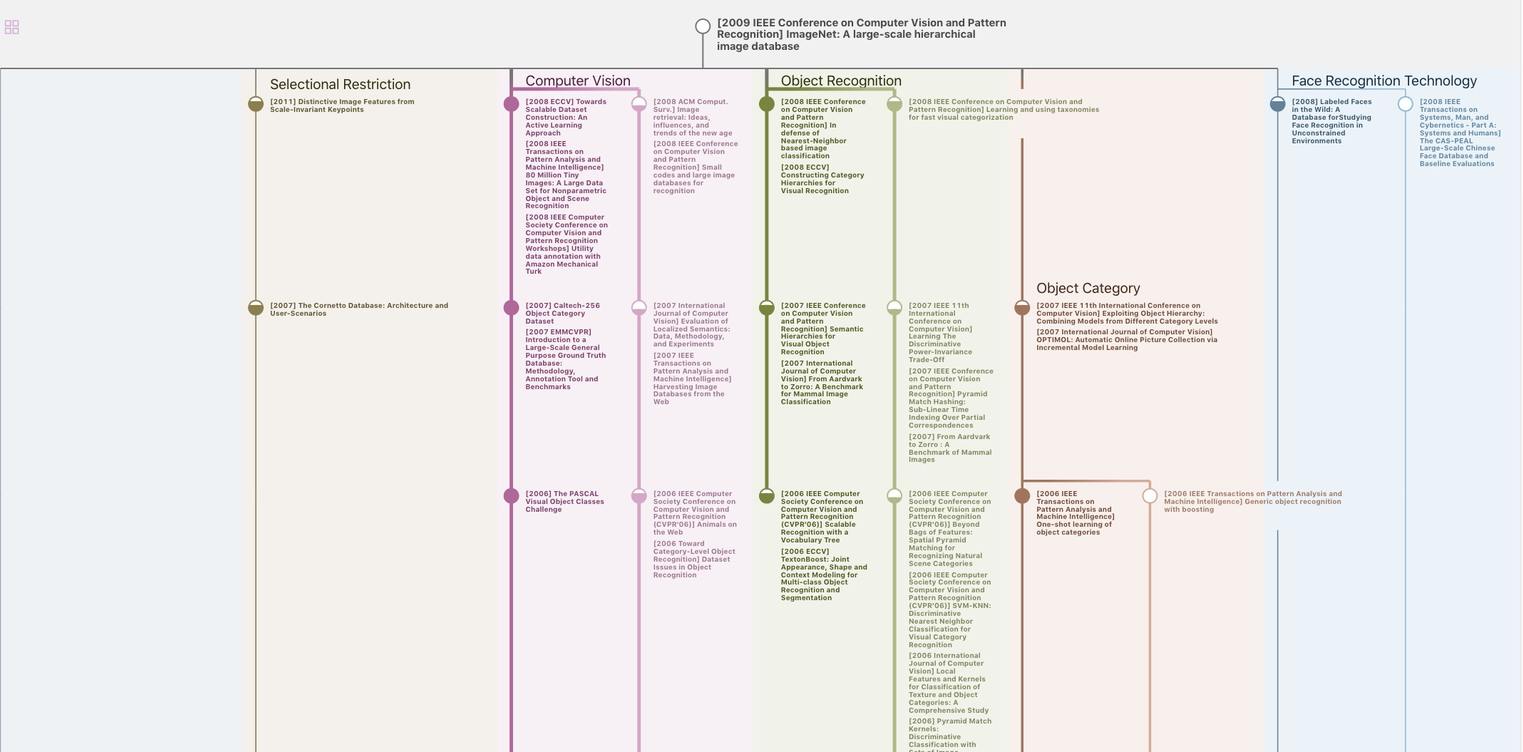
生成溯源树,研究论文发展脉络
Chat Paper
正在生成论文摘要