A Self-Fusion Network Based on Contrastive Learning for Group Emotion Recognition
IEEE TRANSACTIONS ON COMPUTATIONAL SOCIAL SYSTEMS(2023)
摘要
Group emotion recognition (GER) from image has attracted much attention in recent years. Networks using attention mechanism for GER have shown great potential. However, the performance of the current attention-based GER networks suffers from the indistinctive features of individuals in the group, poor feature fusion weights, and the lack of semantic information of the objects in the image. We present a new framework that is composed of three networks, FacesNet, SceneNet, and ObjectsNet, to address these shortcomings. This new framework is designed to recognize group emotion by exploiting the information from the faces, scene, and objects in image. In FacesNet, we use contrastive learning to help the network extract distinctive emotion features and a new attention mechanism named self-fusion module to generate precise fusion weights for aggregation of individual facial features. We design SceneNet to capture the multiscale scene features to exploit the emotion cues from the scene. We construct a fully connected network named ObjectsNet to classify the semantic features of the objects. Finally, we linearly integrate the outputs of these three networks as the final output of this unique framework for GER. Experiment results on three datasets for GER show that our proposed framework achieved better performance in terms of recognition accuracy compared with the state-of-the-art methods.
更多查看译文
关键词
Feature extraction, Emotion recognition, Image recognition, Semantics, Facial features, Faces, Face recognition, Attention mechanism, contrastive learning, group emotion, recognition
AI 理解论文
溯源树
样例
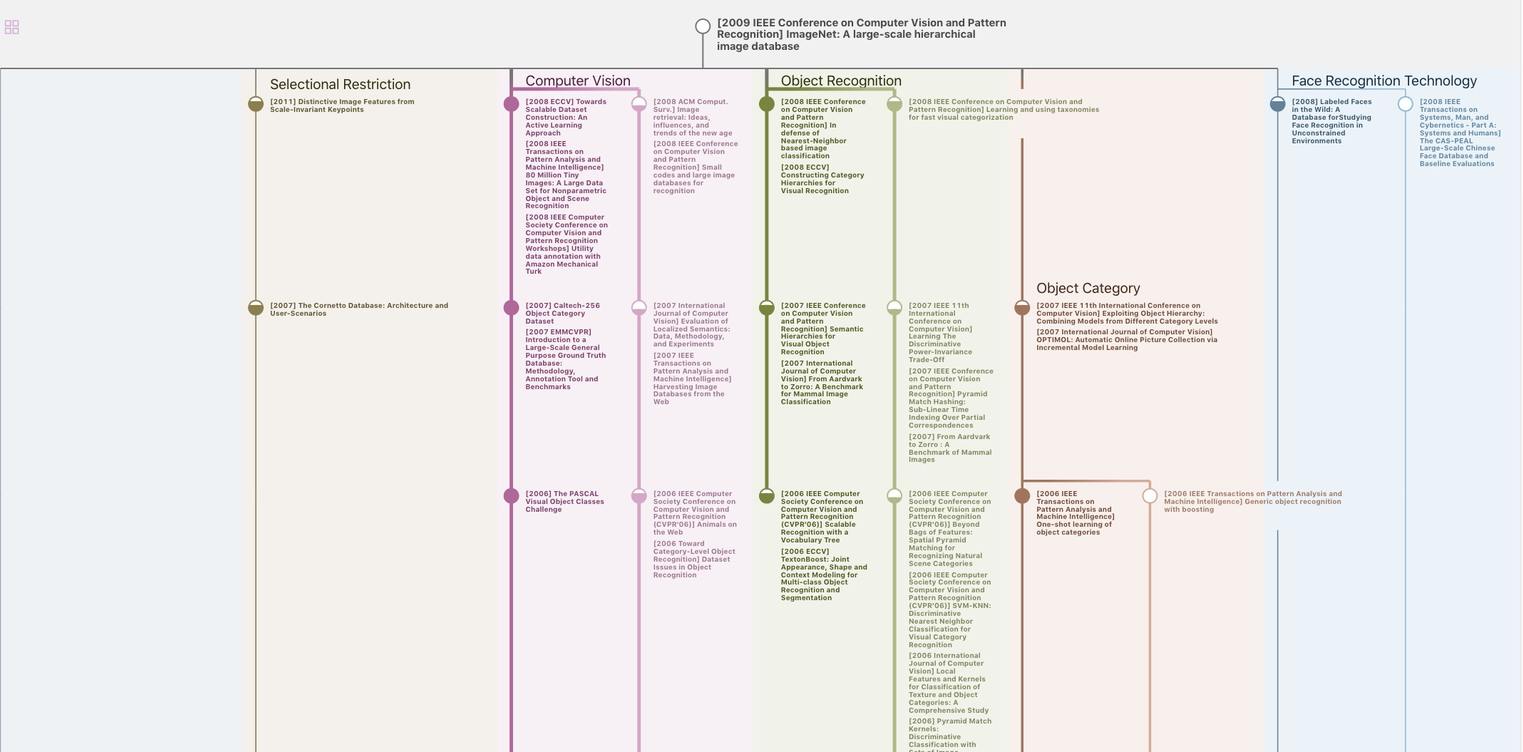
生成溯源树,研究论文发展脉络
Chat Paper
正在生成论文摘要