An adversarial transferability metric based on SVD of Jacobians to disentangle the correlation with robustness
Applied Intelligence(2022)
摘要
Transferability of adversarial samples under different convolutional neural network (CNN) models is one of the metrics indicators to assess the efficiency of adversarial examples and an important research direction in defense of that. Transferability isolate models employ a particular alternative model to avoid black-box attacks. Meanwhile, recent research has revealed that adversarial transferability across sub-models may be utilized to express the diversity requirements of sub-models under ensemble robustness abstractly. Due to the lack of mathematical description for this adversarial transferability, it was utilized to be abstractly described as the diversity of different hypotheses. This paper employs the Jacobians matrix’s singular value decomposition (SVD) to provide a more accurate mathematical description of the transferability of adversarial samples between models and proposes a corresponding evaluation metric. Based on this metric, a new regularization constraint is introduced into the ensemble training, and the adversarial transferability between the sub-models is isolated optimally without the prior information of the adversarial samples. Based on the proposed metric accurately defining the transferability, further ensemble robustness experiments under small-scale dataset disentangle the correlation between transferability and robustness, indicating that the transferability isolation can only achieve robustness under an alternative transfer-based attack with partial sub-models of the ensemble.
更多查看译文
关键词
Adversarial transferability metric, Jacobians matrix, Singular value decomposition, Ensemble robustness
AI 理解论文
溯源树
样例
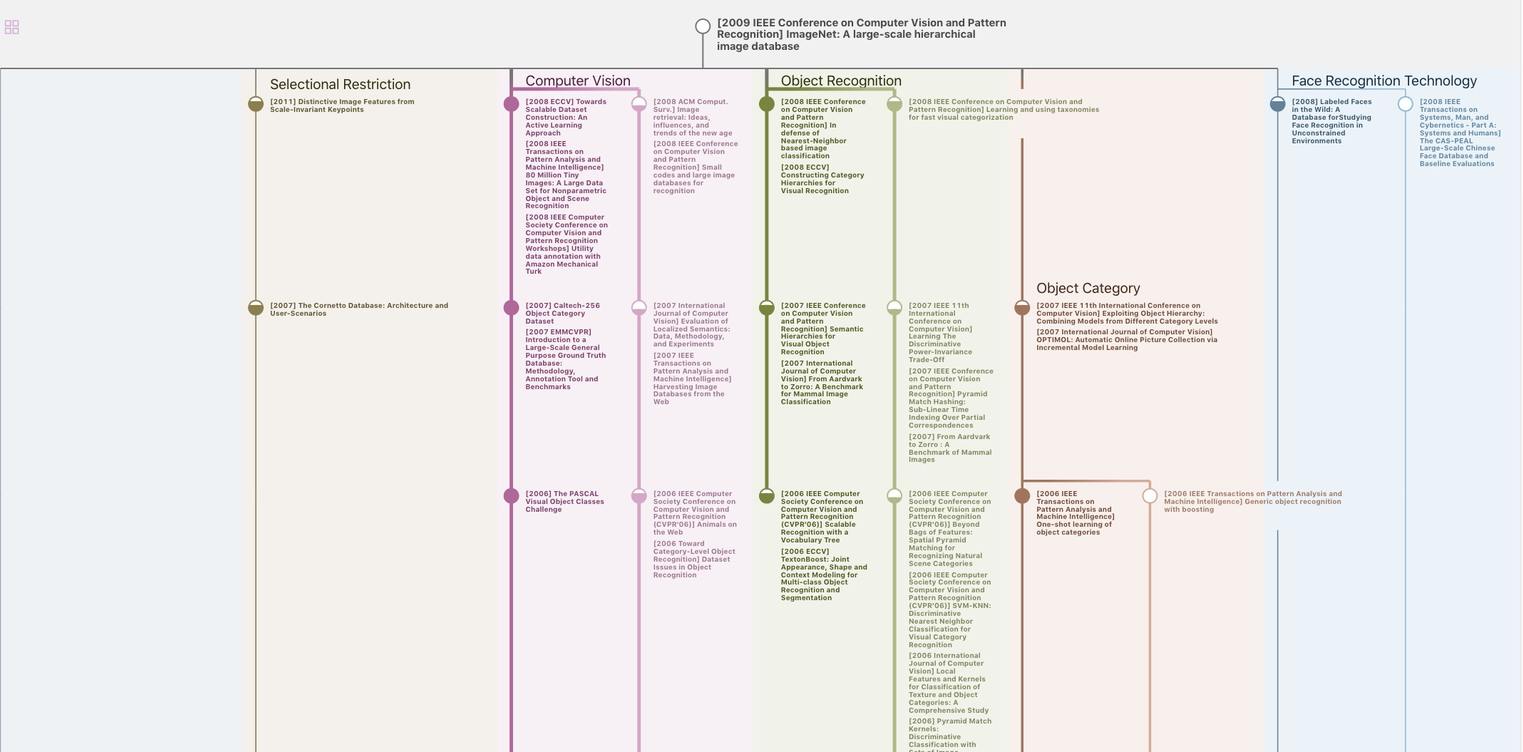
生成溯源树,研究论文发展脉络
Chat Paper
正在生成论文摘要