Fast and effective pedestrian detection based on low-level visual features combination
JOURNAL OF ELECTRONIC IMAGING(2022)
摘要
Automatic pedestrian detection is a crucial task for intelligent vehicles as it helps avoid car-to-pedestrian accidents. Recently, several approaches have been proposed to improve detection accuracy, such as the channel-based family, which is based on the aggregation of channel features (e.g., HOG + LUV) to represent the shape of pedestrians. Previous handcrafted-based detectors significantly improved the detection performance by applying different filters to channel features. However, when there are similar-looking background objects, their detection performance suffers due to the lack of texture features. Another drawback of these approaches lies in their high computational cost, limiting their use for real-world applications, especially for resource constrained systems. To mitigate these drawbacks while maintaining a distinguishable detection accuracy, we propose a method that combines a simple but effective texture descriptor based on local binary patterns with HOG + LUV. The concatenated features are used to train a boosted decision trees classifier. To evaluate our approach, we carried out extensive experiments on four well-known datasets, including INRIA, ETH, KITTI, and Caltech. Experimental results show that the proposed method achieves a competitive performance to the channel-based detectors with significantly lower computational cost; it runs at 91.64 and 6.08 times faster than the closest competitors detectors on the INRIA and Caltech datasets, respectively. Furthermore, it outperforms these methods when evaluating under the corrected annotations of the Caltech dataset.
更多查看译文
关键词
pedestrian detection, channel features, local binary pattern, intelligent vehicles
AI 理解论文
溯源树
样例
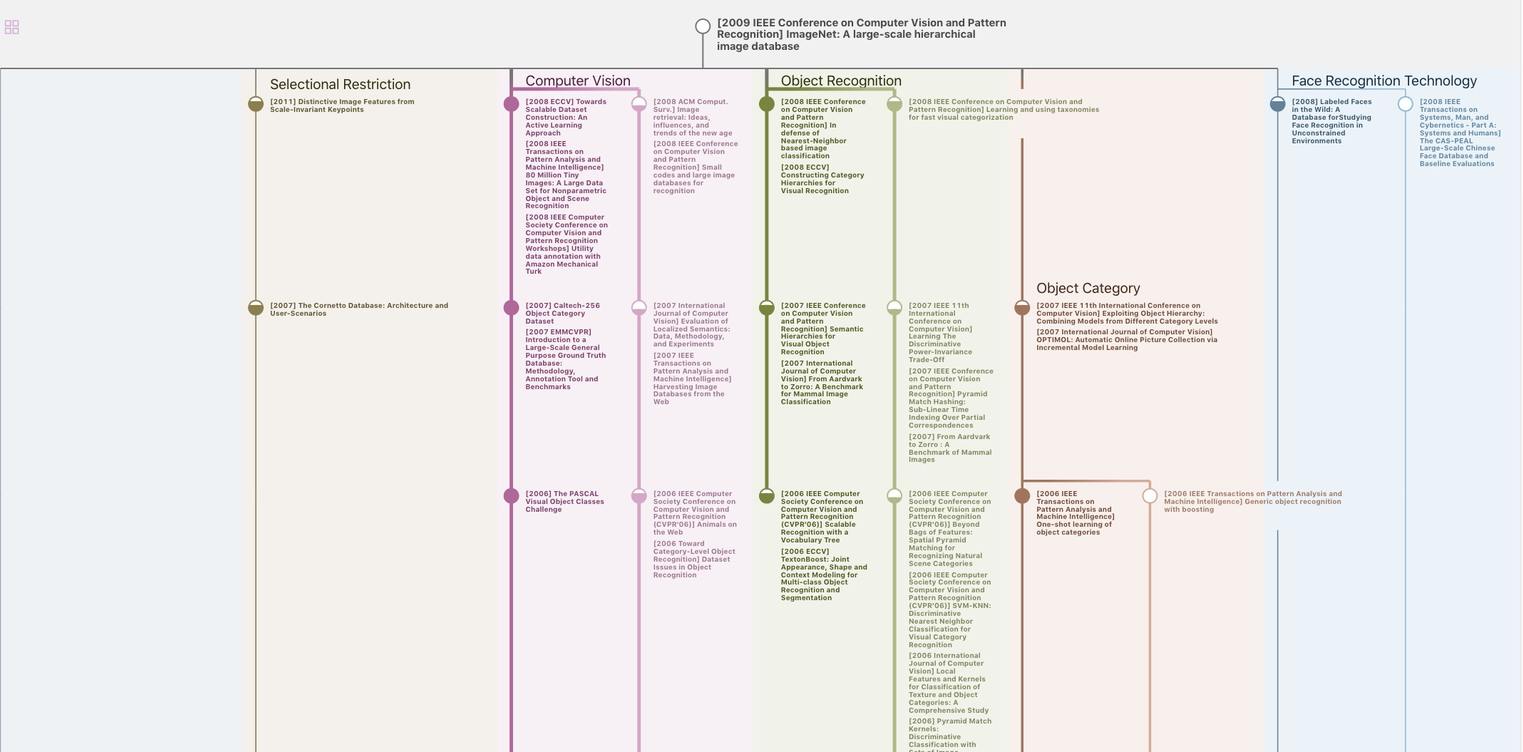
生成溯源树,研究论文发展脉络
Chat Paper
正在生成论文摘要