Goal Programming in Federated Learning: an Application to Time Series Forecasting
2022 INTERNATIONAL CONFERENCE ON DECISION AID SCIENCES AND APPLICATIONS (DASA)(2022)
摘要
As data becomes more prevalent in our societies, the need for large-scale data analysis is expanding at an exponential rate. The advantage of having a plethora of data is that it allows the decision-maker to adopt complicated models in settings that were previously too expensive. A method based on distributed learning is also required because of the sheer volume of data. As a result, Deep Learning models demand a significant amount of resources, and distributed training is required. A technique to distributed learning based on many criteria is presented in this research. An ensemble of decision rules that maximize aprioristically stated performance measures is built using the Weighted Goal Programming technique in its Chebyshev formulation, which is a variation of the Weighted Goal Programming approach. Such a formulation is advantageous since it is model and metric agnostic and produces an output that is easy to understand for the decision-maker to comprehend. We put our technique to the test by demonstrating a real application in the field of power demand forecasting. In our experiments, we found that when we allow for overlap between dataset splits, the performance of our methods is consistently better than the baseline model trained on the entire dataset.
更多查看译文
关键词
Deep Learning, Multiple Data Set, Multiple Criteria Decision Making, Distributed Systems
AI 理解论文
溯源树
样例
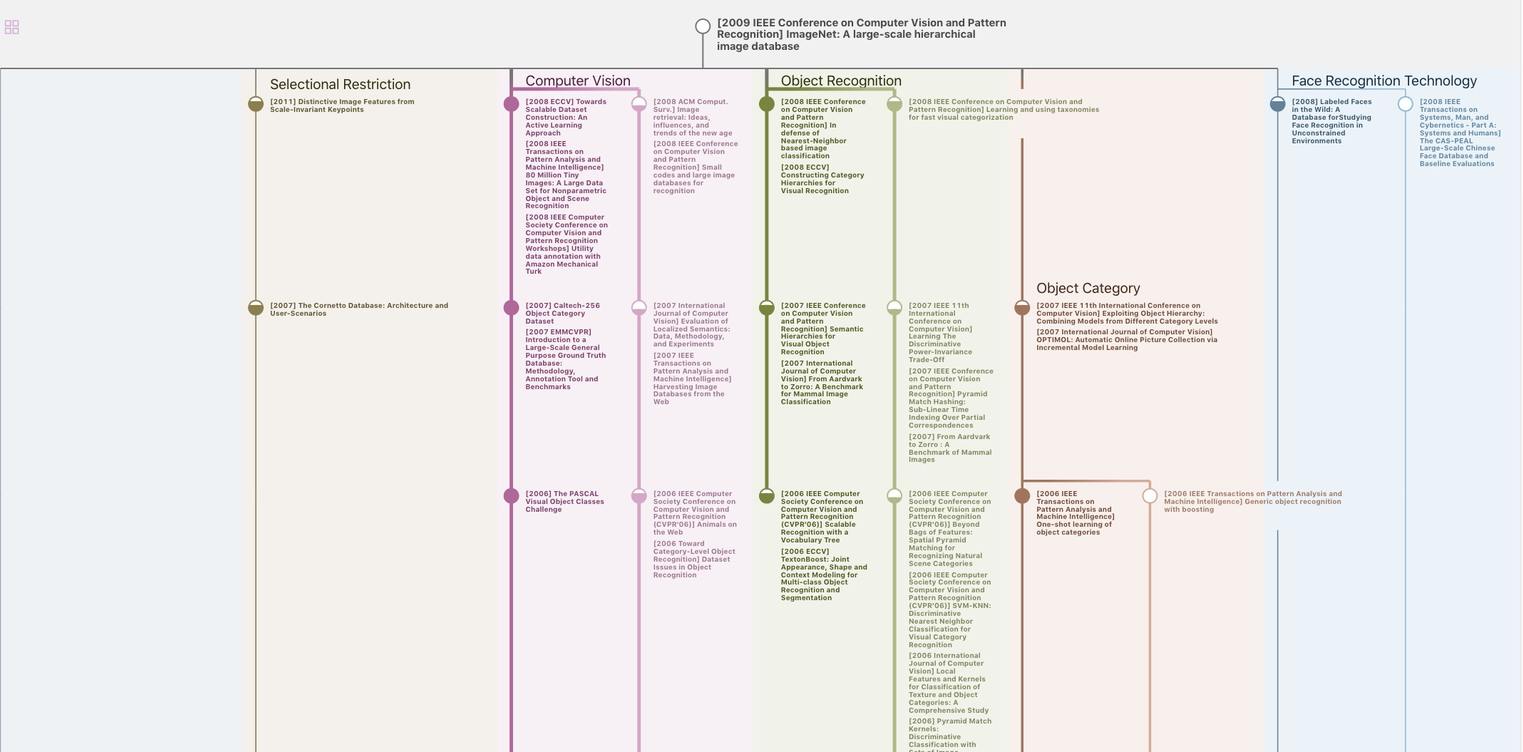
生成溯源树,研究论文发展脉络
Chat Paper
正在生成论文摘要