Effective Heuristic Techniques for Combined Robust Clustering Problem
ASIA-PACIFIC JOURNAL OF OPERATIONAL RESEARCH(2023)
摘要
Clustering is one of the most important problems in the fields of data mining, machine learning, and biological population division, etc. Moreover, robust variant for k-means problem, which includes k-means with penalties and k-means with outliers, is also an active research branch. Most of these problems are NP-hard even the most classical problem, k-means problem. For the NP-hard problems, the heuristic algorithm is a powerful method. When the quality of the output can be guaranteed, the algorithm is called an approximation algorithm. In this paper, combining two types of robust settings, we consider k-means problem with penalties and outliers (k-MPO). In the k-MPO, we are given an n-point set U subset of R-d, a penalty cost pv >= 0 for each v is an element of U, an integer k <= n, and an integer z <= n. The target is to find a center subset S subset of R-d with vertical bar S vertical bar <= k, a penalty subset P subset of U and an outlier subset Z subset of U with vertical bar Z vertical bar <= z, such that the sum of the total costs, including the connection cost and the penalty cost, is minimized. We offer an approximation algorithm using a heuristic local search scheme. Based on a single-swap manipulation, we obtain 274-approximation algorithm.
更多查看译文
关键词
Robust clustering problem, k-means, local search scheme, approximation algorithm
AI 理解论文
溯源树
样例
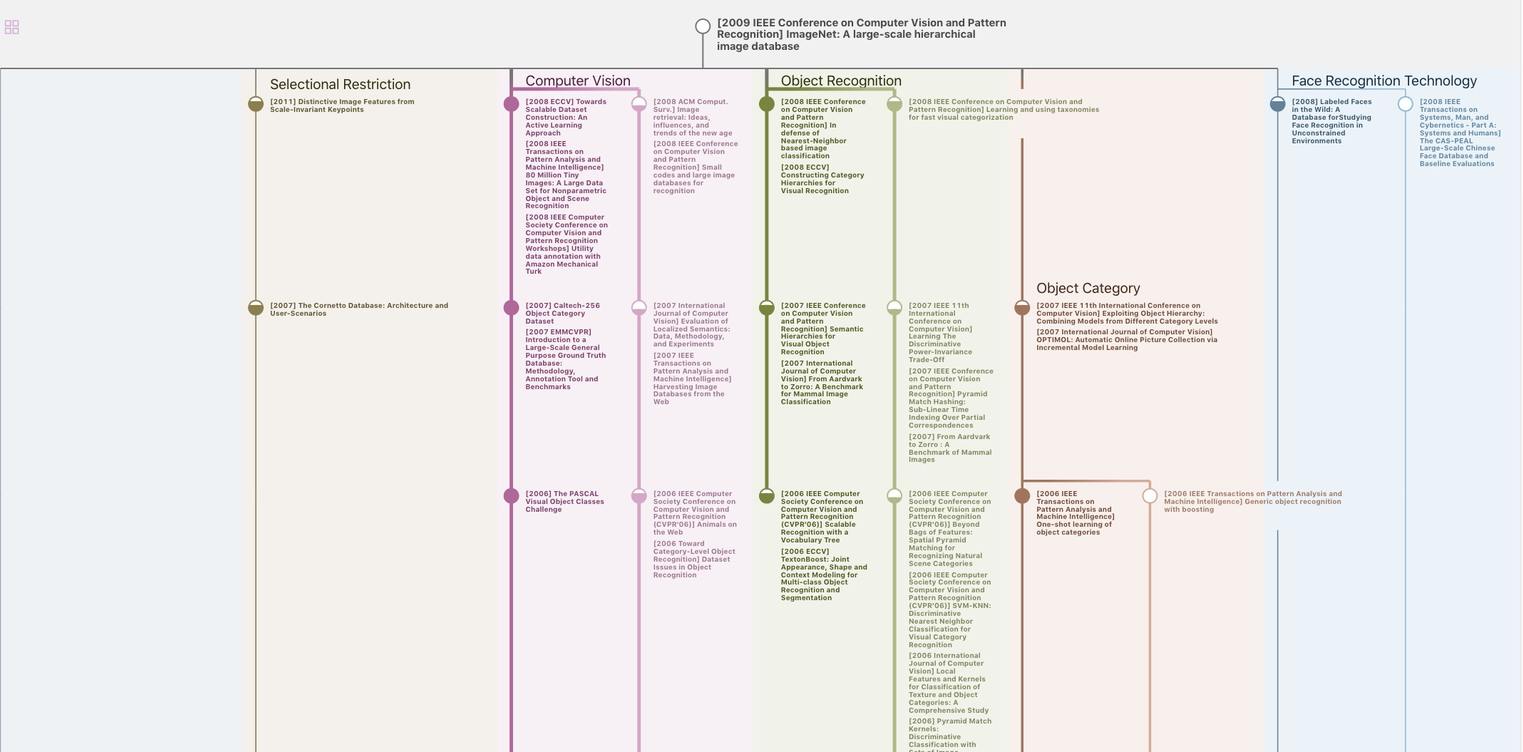
生成溯源树,研究论文发展脉络
Chat Paper
正在生成论文摘要