Stochastic Zeroth-Order Riemannian Derivative Estimation and Optimization
MATHEMATICS OF OPERATIONS RESEARCH(2023)
摘要
We consider stochastic zeroth-order optimization over Riemannian submanifolds embedded in Euclidean space, where the task is to solve Riemannian optimization problems with only noisy objective function evaluations. Toward this, our main contribution is to propose estimators of the Riemannian gradient and Hessian from noisy objective function evaluations, based on a Riemannian version of the Gaussian smoothing technique. The proposed estimators overcome the difficulty of nonlinearity of the manifold constraint and issues that arise in using Euclidean Gaussian smoothing techniques when the function is defined only over the manifold. We use the proposed estimators to solve Riemannian optimization problems in the following settings for the objective function: (i) stochastic and gradient-Lipschitz (in both nonconvex and geodesic convex settings), (ii) sum of gradient Lipschitz and nonsmooth functions, and (iii) Hessian-Lipschitz. For these settings, we analyze the oracle complexity of our algorithms to obtain appropriately defined notions of c-stationary point or c-approximate local minimizer. Notably, our complexities are independent of the dimension of the ambient Euclidean space and depend only on the intrinsic dimension of the manifold under consideration. We demonstrate the applicability of our algorithms by simulation results and real-world applications on black-box stiffness control for robotics and black-box attacks to neural networks.
更多查看译文
关键词
Riemannian optimization, manifold optimization, zeroth-order optimization, black-box attack
AI 理解论文
溯源树
样例
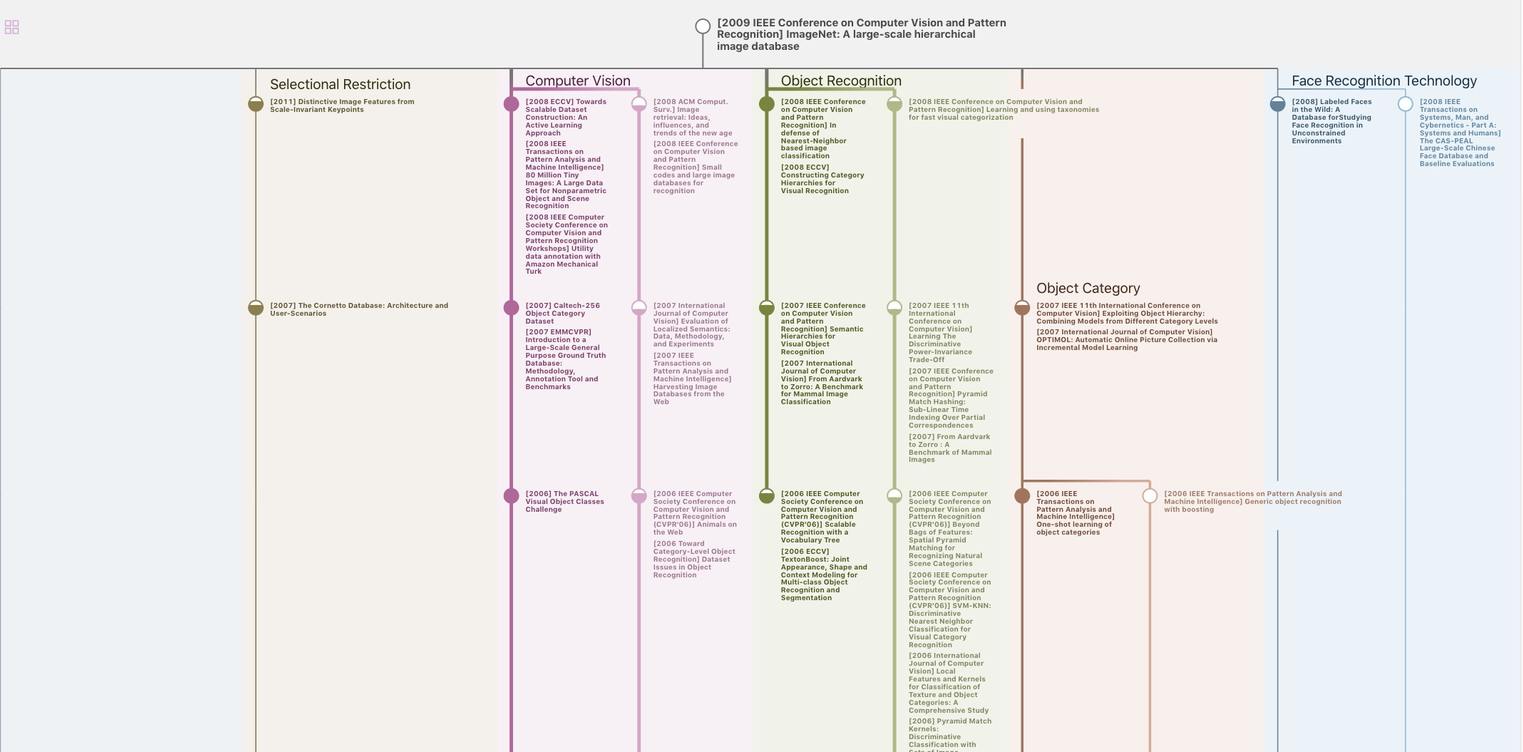
生成溯源树,研究论文发展脉络
Chat Paper
正在生成论文摘要