A Deep Learning Model to Predict Evapotranspiration and Relative Humidity for Moisture Control in Tomato Greenhouses
AGRONOMY-BASEL(2022)
摘要
The greenhouse industry achieves stable agricultural production worldwide. Various information and communication technology techniques to model and control the environment have been applied as data from environmental sensors and actuators in greenhouses are monitored in real time. The current study designed data-based, deep learning models for evapotranspiration (ET) and humidity in tomato greenhouses. Using time-series data and applying long short-term memory (LSTM) modeling, an ET prediction model was developed and validated in comparison with the Stanghellini model. Training with 20-day and testing with 3-day data resulted in RMSEs of 0.00317 and 0.00356 kgm(-2) s(-1), respectively. The standard error of prediction indicated errors of 5.76 and 6.45% in training and testing, respectively. Variables were used to produce a feature map using a two-dimensional convolution layer which was transferred to a subsequent layer and finally connected with the LSTM structure for modeling. The RMSE in humidity prediction using the test dataset was 2.87, indicating a performance better than conventional RNN-LSTM models. Irrigation plans and humidity control may be more accurately conducted in greenhouse cultivation using this model.
更多查看译文
关键词
intelligent modeling for crops and their environment, multi-factor control for greenhouse environment, deep learning in agriculture
AI 理解论文
溯源树
样例
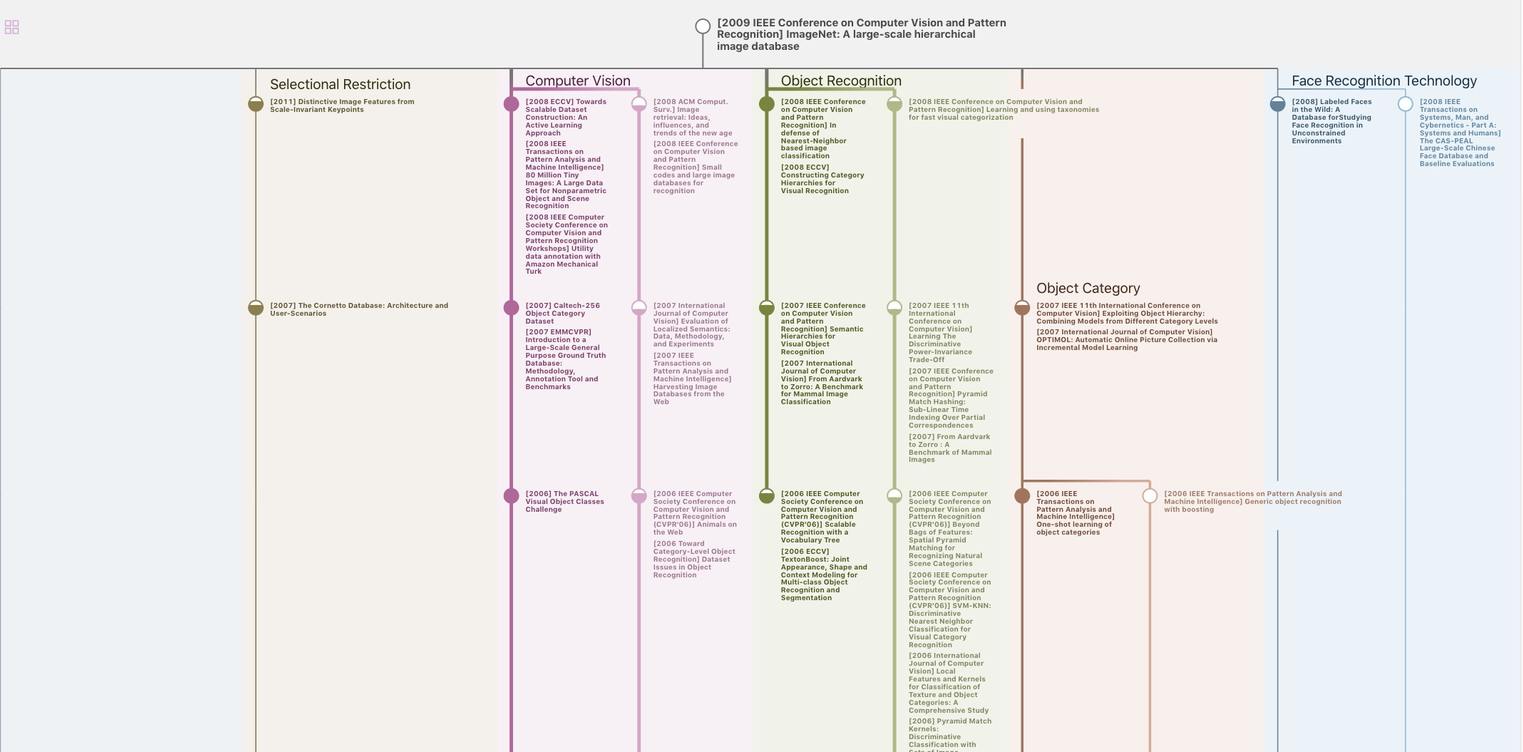
生成溯源树,研究论文发展脉络
Chat Paper
正在生成论文摘要