Fault Classification in a Reciprocating Compressor and a Centrifugal Pump Using Non-Linear Entropy Features
MATHEMATICS(2022)
摘要
This paper describes a comparison of three types of feature sets. The feature sets were intended to classify 13 faults in a centrifugal pump (CP) and 17 valve faults in a reciprocating compressor (RC). The first set comprised 14 non-linear entropy-based features, the second comprised 15 information-based entropy features, and the third comprised 12 statistical features. The classification was performed using random forest (RF) models and support vector machines (SVM). The experimental work showed that the combination of information-based features with non-linear entropy-based features provides a statistically significant accuracy higher than the accuracy provided by the Statistical Features set. Results for classifying the 13 conditions in the CP using non-linear entropy features showed accuracies of up to 99.50%. The same feature set provided a classification accuracy of 97.50% for the classification of the 17 conditions in the RC.
更多查看译文
关键词
approximate entropy, non-linear systems, phase space reconstruction, fault classification, random forest, support vector machines
AI 理解论文
溯源树
样例
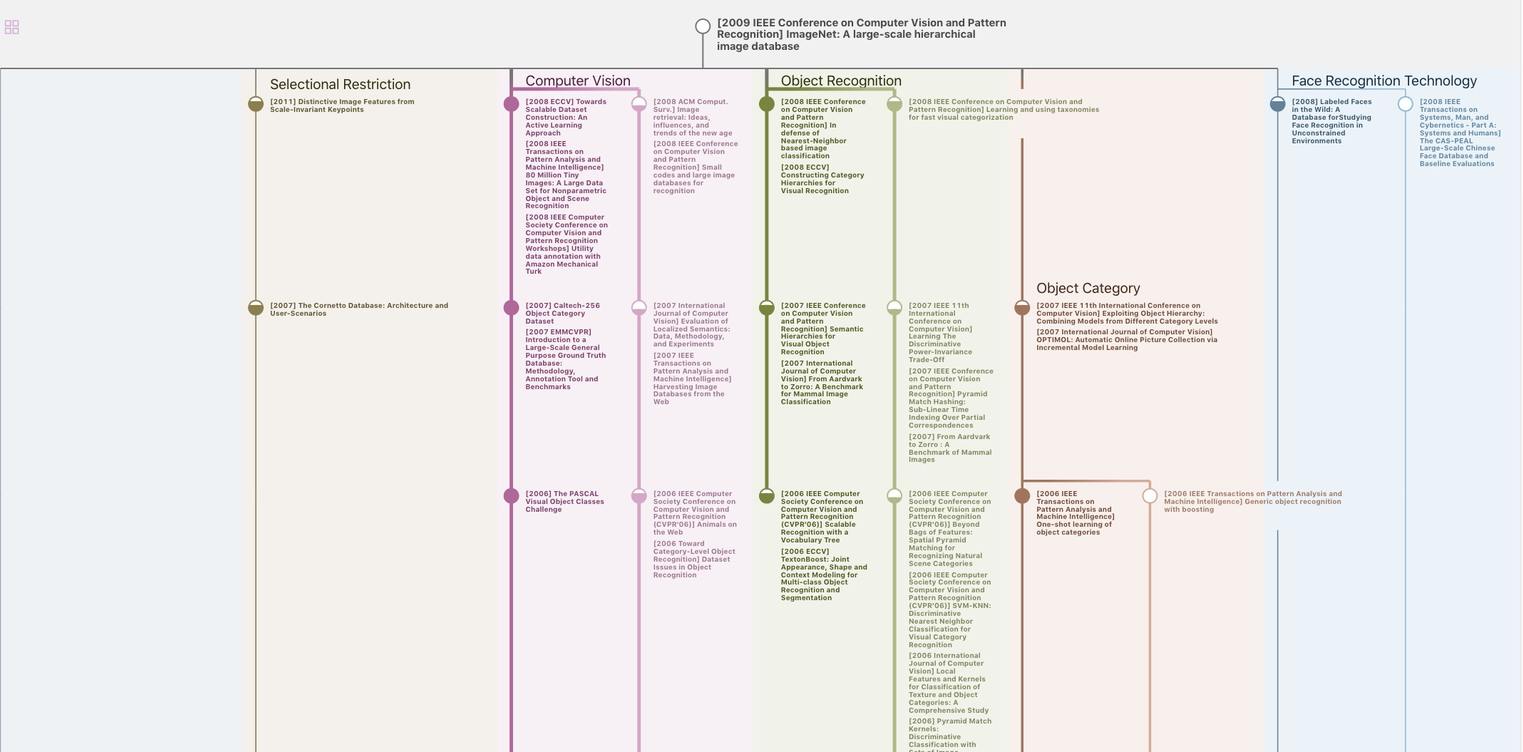
生成溯源树,研究论文发展脉络
Chat Paper
正在生成论文摘要