Metrics for Evaluating Adversarial Attack Patterns
GEOSPATIAL INFORMATICS XII(2022)
摘要
The machine learning community has seen an explosion in the sophistication of adversarial attacks against deep neural network-based computer vision models. In particular, researchers have successfully used adversarial patterns to trigger false positive or false negative results in both research and real-world settings. However, researchers have not yet codified p erformance m etrics f or e valuating t he e fficacy of att ack tec hniques. This evaluation is needed to adequately assess performance improvements of novel adversarial attack methods. This study aims to contribute the following: adversarial pattern performance metrics, demonstration of each metric's strengths and contributions on a case study, and an initial standardized performance evaluation strategy for novel adversarial pattern attacks. We train state-of-the-art deep neural network-based object detection models on an open-source dataset. We then use these trained models to evaluate trained adversarial patterns for both false positive and false negative attacks and evaluate their performance using our suite of metrics in order to establish and codify a workflow to be used when evaluating future adversarial pattern algorithms.
更多查看译文
关键词
adversarial machine learning, ML, attacks, metrics, computer vision, AI, security
AI 理解论文
溯源树
样例
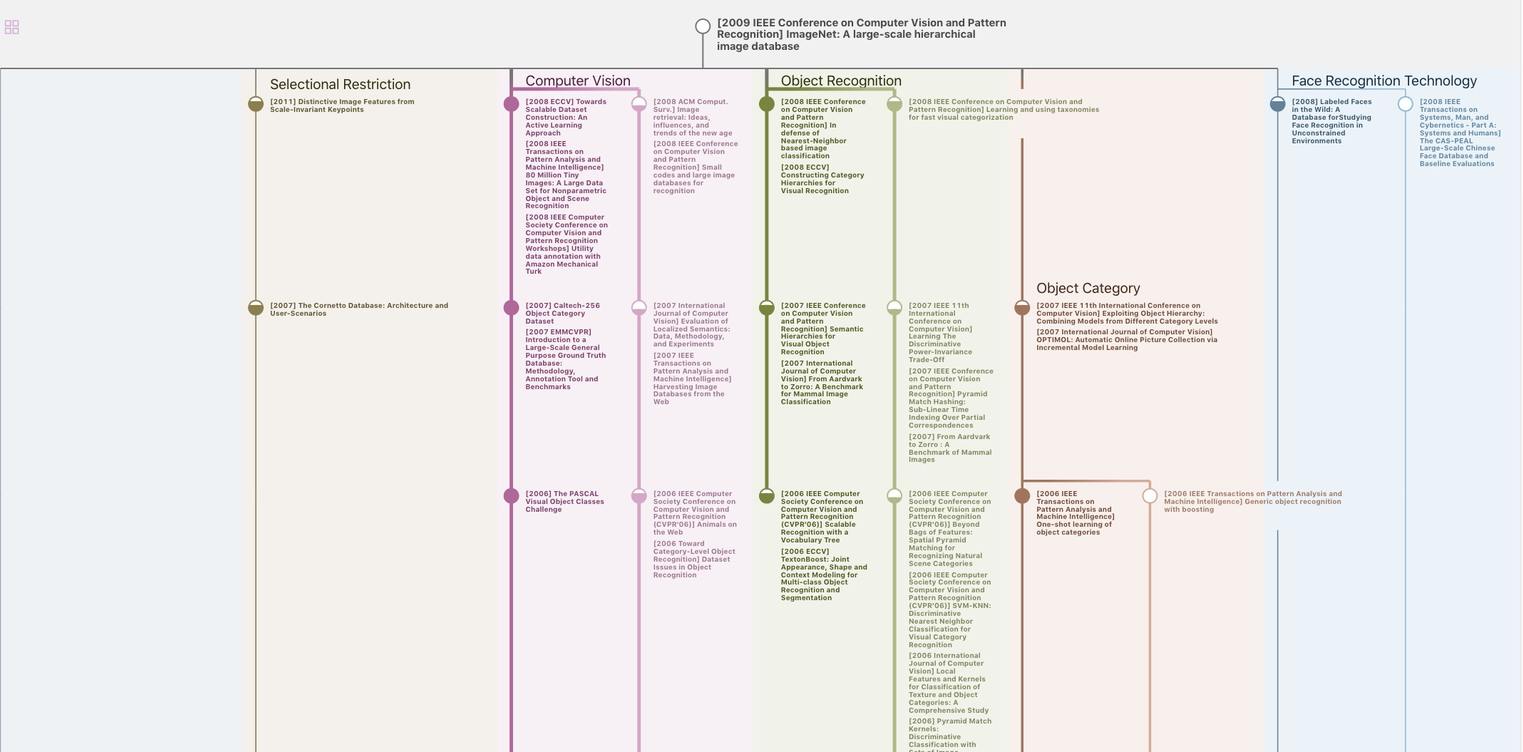
生成溯源树,研究论文发展脉络
Chat Paper
正在生成论文摘要