Hyperspectral Image Completion Via Tensor Factorization with a Bi-regularization Term
Journal of Signal Processing Systems(2022)
摘要
The main purpose of this article is to study a new model of low rank tensor completion. The goal is to predict missing values from a small set of observations. More particular, we present a model of hyperspectral images completion based on a tensor factorization guaranteeing the low rank property and a bi-regularization term promising the preservation of local details in the reconstruction. Such a factorization is used to decompose the tensor as the tensor-matrix mode-3 product of a low-dimensional tensor and a low-dimensional matrix. The bi-regularization term is given by the combination of the piecewise smoothing properties and the coherence constraint between the spectral bands of hyperspectral images. For the minimization process, we consider a Proximal Alternating Minimization (PAM) algorithm to solve the tensor completion model. Several numerical experiments were presented to demonstrate the performance of our proposed method compared to other tensor completion methods in terms of visual and quantitative measurements.
更多查看译文
关键词
Missing values, Tensor factorization, Bi-factor regularization, Hyperspectral images recovery
AI 理解论文
溯源树
样例
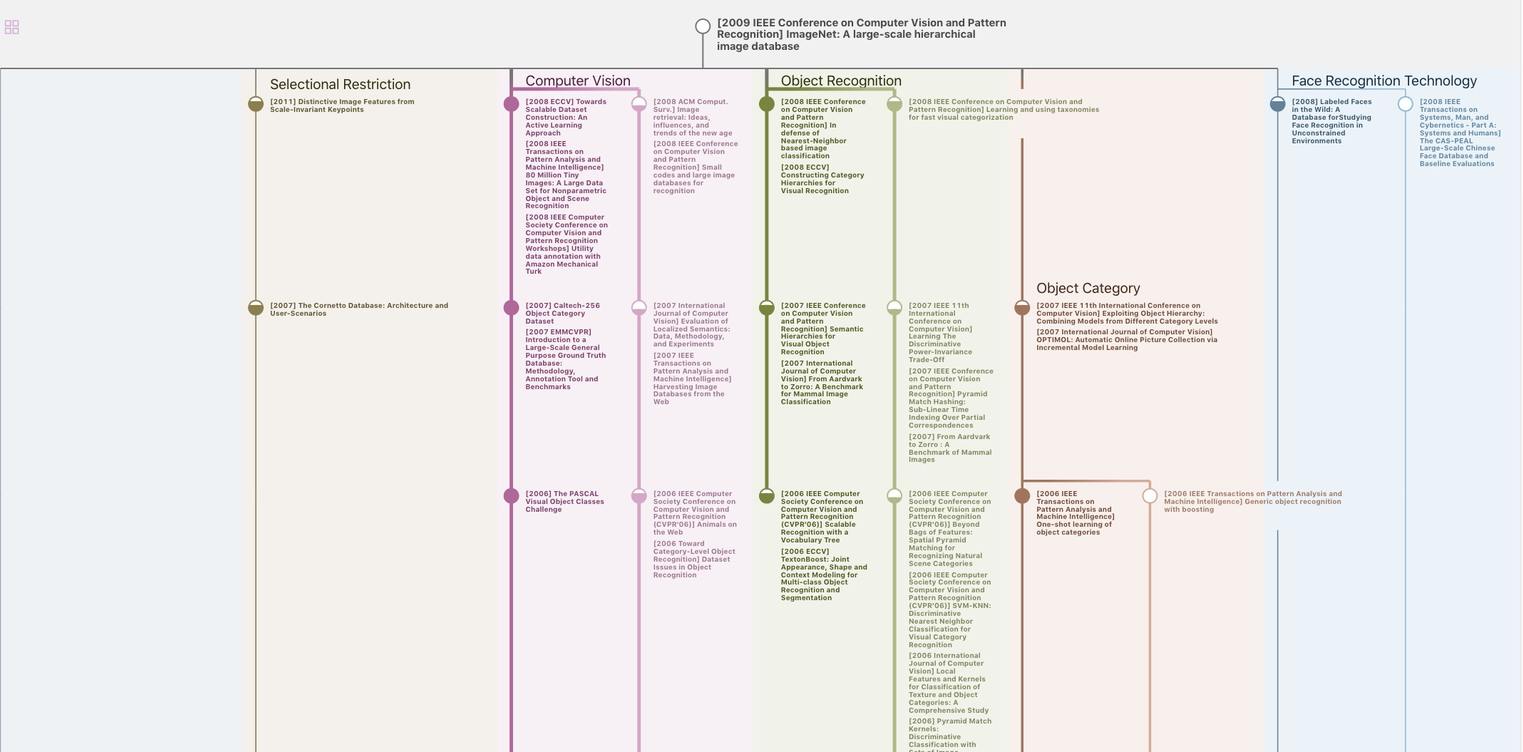
生成溯源树,研究论文发展脉络
Chat Paper
正在生成论文摘要