Class-Level Confidence Based 3D Semi-Supervised Learning
2023 IEEE/CVF Winter Conference on Applications of Computer Vision (WACV)(2023)
摘要
Recent state-of-the-art method FlexMatch firstly demonstrated that correctly estimating learning status is crucial for semi-supervised learning (SSL). However, the estimation method proposed by FlexMatch does not take into account imbalanced data, which is the common case for 3D semi-supervised learning. To address this problem, we practically demonstrate that unlabeled data class-level confidence can represent the learning status in the 3D imbalanced dataset. Based on this finding, we present a novel class-level confidence based 3D SSL method. Firstly, a dynamic thresholding strategy is proposed to utilize more unlabeled data, especially for low learning status classes. Then, a re-sampling strategy is designed to avoid biasing toward high learning status classes, which dynamically changes the sampling probability of each class. To show the effectiveness of our method in 3D SSL tasks, we conduct extensive experiments on 3D SSL classification and detection tasks. Our method significantly outperforms state-of-the-art counterparts for both 3D SSL classification and detection tasks in all datasets.
更多查看译文
关键词
Algorithms: Machine learning architectures,formulations,and algorithms (including transfer),3D computer vision
AI 理解论文
溯源树
样例
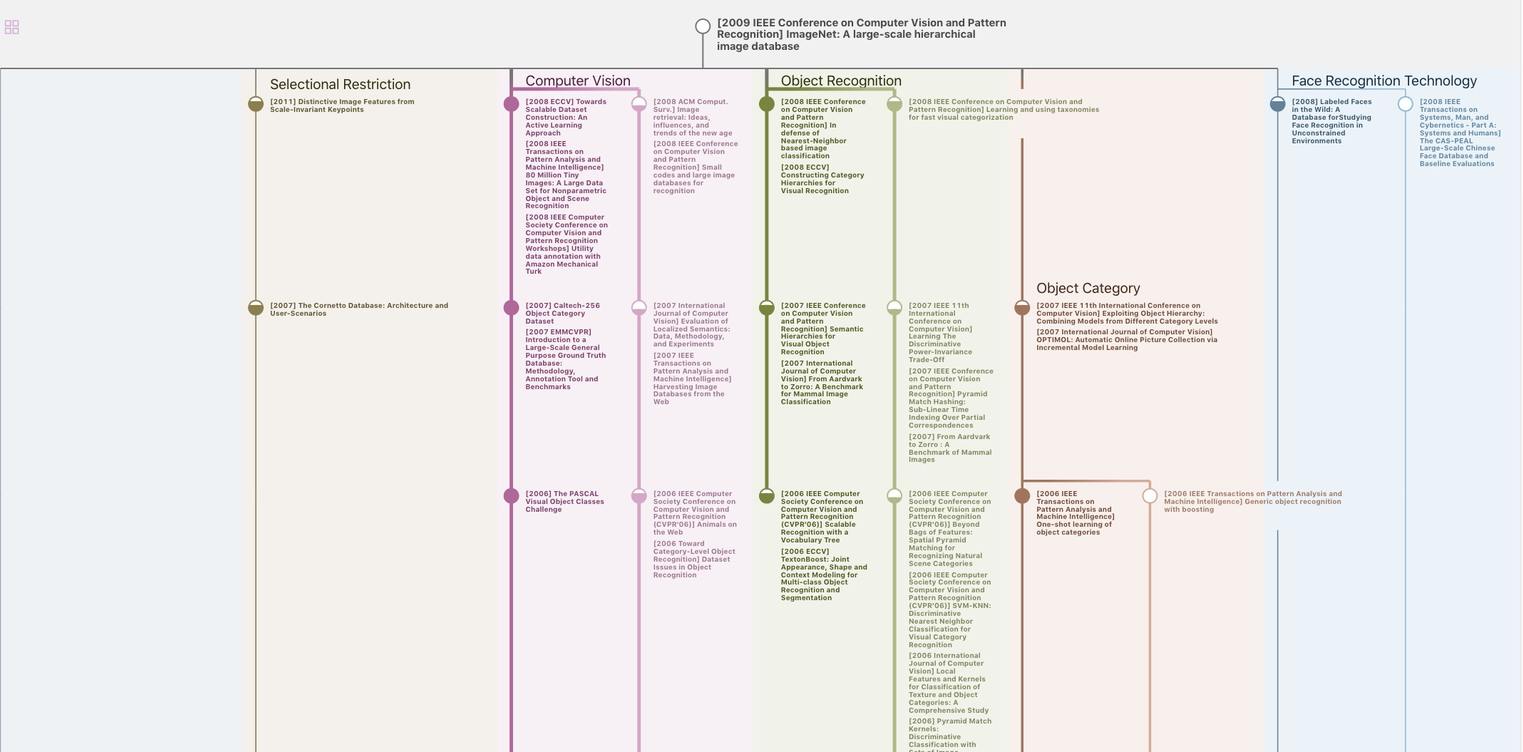
生成溯源树,研究论文发展脉络
Chat Paper
正在生成论文摘要