Anomaly detection for process monitoring based on machine learning technique
Neural Comput. Appl.(2022)
摘要
Anomaly detection is critical to process modeling, monitoring, and control since successful execution of these engineering tasks depends on access to validated data. The industrial process is uncertain in several situations, and the available information is formalized in terms of intervals. This article deals with the diagnostic of uncertain systems by multivariate static analysis. Linear Principal Component Analysis (PCA) and nonlinear Kernel PCA (KPCA) are generally used to deal with certain systems; they exploit single-valued variables. While in real situations these data are marred by uncertainties, these uncertainties cause difficulties in making decision in relation to the presence of defects. Thus, we have studied a recent and robust solution which consists in capturing the variability of multivariate observations by interval variables. In the first part, we treated a fault detection strategy based on interval PCA in the case of static linear systems. It includes first of all a comparative study between the deferent methods of detection of faults with interval PCA in which we proposed a new detection statistics of faults. In the second part, we studied a fault detection strategy based on interval KPCA method; we propose a reduction approach to solve the problem of nonlinearity and uncertainty and the problem of large data. The proposed fault detection methods are illustrated by synthetic data with an in-depth study and comparison using simulations of the air quality monitoring network and the Tennessee Eastman process.
更多查看译文
关键词
Fault detection,Anomaly detection,Uncertain process,RRIKPCA,Interval fault detection index
AI 理解论文
溯源树
样例
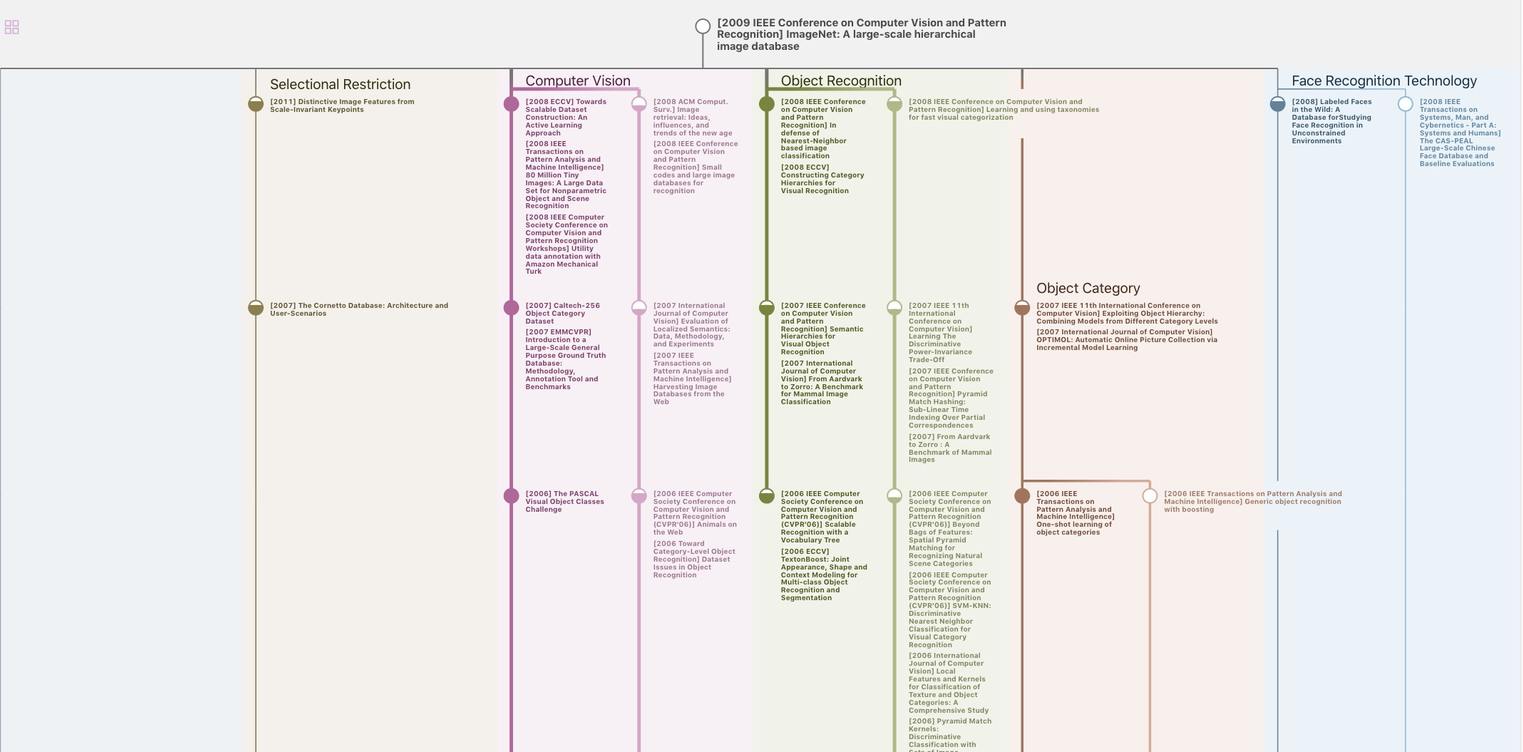
生成溯源树,研究论文发展脉络
Chat Paper
正在生成论文摘要